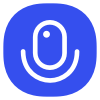
Sign up to save your podcasts
Or
In this episode of In-Ear Insights, the Trust Insights podcast, Katie and Chris discuss Retrieval Augmented Generation (RAG). You’ll learn what RAG is and how it can significantly improve the accuracy and relevance of AI responses by using your own data. You’ll understand the crucial differences between RAG and typical search engines or generative AI models, clarifying when RAG is truly needed. You’ll discover practical examples of when RAG becomes essential, especially for handling sensitive company information and proprietary knowledge. Tune in to learn when and how RAG can be a game-changer for your data strategy and when simpler AI tools will suffice!
Watch the video here:
Can’t see anything? Watch it on YouTube here.
Listen to the audio here:
Download the MP3 audio here.
[podcastsponsor]
What follows is an AI-generated transcript. The transcript may contain errors and is not a substitute for listening to the episode.
Christopher S. Penn – 00:00
Christopher S. Penn – 00:02
Christopher S. Penn – 00:06
Christopher S. Penn – 00:07
Christopher S. Penn – 00:09
Katie Robbert – 00:17
Katie Robbert – 00:41
Katie Robbert – 00:44
Katie Robbert – 01:05
Katie Robbert – 01:07
Christopher S. Penn – 01:09
Probably one of the most well-known and easiest-to-use systems like this is Google’s free NotebookLM where you just put in a bunch of documents. It does all the work—the technical stuff of tokenization and embeddings and all that stuff. And then you can chat with your documents and say, ‘Well, what’s in this?’
In our examples, we’ve used the letters from the corner office books that we’ve written every year, and those are all of your cold opens from the newsletter.
Christopher S. Penn – 01:58
Christopher S. Penn – 02:07
Christopher S. Penn – 02:10
There is a querying system that your generative AI tool knows to ask the database, and then you can constrain the system. So you can say, ‘I only want you to use this database,’ or you can use this database plus your other knowledge that you’ve already been trained on.
Christopher S. Penn – 02:34
Christopher S. Penn – 02:48
Christopher S. Penn – 02:53
Katie Robbert – 02:56
Katie Robbert – 03:15
Katie Robbert – 03:17
Katie Robbert – 03:26
Katie Robbert – 03:27
Katie Robbert – 03:43
Katie Robbert – 03:45
Katie Robbert – 03:47
Katie Robbert – 03:48
Katie Robbert – 04:04
Katie Robbert – 04:10
Christopher S. Penn – 04:14
Christopher S. Penn – 04:27
Christopher S. Penn – 04:42
Christopher S. Penn – 04:43
And then be able to say, ‘Okay, Katie’s asking about accounting receipts from 2023.’ And it’s vectorized and converted all the knowledge into the specific language, the specific format that generative AI requires.
And then when you write the prompt…
Christopher S. Penn – 05:21
Christopher S. Penn – 05:23
Christopher S. Penn – 05:33
Christopher S. Penn – 05:36
Katie Robbert – 05:43
Christopher S. Penn – 05:44
Katie Robbert – 05:45
Katie Robbert – 06:01
Katie Robbert – 06:02
Katie Robbert – 06:14
Katie Robbert – 06:16
Christopher S. Penn – 06:33
Christopher S. Penn – 06:36
Christopher S. Penn – 06:38
Christopher S. Penn – 06:40
Christopher S. Penn – 06:47
Christopher S. Penn – 06:48
I might say for any prompts that we pass this thing, ‘You can only use Katie’s newsletters.’ Or I might say, ‘You should use Katie’s newsletters first.’
So if I say, ‘Write a blog post about project management,’ it would refer…
Christopher S. Penn – 07:25
Christopher S. Penn – 07:29
Christopher S. Penn – 07:47
Christopher S. Penn – 07:50
Katie Robbert – 08:04
Katie Robbert – 08:08
Katie Robbert – 08:22
Katie Robbert – 08:24
Christopher S. Penn – 08:26
When you create something like a GPT where you upload documents, there’s a limit.
