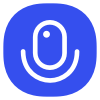
Sign up to save your podcasts
Or
As I sat in a meeting recently, a colleague shared a fascinating statistic that left me awestruck: the average car today boasts around 200 sensors, generating data in approximately 195 formats. It's incredible to think that our daily lives are now filled with such intricate information highways. Yet, despite the enormity of the data generated, many organizations are stumbling in capturing its true value. In this exploration of the IoT data economy, I am excited to unpack how we can refine this raw data into something truly innovative and market-ready.
DataScience Show is a reader-supported publication. To receive new posts and support my work, consider becoming a free or paid subscriber.
Understanding the Data Economy
Have you ever heard the phrase, “Data is the new oil”? It’s an analogy that resonates deeply in today’s digital landscape. Just like crude oil needs refinement to unlock its true potential, data requires meticulous processing to unveil its value. In this section, I want to explore how data, especially from Internet of Things (IoT) devices, can transform businesses if handled correctly.
Data as the New Oil: Why It’s Valuable
Let’s dive into the core idea that positions data as a priceless commodity. Just as nations have battled over oil reserves, companies are now competing to harness data. It’s not just about having data but knowing how to refine it. Thomas H. Davenport succinctly stated,
"Information is the new oil."
This quote underscores a crucial point: without proper refinement, data remains useless.
Consider this: an average modern car is equipped with approximately 200 sensors. Each of these sensors generates data in up to 195 different formats. This staggering amount of information becomes a tangled web of complexity. How can businesses make sense of it all? This fragmentation is a barrier to extracting valuable insights. However, with the right strategies, companies can transform this chaotic data into lucrative assets.
Complexities of the IoT Data Landscape
The IoT landscape presents unique challenges. The sheer volume of data created can be overwhelming. Here are a few complexities we face:
* Data Variety: Data comes in numerous formats, from structured numbers to unstructured text.
* Real-time Processing: Many applications need data processed instantly for timely decisions.
* Integration Issues: Different devices often operate on incompatible platforms, making it hard to consolidate data.
Organizations often struggle to maximize their IoT deployments without a solid analytics framework. It’s like trying to drive a car without knowing where the steering wheel is. Without a clear path forward, the data remains scattered and is unable to fuel operational excellence.
Importance of Structured Methodologies for Data Refinement
So, how do we turn raw data into refined products? This is where structured methodologies come into play. Just like oil refining follows a strict process, data refinement can benefit from a systematic approach. Here’s why this is crucial:
* Efficiency: Structured methodologies help streamline data collection and processing.
* Quality: Ensures that the insights derived are reliable and actionable.
* Scalability: A well-defined framework can grow with an organization’s data needs.
By adopting a refined process, businesses can focus on key metrics that matter most to their customers. Instead of gathering data haphazardly, they can target specific information that drives engagement. For instance, logistics companies might highlight delivery times while fitness trackers concentrate on calories burned.
In essence, the journey of transforming raw data into valuable insights is akin to refining crude oil. It requires careful navigating through various stages—from acquisition to analysis. As we continue to explore the data economy, let’s keep in mind that the potential for innovation lies in how we handle the data we possess.
The intricate dance between technology, methodology, and human insight defines the future of the data economy. It’s not just about having the data; it’s about understanding it, refining it, and ultimately using it to create value for ourselves and our customers.
The Seven Stages of Data Refinement
When I dive into the world of data refinement, I find myself fascinated by a structured framework that helps transform raw data into valuable products. This journey can be broken down into seven clear stages. Each stage serves a unique purpose, ensuring that no valuable insight goes unnoticed. Let's explore this refined data framework and its components.
Overview of the Refined Data Framework
At its core, the refined data framework is about turning fragmented raw sensor data into actionable insights. Just picture the average modern car. It has around 200 sensors, each generating data in about 195 different formats. It’s chaos! How can organizations possibly unlock valuable insights from such a mess? That's where the seven-stage framework comes into play. It provides a method to streamline this chaotic environment into something manageable.
