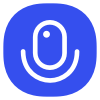
Sign up to save your podcasts
Or
In this episode, we take a deep dive into one of the most exciting breakthroughs in modern robotics — the new .5 model (Point Five), based on the Vision-Language-Action paradigm. It was developed to tackle one of the most persistent challenges in robotics: teaching robots to act effectively in uncontrolled, unpredictable home environments, far beyond the repetitive tasks of factory floors.
The .5 model introduces a radically different approach: co-training on heterogeneous tasks and transfer learning across diverse data types, including:
recordings and behaviors from a wide range of robots — from stationary lab arms to mobile home assistants;
natural language instructions from humans;
multimodal web data — images, captions, visual question answering, and object detection datasets;
hierarchical task planning: breaking down vague commands like "clean the room" into specific steps such as "place books on the shelf."
Despite only 2.4% of training data coming from mobile robots performing real household tasks, .5 demonstrated the ability to generalize to new, unseen homes. It succeeded in carrying out multi-step tasks like tidying up, moving laundry, and placing dishes — all without prior exposure to these environments.
This is possible thanks to:
semantic subtask prediction, helping the model plan intermediate steps;
cross-embodiment learning, where robots learn from others with completely different designs;
flow matching, a technique for generating smooth, continuous real-world motion;
and a tokenized + continuous action representation, combining discrete learning efficiency with smooth robotic control.
Even more fascinating is that .5 can learn how to interact with objects it has never seen in real life — simply by analyzing images and descriptions online. This builds a kind of common sense in AI, essential for navigating the real world.
We’ll also cover:
how the .5 architecture enables hierarchical thinking and decision-making;
how greater diversity in training environments directly improved generalization;
which data types were most critical based on ablation experiments;
and what’s next for truly versatile, general-purpose robots.
Read more: https://www.pi.website/blog/pi05
In this episode, we take a deep dive into one of the most exciting breakthroughs in modern robotics — the new .5 model (Point Five), based on the Vision-Language-Action paradigm. It was developed to tackle one of the most persistent challenges in robotics: teaching robots to act effectively in uncontrolled, unpredictable home environments, far beyond the repetitive tasks of factory floors.
The .5 model introduces a radically different approach: co-training on heterogeneous tasks and transfer learning across diverse data types, including:
recordings and behaviors from a wide range of robots — from stationary lab arms to mobile home assistants;
natural language instructions from humans;
multimodal web data — images, captions, visual question answering, and object detection datasets;
hierarchical task planning: breaking down vague commands like "clean the room" into specific steps such as "place books on the shelf."
Despite only 2.4% of training data coming from mobile robots performing real household tasks, .5 demonstrated the ability to generalize to new, unseen homes. It succeeded in carrying out multi-step tasks like tidying up, moving laundry, and placing dishes — all without prior exposure to these environments.
This is possible thanks to:
semantic subtask prediction, helping the model plan intermediate steps;
cross-embodiment learning, where robots learn from others with completely different designs;
flow matching, a technique for generating smooth, continuous real-world motion;
and a tokenized + continuous action representation, combining discrete learning efficiency with smooth robotic control.
Even more fascinating is that .5 can learn how to interact with objects it has never seen in real life — simply by analyzing images and descriptions online. This builds a kind of common sense in AI, essential for navigating the real world.
We’ll also cover:
how the .5 architecture enables hierarchical thinking and decision-making;
how greater diversity in training environments directly improved generalization;
which data types were most critical based on ablation experiments;
and what’s next for truly versatile, general-purpose robots.
Read more: https://www.pi.website/blog/pi05