Christopher S. Penn – 08:34
Christopher S. Penn – 08:36
Christopher S. Penn – 08:51
The table collapsed because it was so heavy, and it was hundreds of thousands of pages. You can’t put that in knowledge—in knowledge files. There’s just too much of it.
But what you can do is you could download it, put it into this one of these retrieval augmented generation databases.
Christopher S. Penn – 09:15
Christopher S. Penn – 09:17
Christopher S. Penn – 09:20
Katie Robbert – 09:41
Katie Robbert – 09:49
Katie Robbert – 09:57
It’s just going to look for everything because you’re looking for everything because you don’t know specifically. Otherwise, you would have said, ‘Bring me to the U.S. Tax database…’
Katie Robbert – 10:17
Katie Robbert – 10:23
Katie Robbert – 10:36
Katie Robbert – 10:44
Katie Robbert – 10:49
Katie Robbert – 10:53
Katie Robbert – 11:01
Christopher S. Penn – 11:04
Katie Robbert – 11:05
So if I’m your everyday marketer, which I am, I’m not overly technical. I understand technical theories and I understand technical practices.
But if I’m not necessarily a power user of generative AI like you are, Chris, what are some—why do I need to understand what retrieval augmented generation is? How would I use this thing?
Christopher S. Penn – 11:32
Christopher S. Penn – 11:35
Christopher S. Penn – 11:37
You are a healthcare system. You have patient data. You cannot load that to NotebookLM, but you absolutely could create a RAG system internally and then allow—within your own secured network—doctors to query all of the medical records to say, ‘Have we seen a case like this before? Hey, this person came in with these symptoms.’
Christopher S. Penn – 12:03
Christopher S. Penn – 12:04
Christopher S. Penn – 12:07
Christopher S. Penn – 12:08
For the average marketing, I’m writing a social media post. You’re not going to use RAG because there’s no point in doing that.
If you had confidential information or proprietary information that you did not feel comfortable loading into a NotebookLM, then a RAG system would make sense.
So if you were to say maybe you have a new piece of software that your company is going to be rolling out and the developers actually did their job and wrote documentation and you didn’t want Google to be aware of it—wow, I know we’re in science fiction land here—you might load that to a RAG system, say, ‘Now let me help me…
Christopher S. Penn – 12:48
Christopher S. Penn – 12:50
Or I’m an agency and I’m working with client data and our contract says we may not use third parties.
Regardless of the reason, no matter how safe you think it is, your contract says you cannot use third party. So you would build a RAG system internally for that client data and then query it because your contract says you can’t use NotebookLM.
Katie Robbert – 13:22
Katie Robbert – 13:26
Katie Robbert – 13:28
Christopher S. Penn – 13:49
Christopher S. Penn – 13:51
Christopher S. Penn – 13:53
I mean, that’s really fundamentally what Retrieval Augmented Generation is about. It’s us saying, ‘Hey, AI model, you don’t understand this topic well.’
Like, if you were writing content about SEO and you notice that AI is spitting out SEO tips from 2012, you’re like, ‘Okay, clearly you don’t know SEO as well as we do.’
You might use a RAG system to say, ‘This is what we know to be true about SEO in 2025.’
Christopher S. Penn – 14:34
Christopher S. Penn – 14:36
Katie Robbert – 14:41
Katie Robbert – 14:48
Christopher S. Penn – 14:53
Katie Robbert – 14:54
Katie Robbert – 15:05
Katie Robbert – 15:09
We’re just now understanding the proper terminology.
Katie Robbert – 15:16
Katie Robbert – 15:18
Katie Robbert – 15:28
Katie Robbert – 15:34
And that’s what I’m trying to understand is it sounds like for marketers, for B2B marketers, B2C marketers, even operations, even project managers, sales teams, the everyday, you probably don’t need a RAG system.