Importance of Product Conceptualization
The first stage and arguably the most crucial is product conceptualization. In this phase, successful organizations avoid the common pitfall of collecting data haphazardly. Instead, they focus on identifying one core metric that truly matters to their customers. For instance, what would a logistics company prioritize? Delivery reliability, of course! Meanwhile, fitness trackers might zoom in on calories burned. This targeted approach encourages strategic data collection and sets a solid foundation for the subsequent stages.
As I reflect on this stage, I can’t help but think: Why do organizations often overlook this? It’s a simple yet powerful concept. Identifying the most critical metric can save time and resources, ultimately leading to better products. By emphasizing product conceptualization, companies can avoid drowning in a sea of irrelevant data.
Additional Stages for Contemporary IoT Complexities
As we step into the following stages, we encounter the complexities of today's Internet of Things (IoT). Initially inspired by Meyer and Zack's five-stage model, this framework has adapted to meet modern needs. Here are some critical stages that follow:
* Stage 2: Acquisition - This is where raw data is strategically collected.
* Stage 3: Refinement - In this stage, data undergoes cleaning and normalization.
* Stage 4: Storage - Here, organizations utilize cloud computing to manage vast amounts of data.
* Stage 5: Retrieval - This stage emphasizes quick access to stored data for real-time decision-making.
* Stage 6: Distribution - The focus shifts to how data is shared and presented to end-users.
* Stage 7: Market Feedback - This final stage emphasizes user engagement and iterative improvements.
As I delve deeper into these stages, I find that each builds upon the last. They create a comprehensive approach to managing data within the IoT landscape. Companies like General Electric and Progressive Insurance exemplify success in navigating these stages. They have developed sophisticated data refineries, turning raw, fragmented data into coherent, saleable products.
It’s intriguing to think about how advancements in technology have paved the way for these frameworks. The emergence of cloud computing allows organizations of all sizes to access storage solutions that were once out of reach. This democratization of technology is a game changer!
In conclusion, the journey through the seven stages of data refinement is essential for any organization wishing to harness the power of data. Emphasizing the importance of product conceptualization, along with understanding how to navigate contemporary IoT complexities, lays the groundwork for success. Every stage we explore offers new insights and opportunities, making this process not just necessary, but an exciting adventure into the world of data.
From Acquisition to Refinement: Gathering Valuable Insights
In today's data-driven world, the journey from raw data acquisition to meaningful refinement is crucial. But why is that? Well, think of data as the new oil—a valuable resource that must be properly extracted and refined to reveal its true worth.
Successful Data Acquisition Examples
Companies across industries are harnessing the power of effective data acquisition strategies. Here are a few examples:
* Logistics Companies: They often focus on key metrics like delivery reliability. By tracking data from multiple sources, they can optimize routes and improve efficiency.
* Fitness Trackers: These devices typically prioritize calories burned and activity levels. They gather specific data to help users reach their fitness goals.
By narrowing the focus on critical metrics, organizations avoid the pitfalls of data overload. Instead of collecting everything, they target what truly matters to their customers. This strategic approach forms the foundation for later stages of data refinement.
The Power of GE's Predix Platform
A prime example of successful data acquisition and refinement is General Electric's (GE) Predix platform. This innovative platform integrates diverse data from various machines, including jet engines and wind turbines, and does so efficiently. But what makes Predix stand out? It's the seamless combination of data streams that allows for real-time insights.
GE's method demonstrates how effective data acquisition isn't just about gathering data; it's about the context in which it's collected. The integration of data from machines across different sectors provides a comprehensive view, enabling better decision-making and operational efficiency.
Utilizing Edge Computing for Efficiency
Another critical aspect of data acquisition is the use of edge computing. This technology processes data closer to where it’s generated, reducing latency and bandwidth usage. Imagine trying to send a flood of data to a central server. It can take time and strain the system. Edge computing alleviates this by handling data at the source.
This approach is especially beneficial for industries reliant on real-time data. For instance, in manufacturing, machines can analyze their operational metrics on the spot, even if connectivity is lost. As a result, data remains accessible and actionable, promoting efficiency.
Choosing Critical Metrics
However, the effectiveness of any data acquisition strategy hinges on selecting the right metrics. Not all data is created equal. By focusing on what really counts, organizations can ensure they’re not just collecting scraps of information but are instead creating a wealth of valuable insights.