Katie Robbert – 15:59
Katie Robbert – 16:00
Katie Robbert – 16:12
Katie Robbert – 16:14
Katie Robbert – 16:16
Katie Robbert – 16:23
Katie Robbert – 16:26
Katie Robbert – 16:30
Katie Robbert – 16:41
Katie Robbert – 16:43
Katie Robbert – 16:46
Katie Robbert – 16:51
Christopher S. Penn – 16:55
Christopher S. Penn – 16:57
We write up, ‘Here’s what Trust Insights is, here’s who it does.’
Think of a RAG system as a system that can generate a relevant knowledge block dynamically on the fly.
Christopher S. Penn – 17:10
And we record those; we have the transcripts of those. That’s a lot. That’s basically an hour-plus of audio every week. It’s 6,000 words.
And on those calls, we discuss everything from our dogs to sales things. I would never want to try to include all 500 transcripts of the company into an AI prompt.
Christopher S. Penn – 17:40
Christopher S. Penn – 17:41
Christopher S. Penn – 17:44
I would create a database, a RAG system that would create all the relevant embeddings and things and put that there.
And then when I say, ‘What neat…
Christopher S. Penn – 17:57
Christopher S. Penn – 17:58
Christopher S. Penn – 18:02
Christopher S. Penn – 18:05
Christopher S. Penn – 18:08
Christopher S. Penn – 18:10
Christopher S. Penn – 18:16
Christopher S. Penn – 18:18
Christopher S. Penn – 18:20
Christopher S. Penn – 18:22
So that’s a really good example of where that RAG system would come into play.
If you have, for example…
Christopher S. Penn – 18:43
Christopher S. Penn – 18:46
Christopher S. Penn – 18:52
Christopher S. Penn – 18:53
And it’d be able to spit that out. And then you could have a conversation with just that knowledge block that it generated by itself.
Katie Robbert – 19:09
Katie Robbert – 19:11
Katie Robbert – 19:13
And I’m like, ‘Okay, yeah, so where’s that thing? I need that.’
But what you’re doing is you’re giving that real-world demonstration of when a retrieval augmented generation system is actually applicable.
Katie Robbert – 19:34
Katie Robbert – 19:37
Katie Robbert – 19:41
Katie Robbert – 19:42
Katie Robbert – 19:45
Katie Robbert – 19:47
Christopher S. Penn – 19:50
Christopher S. Penn – 20:07
Christopher S. Penn – 20:10
So for example, maybe you want to do a wrap-up of SEO best practices in 2025. So you go to Google deep research and OpenAI deep research and Perplexity Deep Research and you get some reports and you merge them together.
You don’t need a RAG system for that. These other tools have stepped in.
Christopher S. Penn – 20:32
Christopher S. Penn – 20:34
Yeah, you don’t need a RAG system for that because you’re providing the knowledge block.
Christopher S. Penn – 20:51
Christopher S. Penn – 20:52
Katie Robbert – 21:08
Katie Robbert – 21:12
Christopher S. Penn – 21:16
One of the biggest use cases for that is encoding, where you have a really big system, you load all of your code into your own internal RAG, and then you can have your coding agents reference your own code, figure out what code is in your code base, and then make changes to it that way. That’s a good use of that type of system.
But for the average marketer, that is ridiculous. There’s no reason to that. That’s like taking your fighter jet to the grocery store.
It’s vast overkill. When a bicycle would have done just fine.
Katie Robbert – 22:00
Katie Robbert – 22:11
Christopher S. Penn – 22:15
Christopher S. Penn – 22:30
Christopher S. Penn – 22:36
Katie Robbert – 22:38
All right, so it sounds like for everyday use, you don’t necessarily need to…
Katie Robbert – 22:54
Katie Robbert – 23:01
Katie Robbert – 23:08
Katie Robbert – 23:10
If you have proprietary data like personally identifying information, patient information, customer information—that’s where you would probably want to build…
Katie Robbert – 23:27
Katie Robbert – 23:30
Katie Robbert – 23:32
Christopher S. Penn – 23:35
Christopher S. Penn – 23:36
Christopher S. Penn – 23:37
Christopher S. Penn – 23:42
Christopher S. Penn – 23:43
And then we can talk about setting up like a Pinecone or Weaviate or a Milvus for an organization. Because there are RAG systems you can run locally on your computer that are unique to you and those are actually a really good idea, and you can talk about that on the livestream.
But then there’s the institutional version, which has much higher overhead for administration. But as we talked about in the use cases in this episode, there may be really good reasons to do that.
Katie Robbert – 24:22
Katie Robbert – 24:24
Katie Robbert – 24:27
Katie Robbert – 24:34
Katie Robbert – 24:40
Katie Robbert – 24:46
Katie Robbert – 24:47
Katie Robbert – 24:51
Christopher S. Penn – 24:52
Christopher S. Penn – 25:02
Christopher S. Penn – 25:03
All right, so if you’ve got some things you want to share about your experiences with RAG or you have questions about retrieval augmented generation, pop on by our free Slack group. Go to TrustInsights.ai/analyticsformarketers, where you and over 4,000 other marketers are asking and answering each other’s questions every single day about analytics, data science, machine learning, and AI.
And wherever it is you watch or listen to the show, if there’s a…
Christopher S. Penn – 25:29
Christopher S. Penn – 25:31
Christopher S. Penn – 25:46
Christopher S. Penn – 25:50
Christopher S. Penn – 25:52
Trust Insights services span the gamut from developing comprehensive data strategies and conducting deep-dive marketing analysis to building predictive models using tools like TensorFlow and PyTorch, and optimizing content strategies. Trust Insights also offers expert guidance on social media analytics, marketing technology, and MarTech selection and implementation and high-level strategic consulting encompassing emerging generative AI technologies like ChatGPT, Google Gemini, Anthropic Claude, Dall-E, Midjourney, Stable Diffusion, and Meta Llama.
Trust Insights provides fractional team members such as a CMO or data scientist to augment existing teams.
Christopher S. Penn – 26:55
Trust Insights are adept at leveraging cutting-edge generative AI techniques like large language models and diffusion models, yet they excel explaining complex concepts clearly through compelling narratives and visualizations—Data Storytelling. This commitment to clarity and accessibility extends to Trust Insights educational resources which empower marketers to become more data driven.
Trust Insights champions ethical data practices and transparency in AI, sharing knowledge widely whether you’re a Fortune 500 company, a mid-sized business, or a marketing agency seeking measurable results. Trust Insights offers a unique blend of technical expertise, strategic guidance, and educational resources to help you navigate the ever-evolving landscape of modern marketing and business in the age of generative AI.
Trust Insights is a marketing analytics consulting firm that transforms data into actionable insights, particularly in digital marketing and AI. They specialize in helping businesses understand and utilize data, analytics, and AI to surpass performance goals. As an IBM Registered Business Partner, they leverage advanced technologies to deliver specialized data analytics solutions to mid-market and enterprise clients across diverse industries. Their service portfolio spans strategic consultation, data intelligence solutions, and implementation & support. Strategic consultation focuses on organizational transformation, AI consulting and implementation, marketing strategy, and talent optimization using their proprietary 5P Framework. Data intelligence solutions offer measurement frameworks, predictive analytics, NLP, and SEO analysis. Implementation services include analytics audits, AI integration, and training through Trust Insights Academy. Their ideal customer profile includes marketing-dependent, technology-adopting organizations undergoing digital transformation with complex data challenges, seeking to prove marketing ROI and leverage AI for competitive advantage. Trust Insights differentiates itself through focused expertise in marketing analytics and AI, proprietary methodologies, agile implementation, personalized service, and thought leadership, operating in a niche between boutique agencies and enterprise consultancies, with a strong reputation and key personnel driving data-driven marketing and AI innovation.