Take logistics, for instance. A company aiming to improve delivery performance might concentrate solely on tracking time-sensitive deliveries. This approach not only enhances operational efficiency but also elevates customer satisfaction.
Varying Company Strategies
Every organization has a unique strategy regarding data acquisition. Companies in different sectors adopt differing methods based on their specific needs and goals. For example, while a healthcare firm may prioritize patient outcomes, a retailer might focus on consumer behavior to enhance shopping experiences.
As we navigate this complex landscape, it's essential to recognize that data acquisition and refinement are not one-size-fits-all processes. Tailoring strategies to fit organizational goals can significantly impact overall effectiveness.
In summary, as I explore this intricate realm of data acquisition and refinement, I’m reminded that the journey is about more than just gathering data—it’s about understanding how to transform raw information into actionable insights. The path to becoming data-driven is paved with decisions that can make or break an organization's success.
Storage and Retrieval: The Cloud Revolution
As I explore the Storage and Retrieval stages, I recognize a paradigm shift in data management propelled by cloud computing. This shift has transformed how organizations handle their data. The cloud offers an expansive storage solution, a game-changer for businesses of all sizes.
A Shift in Data Management Practices
Data management has evolved dramatically over the years. Traditionally, organizations relied on physical servers and on-premises solutions. This often led to *high costs* and *limited scalability*. However, with the adoption of cloud technology, companies can now access vast amounts of storage without the hefty price tag. This has leveled the playing field, especially for startups.
* Cloud computing reduces the need for physical infrastructure.
* Organizations can now scale their storage needs based on demand.
* Data retrieval has become more efficient, allowing for real-time access.
But what does this mean for small businesses? It means they can compete with larger enterprises. They can now access technologies that were once out of reach. Imagine a small startup being able to handle data as efficiently as a multinational corporation. That’s the power of the cloud.
The Role of Cloud Computing in Democratizing Access
Cloud computing has democratized access to sophisticated data management tools. In the past, only large companies had the resources to invest in complex data systems. Now, these systems are available to everyone.
Here’s why this matters:
* Cost Efficiency: The cloud eliminates the need for expensive hardware. This allows even startups with minimal budgets to leverage state-of-the-art technology.
* Scalability: Businesses can easily adjust their storage capacity. This flexibility means they can grow without facing huge financial hurdles.
* Accessibility: Team members can access data from anywhere, promoting collaboration and efficiency.
Now, we have the luxury of choosing from various platforms tailored to business needs. Whether you’re a tech whiz or just starting, there’s a cloud solution out there for you.
Benefits of Time-Series Databases
When we talk about data management, we can't ignore the significance of time-series databases. These specialized databases are crucial for real-time decision-making. They allow organizations to record, analyze, and visualize data points collected over time.
Here are some key benefits:
* Real-Time Insights: Time-series databases enable organizations to monitor changes as they happen. This is essential for sectors like finance and healthcare.
* Efficient Data Handling: They are optimized for handling large volumes of data, making it easier to track trends and patterns.
* Predictive Analytics: With the right data, organizations can anticipate future trends, influencing strategic decisions.
For example, companies can monitor equipment performance in real time. They can predict failures before they happen, saving time and costs. Imagine a manufacturing unit that prevents downtime by anticipating machine failures—this is just one of the many ways time-series databases revolutionize data management.
In conclusion, the cloud revolution is reshaping how we think about storage and retrieval. Organizations that embrace these new practices stand to gain a competitive edge. As we move forward, it’s clear that the future of data management lies in the cloud. With cost-effective, scalable solutions at our fingertips, the possibilities are endless. How will you leverage this change?
Distribution and Presentation: User Experience Matters
In today's data-driven world, the success of a product hinges on many factors. One of the most crucial is user experience. As I've delved deeper into this topic, I've come to realize just how significant the distribution and presentation of data can be. When we talk about successful data products, we must recognize that they typically prioritize user-focused design.
Deciding Factors in Data Product Success
So, what makes a data product successful? It's not just about having accurate data or advanced analytics. The user experience plays a vital role. Key factors that contribute to success include:
* User Interface (UI) Design: A clean, intuitive interface can dramatically enhance user engagement.
* Accessibility: Products that cater to a diverse audience tend to perform better.
* Mobile-Friendliness: With the rise of mobile device usage, ensuring data products are optimized for smartphones is essential.
It's fascinating to see how a well-thought-out design can make or break a product. Have you ever struggled with a confusing app? You’re not alone! Poor design leads to frustration, and ultimately, user drop-off.
Case Study: LinkedIn's Data Features
Let’s take LinkedIn as a case study. Their data features, like “People You May Know,” are a great example of user-centric design in action. They’ve refined this feature over time based on user feedback. By analyzing interactions and preferences, LinkedIn has made it easier for users to connect with others.
What stands out is their commitment to iterative improvement. They don't just launch a feature and forget about it. Instead, they constantly seek ways to enhance user experience. This approach has led to increased engagement on their platform. Isn't it interesting how a simple change can lead to significant results?
The Impact of Visual Design on User Engagement
Now let’s discuss visual design. How does it impact user engagement? Consider this: when data is presented in a visually appealing manner, users are more likely to interact with it. Think about the last time you used a dashboard that was cluttered and hard to read. Frustrating, right?
On the other hand, well-designed visuals can simplify complex data, making it easier for users to cherish insights. Here are some ways visual design impacts engagement:
* Clarity: Clear visuals help users understand data quickly.
* Attractiveness: Aesthetically pleasing designs draw users in.
* Interactivity: Engaging visuals encourage users to explore data further.
Industries like transportation and healthcare have a lot to gain from good design. For example, in transportation, clear maps and real-time data visualization can drastically improve user experience. In healthcare, patient dashboards that display critical health metrics effectively can enhance patient engagement.
The Importance of Mobile-First Solutions
As we navigate through this digital era, we must communicate the importance of mobile-first solutions. More users access data on mobile devices than ever before. Ignoring this trend can be a costly mistake. Mobile-first design ensures that products are responsive, making data accessible anytime, anywhere.
In conclusion, the relationship between interface design and user uptake is undeniable. As we refine our approaches to data distribution and presentation, we must prioritize user experience. After all, a product that resonates with its users is more likely to succeed.
Iterative Improvement through Market Feedback
At the heart of product development lies a vital yet often overlooked factor—market feedback. Engaging users for continual enhancement isn't just a good practice; it’s the lifeblood of creating products that resonate. Have you ever wondered why some products thrive while others flop? The answer often lies in how well companies listen to their users.
Strategies for Collecting Actionable User Feedback
One of the first steps is to establish effective channels for gathering user insights. Here are a few strategies that I find particularly useful:
* Surveys and Polls: Short, targeted surveys can yield valuable insights. Don’t overwhelm your users. Keep questions concise.
* User Testing: Observing users interact with your product can reveal pain points you never considered.
* Feedback Forms: Simple forms on your website or app can encourage users to share their thoughts anytime.
These methods not only provide data but also create a sense of involvement among users. When they see their feedback implemented, it reinforces their loyalty and trust. Who doesn’t love feeling heard?
The Success Story of Progressive Insurance
One company that exemplifies the power of market feedback is Progressive Insurance. Through its innovative Snapshot program, Progressive collects real-time driving data from users. This isn’t just a gimmick; it fundamentally changes how they calculate insurance premiums. By using behavior data, they can offer personalized rates based on actual driving habits. Isn’t that a game changer?
They’ve turned the old, cumbersome model of flat-rate insurance on its head, creating a more equitable system. Their success stems from listening to their customers and adapting accordingly. So, what can we learn from Progressive? Listening leads to innovation.
Reimagining Industry Standards through Behavior Data
Now, let’s talk about the broader implications of using behavior data. It’s not just about making money; it’s about raising industry standards. By leveraging insights gathered from user interactions, companies can create products that not only meet but exceed expectations.
For example, in the insurance industry, behavior data is reshaping how pricing models are constructed. No longer do companies need to rely solely on historical data. Instead, they can assess live behavior patterns, leading to fairer pricing for safe drivers and potentially lower costs overall. This is a win-win, isn’t it?