5
99 ratings
In this episode of In-Ear Insights, the Trust Insights podcast, Katie and Chris discuss Retrieval Augmented Generation (RAG). You’ll learn what RAG is and how it can significantly improve the accuracy and relevance of AI responses by using your own data. You’ll understand the crucial differences between RAG and typical search engines or generative AI models, clarifying when RAG is truly needed. You’ll discover practical examples of when RAG becomes essential, especially for handling sensitive company information and proprietary knowledge. Tune in to learn when and how RAG can be a game-changer for your data strategy and when simpler AI tools will suffice!
Watch the video here:
Can’t see anything? Watch it on YouTube here.
Listen to the audio here:
Download the MP3 audio here.
[podcastsponsor]
What follows is an AI-generated transcript. The transcript may contain errors and is not a substitute for listening to the episode.
Christopher S. Penn – 00:00
Christopher S. Penn – 00:02
Christopher S. Penn – 00:06
Christopher S. Penn – 00:07
Christopher S. Penn – 00:09
Katie Robbert – 00:17
Katie Robbert – 00:41
Katie Robbert – 00:44
Katie Robbert – 01:05
Katie Robbert – 01:07
Christopher S. Penn – 01:09
Probably one of the most well-known and easiest-to-use systems like this is Google’s free NotebookLM where you just put in a bunch of documents. It does all the work—the technical stuff of tokenization and embeddings and all that stuff. And then you can chat with your documents and say, ‘Well, what’s in this?’
In our examples, we’ve used the letters from the corner office books that we’ve written every year, and those are all of your cold opens from the newsletter.
Christopher S. Penn – 01:58
Christopher S. Penn – 02:07
Christopher S. Penn – 02:10
There is a querying system that your generative AI tool knows to ask the database, and then you can constrain the system. So you can say, ‘I only want you to use this database,’ or you can use this database plus your other knowledge that you’ve already been trained on.
Christopher S. Penn – 02:34
Christopher S. Penn – 02:48
Christopher S. Penn – 02:53
Katie Robbert – 02:56
Katie Robbert – 03:15
Katie Robbert – 03:17
Katie Robbert – 03:26
Katie Robbert – 03:27
Katie Robbert – 03:43
Katie Robbert – 03:45
Katie Robbert – 03:47
Katie Robbert – 03:48
Katie Robbert – 04:04
Katie Robbert – 04:10
Christopher S. Penn – 04:14
Christopher S. Penn – 04:27
Christopher S. Penn – 04:42
Christopher S. Penn – 04:43
And then be able to say, ‘Okay, Katie’s asking about accounting receipts from 2023.’ And it’s vectorized and converted all the knowledge into the specific language, the specific format that generative AI requires.
And then when you write the prompt…
Christopher S. Penn – 05:21
Christopher S. Penn – 05:23
Christopher S. Penn – 05:33
Christopher S. Penn – 05:36
Katie Robbert – 05:43
Christopher S. Penn – 05:44
Katie Robbert – 05:45
Katie Robbert – 06:01
Katie Robbert – 06:02
Katie Robbert – 06:14
Katie Robbert – 06:16
Christopher S. Penn – 06:33
Christopher S. Penn – 06:36
Christopher S. Penn – 06:38
Christopher S. Penn – 06:40
Christopher S. Penn – 06:47
Christopher S. Penn – 06:48
I might say for any prompts that we pass this thing, ‘You can only use Katie’s newsletters.’ Or I might say, ‘You should use Katie’s newsletters first.’
So if I say, ‘Write a blog post about project management,’ it would refer…
Christopher S. Penn – 07:25
Christopher S. Penn – 07:29
Christopher S. Penn – 07:47
Christopher S. Penn – 07:50
Katie Robbert – 08:04
Katie Robbert – 08:08
Katie Robbert – 08:22
Katie Robbert – 08:24
Christopher S. Penn – 08:26
When you create something like a GPT where you upload documents, there’s a limit.