The Importance of Ongoing User Engagement
Engaging users isn’t a one-time event; it’s an ongoing relationship. Continuous interaction helps to create a feedback loop. When users feel that their opinions matter, they’re more likely to stay loyal. They become advocates for your brand. Think about how companies like Apple cultivate a community around their products. Users aren't just customers; they’re part of a family. How can we foster that same sense of belonging?
Incorporating feedback loops into the development process allows for iterative improvement. Imagine launching an app, gathering user feedback, making adjustments, and then repeating the cycle. Each iteration leads to a better product. And that’s the goal, right? To create something that genuinely solves problems and delights users.
By the time I reach the final stages—Market Feedback—I observe how iterative improvement and active user engagement can breathe life into otherwise stagnant products. It’s clear that organizations investing in this approach see better outcomes. The case of Progressive Insurance shows us that user-driven data can redefine industry standards and create deeper customer relationships.
Ethics and Considerations in IoT Data Monetization
As I delve into the world of IoT data monetization, I'm struck by the ethical considerations that emerge. The potential for innovation is enormous, yet it comes with significant responsibilities. It's essential to navigate the intricate web of data ownership and consent. Questions about who owns the data we collect and how it's used are increasingly pressing.
Navigating Data Ownership and Consent
First, let’s talk about data ownership. In many cases, data generated by IoT devices belongs to the user. However, when it comes to monetization, things can get tricky. Who truly owns the data, the user or the company that collects it? It's a debate that companies must engage with transparently.
* Consent is Key: Users must give their permission for companies to use their data. This shouldn’t just be a checkbox on a form; it should be an ongoing conversation. Think about it: would you lend your car to someone without knowing how they'll drive it?
* Transparency Builds Trust: Companies should clearly communicate what data they collect and how it will be used. When users understand this, they're more likely to engage.
Implications of GDPR and CCPA
Next, let’s consider the implications of regulations like the GDPR (General Data Protection Regulation) and CCPA (California Consumer Privacy Act). These frameworks are designed to protect user data and privacy. They impose strict requirements on businesses regarding data handling.
* Rights of the Users: GDPR, for example, grants users rights to access their data and request deletion. This puts power back in the hands of consumers, which is crucial in building trust.
* Penalties for Non-Compliance: Companies that fail to comply with these regulations face hefty fines. This creates a real incentive to prioritize ethical practices.
But with regulations come challenges. For many companies, adapting to the constantly evolving legislative landscape can feel daunting. It raises a question: how can businesses remain competitive while adhering to these laws?
Building User Trust in Data-Driven Products
Finally, trust is the foundation of successful data monetization. If users don't trust a brand, they won’t share their data, and without data, monetization efforts will falter. Building this trust involves a multifaceted approach:
* Prioritize Privacy: Implement robust security measures to protect user data. This shows users that their information is safe with you.
* Continuous Engagement: Keep the lines of communication open. Regular updates about how their data is used can reassure users.
* Implement Ethical Frameworks: Adopt ethical frameworks that prioritize user welfare. This isn’t just about compliance; it’s about creating a culture of responsibility.
Incorporating these practices can help companies not just to comply with regulations, but to genuinely connect with their users. As I reflect on the complex landscape of IoT data monetization, the balance between innovation and ethics becomes increasingly clear.
“In the world of IoT, data is the new oil. But, like oil, it must be refined and handled with care.”
With every section, I become increasingly aware of the ethical considerations surrounding IoT data monetization. It’s not just about profits; it’s about building a sustainable and trust-based relationship with users. The narrative of data ownership, consent, and ethical monetization is crucial for the future of the industry.
Thanks for reading DataScience Show! This post is public so feel free to share it.
Conclusion: The Future of the IoT Data Economy
As I take a moment to reflect on the journey we have explored together, I am struck by the profound changes shaping the future of the IoT data economy. This transformation isn't just a technical shift; it's about how we democratize technology for businesses of all sizes. The barriers that once kept smaller enterprises from accessing cutting-edge tools are crumbling. Now, organizations can harness the power of IoT without needing a massive budget or extensive resources.