Christopher S. Penn – 08:34
Christopher S. Penn – 08:36
Christopher S. Penn – 08:51
The table collapsed because it was so heavy, and it was hundreds of thousands of pages. You can’t put that in knowledge—in knowledge files. There’s just too much of it.
But what you can do is you could download it, put it into this one of these retrieval augmented generation databases.
Christopher S. Penn – 09:15
Christopher S. Penn – 09:17
Christopher S. Penn – 09:20
Katie Robbert – 09:41
Katie Robbert – 09:49
Katie Robbert – 09:57
It’s just going to look for everything because you’re looking for everything because you don’t know specifically. Otherwise, you would have said, ‘Bring me to the U.S. Tax database…’
Katie Robbert – 10:17
Katie Robbert – 10:23
Katie Robbert – 10:36
Katie Robbert – 10:44
Katie Robbert – 10:49
Katie Robbert – 10:53
Katie Robbert – 11:01
Christopher S. Penn – 11:04
Katie Robbert – 11:05
So if I’m your everyday marketer, which I am, I’m not overly technical. I understand technical theories and I understand technical practices.
But if I’m not necessarily a power user of generative AI like you are, Chris, what are some—why do I need to understand what retrieval augmented generation is? How would I use this thing?
Christopher S. Penn – 11:32
Christopher S. Penn – 11:35
Christopher S. Penn – 11:37
You are a healthcare system. You have patient data. You cannot load that to NotebookLM, but you absolutely could create a RAG system internally and then allow—within your own secured network—doctors to query all of the medical records to say, ‘Have we seen a case like this before? Hey, this person came in with these symptoms.’
Christopher S. Penn – 12:03
Christopher S. Penn – 12:04
Christopher S. Penn – 12:07
Christopher S. Penn – 12:08
For the average marketing, I’m writing a social media post. You’re not going to use RAG because there’s no point in doing that.
If you had confidential information or proprietary information that you did not feel comfortable loading into a NotebookLM, then a RAG system would make sense.
So if you were to say maybe you have a new piece of software that your company is going to be rolling out and the developers actually did their job and wrote documentation and you didn’t want Google to be aware of it—wow, I know we’re in science fiction land here—you might load that to a RAG system, say, ‘Now let me help me…
Christopher S. Penn – 12:48
Christopher S. Penn – 12:50
Or I’m an agency and I’m working with client data and our contract says we may not use third parties.
Regardless of the reason, no matter how safe you think it is, your contract says you cannot use third party. So you would build a RAG system internally for that client data and then query it because your contract says you can’t use NotebookLM.
Katie Robbert – 13:22
Katie Robbert – 13:26
Katie Robbert – 13:28
Christopher S. Penn – 13:49
Christopher S. Penn – 13:51
Christopher S. Penn – 13:53
I mean, that’s really fundamentally what Retrieval Augmented Generation is about. It’s us saying, ‘Hey, AI model, you don’t understand this topic well.’
Like, if you were writing content about SEO and you notice that AI is spitting out SEO tips from 2012, you’re like, ‘Okay, clearly you don’t know SEO as well as we do.’
You might use a RAG system to say, ‘This is what we know to be true about SEO in 2025.’
Christopher S. Penn – 14:34
Christopher S. Penn – 14:36
Katie Robbert – 14:41
Katie Robbert – 14:48
Christopher S. Penn – 14:53
Katie Robbert – 14:54
Katie Robbert – 15:05
Katie Robbert – 15:09
We’re just now understanding the proper terminology.
Katie Robbert – 15:16
Katie Robbert – 15:18
Katie Robbert – 15:28
Katie Robbert – 15:34
And that’s what I’m trying to understand is it sounds like for marketers, for B2B marketers, B2C marketers, even operations, even project managers, sales teams, the everyday, you probably don’t need a RAG system.
Katie Robbert – 15:59
Katie Robbert – 16:00
Katie Robbert – 16:12
Katie Robbert – 16:14
Katie Robbert – 16:16
Katie Robbert – 16:23
Katie Robbert – 16:26
Katie Robbert – 16:30
Katie Robbert – 16:41
Katie Robbert – 16:43
Katie Robbert – 16:46
Katie Robbert – 16:51
Christopher S. Penn – 16:55
Christopher S. Penn – 16:57
We write up, ‘Here’s what Trust Insights is, here’s who it does.’
Think of a RAG system as a system that can generate a relevant knowledge block dynamically on the fly.
Christopher S. Penn – 17:10
And we record those; we have the transcripts of those. That’s a lot. That’s basically an hour-plus of audio every week. It’s 6,000 words.
And on those calls, we discuss everything from our dogs to sales things. I would never want to try to include all 500 transcripts of the company into an AI prompt.
Christopher S. Penn – 17:40
Christopher S. Penn – 17:41
Christopher S. Penn – 17:44
I would create a database, a RAG system that would create all the relevant embeddings and things and put that there.
And then when I say, ‘What neat…
Christopher S. Penn – 17:57
Christopher S. Penn – 17:58
Christopher S. Penn – 18:02
Christopher S. Penn – 18:05
Christopher S. Penn – 18:08
Christopher S. Penn – 18:10
Christopher S. Penn – 18:16
Christopher S. Penn – 18:18
Christopher S. Penn – 18:20
Christopher S. Penn – 18:22
So that’s a really good example of where that RAG system would come into play.
If you have, for example…
Christopher S. Penn – 18:43
Christopher S. Penn – 18:46
Christopher S. Penn – 18:52
Christopher S. Penn – 18:53
And it’d be able to spit that out. And then you could have a conversation with just that knowledge block that it generated by itself.
Katie Robbert – 19:09
Katie Robbert – 19:11
Katie Robbert – 19:13
And I’m like, ‘Okay, yeah, so where’s that thing? I need that.’
But what you’re doing is you’re giving that real-world demonstration of when a retrieval augmented generation system is actually applicable.
Katie Robbert – 19:34
Katie Robbert – 19:37
Katie Robbert – 19:41
Katie Robbert – 19:42
Katie Robbert – 19:45
Katie Robbert – 19:47
Christopher S. Penn – 19:50
Christopher S. Penn – 20:07
Christopher S. Penn – 20:10
So for example, maybe you want to do a wrap-up of SEO best practices in 2025. So you go to Google deep research and OpenAI deep research and Perplexity Deep Research and you get some reports and you merge them together.
You don’t need a RAG system for that. These other tools have stepped in.
Christopher S. Penn – 20:32
Christopher S. Penn – 20:34
Yeah, you don’t need a RAG system for that because you’re providing the knowledge block.
Christopher S. Penn – 20:51
Christopher S. Penn – 20:52
Katie Robbert – 21:08
Katie Robbert – 21:12
Christopher S. Penn – 21:16
One of the biggest use cases for that is encoding, where you have a really big system, you load all of your code into your own internal RAG, and then you can have your coding agents reference your own code, figure out what code is in your code base, and then make changes to it that way. That’s a good use of that type of system.
But for the average marketer, that is ridiculous. There’s no reason to that. That’s like taking your fighter jet to the grocery store.
It’s vast overkill. When a bicycle would have done just fine.
Katie Robbert – 22:00
Katie Robbert – 22:11
Christopher S. Penn – 22:15
Christopher S. Penn – 22:30
Christopher S. Penn – 22:36
Katie Robbert – 22:38
All right, so it sounds like for everyday use, you don’t necessarily need to…
Katie Robbert – 22:54
Katie Robbert – 23:01
Katie Robbert – 23:08
Katie Robbert – 23:10
If you have proprietary data like personally identifying information, patient information, customer information—that’s where you would probably want to build…
Katie Robbert – 23:27
Katie Robbert – 23:30
Katie Robbert – 23:32
Christopher S. Penn – 23:35
Christopher S. Penn – 23:36
Christopher S. Penn – 23:37
Christopher S. Penn – 23:42
Christopher S. Penn – 23:43
And then we can talk about setting up like a Pinecone or Weaviate or a Milvus for an organization. Because there are RAG systems you can run locally on your computer that are unique to you and those are actually a really good idea, and you can talk about that on the livestream.
But then there’s the institutional version, which has much higher overhead for administration. But as we talked about in the use cases in this episode, there may be really good reasons to do that.
Katie Robbert – 24:22
Katie Robbert – 24:24
Katie Robbert – 24:27
Katie Robbert – 24:34
Katie Robbert – 24:40
Katie Robbert – 24:46
Katie Robbert – 24:47
Katie Robbert – 24:51
Christopher S. Penn – 24:52
Christopher S. Penn – 25:02
Christopher S. Penn – 25:03
All right, so if you’ve got some things you want to share about your experiences with RAG or you have questions about retrieval augmented generation, pop on by our free Slack group. Go to TrustInsights.ai/analyticsformarketers, where you and over 4,000 other marketers are asking and answering each other’s questions every single day about analytics, data science, machine learning, and AI.
And wherever it is you watch or listen to the show, if there’s a…
Christopher S. Penn – 25:29
Christopher S. Penn – 25:31
Christopher S. Penn – 25:46
Christopher S. Penn – 25:50
Christopher S. Penn – 25:52
Trust Insights services span the gamut from developing comprehensive data strategies and conducting deep-dive marketing analysis to building predictive models using tools like TensorFlow and PyTorch, and optimizing content strategies. Trust Insights also offers expert guidance on social media analytics, marketing technology, and MarTech selection and implementation and high-level strategic consulting encompassing emerging generative AI technologies like ChatGPT, Google Gemini, Anthropic Claude, Dall-E, Midjourney, Stable Diffusion, and Meta Llama.
Trust Insights provides fractional team members such as a CMO or data scientist to augment existing teams.
Christopher S. Penn – 26:55
Trust Insights are adept at leveraging cutting-edge generative AI techniques like large language models and diffusion models, yet they excel explaining complex concepts clearly through compelling narratives and visualizations—Data Storytelling. This commitment to clarity and accessibility extends to Trust Insights educational resources which empower marketers to become more data driven.
Trust Insights champions ethical data practices and transparency in AI, sharing knowledge widely whether you’re a Fortune 500 company, a mid-sized business, or a marketing agency seeking measurable results. Trust Insights offers a unique blend of technical expertise, strategic guidance, and educational resources to help you navigate the ever-evolving landscape of modern marketing and business in the age of generative AI.
Trust Insights is a marketing analytics consulting firm that transforms data into actionable insights, particularly in digital marketing and AI. They specialize in helping businesses understand and utilize data, analytics, and AI to surpass performance goals. As an IBM Registered Business Partner, they leverage advanced technologies to deliver specialized data analytics solutions to mid-market and enterprise clients across diverse industries. Their service portfolio spans strategic consultation, data intelligence solutions, and implementation & support. Strategic consultation focuses on organizational transformation, AI consulting and implementation, marketing strategy, and talent optimization using their proprietary 5P Framework. Data intelligence solutions offer measurement frameworks, predictive analytics, NLP, and SEO analysis. Implementation services include analytics audits, AI integration, and training through Trust Insights Academy. Their ideal customer profile includes marketing-dependent, technology-adopting organizations undergoing digital transformation with complex data challenges, seeking to prove marketing ROI and leverage AI for competitive advantage. Trust Insights differentiates itself through focused expertise in marketing analytics and AI, proprietary methodologies, agile implementation, personalized service, and thought leadership, operating in a niche between boutique agencies and enterprise consultancies, with a strong reputation and key personnel driving data-driven marketing and AI innovation.
166 Listeners
1,248 Listeners
252 Listeners
175 Listeners
170 Listeners
397 Listeners
151 Listeners
345 Listeners
193 Listeners
50 Listeners
28 Listeners
434 Listeners
56 Listeners
50 Listeners