The Democratization of Technology
Imagine a world where a small startup can access the same advanced data analytics tools as a Fortune 500 company. That world is becoming a reality today. The emergence of cloud computing and open-source platforms has enabled even the tiniest businesses to leverage IoT technologies effectively. They can now optimize their operations, understand customer behaviors, and innovate their services in ways previously thought impossible. With affordable access to sophisticated tools, the playing field is leveling.
Integrating User Needs and Innovations
However, technology alone is not enough. We must focus on integrating user needs into our innovations. What does this mean? It’s about understanding our customers deeply—what they want, what they value, and how they interact with our products. By centering our development around these insights, we create solutions that genuinely resonate with users. Think of a fitness tracker: it’s not just about counting steps. It’s about helping users achieve their health goals in a meaningful way. This approach transforms raw data into valuable insights that enhance user experiences.
Balancing Technology and Ethics
Yet, with great power comes great responsibility. As we embrace technology, we must also address the ethical implications that accompany it. The conversations around data ownership, privacy, and consent are more important than ever. We need to ask ourselves: How do we build trust with our users? How do we ensure their data is protected while providing personalized experiences? Frameworks like GDPR and CCPA aim to navigate these complexities, but they also highlight the challenges we face.
As I consider the implications of adaptive technologies, it becomes clear that all companies must be vigilant. They need to balance innovation with a commitment to ethical practices. This balance is not just a nice-to-have; it's essential for maintaining user trust and fostering long-term relationships. When companies prioritize ethical standards, they don't just comply with regulations—they also cultivate loyalty among their customers.
In conclusion, the journey from raw data to real value is intricate but rewarding. It's about refining and transforming information into insights that drive decisions and create new opportunities. I believe that the future of the IoT data economy rests on our ability to combine technological advancements with a genuine understanding of human insights. As we move forward, let’s keep this holistic view at the forefront. The future is bright for those who embrace this journey and are willing to adapt, innovate, and prioritize the needs of their users.
Let’s celebrate the potential that lies ahead and commit to creating a data-driven landscape that is equitable, ethical, and enriching for all.
As I sat in a meeting recently, a colleague shared a fascinating statistic that left me awestruck: the average car today boasts around 200 sensors, generating data in approximately 195 formats. It's incredible to think that our daily lives are now filled with such intricate information highways. Yet, despite the enormity of the data generated, many organizations are stumbling in capturing its true value. In this exploration of the IoT data economy, I am excited to unpack how we can refine this raw data into something truly innovative and market-ready.
DataScience Show is a reader-supported publication. To receive new posts and support my work, consider becoming a free or paid subscriber.
Understanding the Data Economy
Have you ever heard the phrase, “Data is the new oil”? It’s an analogy that resonates deeply in today’s digital landscape. Just like crude oil needs refinement to unlock its true potential, data requires meticulous processing to unveil its value. In this section, I want to explore how data, especially from Internet of Things (IoT) devices, can transform businesses if handled correctly.
Data as the New Oil: Why It’s Valuable
Let’s dive into the core idea that positions data as a priceless commodity. Just as nations have battled over oil reserves, companies are now competing to harness data. It’s not just about having data but knowing how to refine it. Thomas H. Davenport succinctly stated,
"Information is the new oil."
This quote underscores a crucial point: without proper refinement, data remains useless.
Consider this: an average modern car is equipped with approximately 200 sensors. Each of these sensors generates data in up to 195 different formats. This staggering amount of information becomes a tangled web of complexity. How can businesses make sense of it all? This fragmentation is a barrier to extracting valuable insights. However, with the right strategies, companies can transform this chaotic data into lucrative assets.
Complexities of the IoT Data Landscape
The IoT landscape presents unique challenges. The sheer volume of data created can be overwhelming. Here are a few complexities we face:
* Data Variety: Data comes in numerous formats, from structured numbers to unstructured text.
* Real-time Processing: Many applications need data processed instantly for timely decisions.
* Integration Issues: Different devices often operate on incompatible platforms, making it hard to consolidate data.
Organizations often struggle to maximize their IoT deployments without a solid analytics framework. It’s like trying to drive a car without knowing where the steering wheel is. Without a clear path forward, the data remains scattered and is unable to fuel operational excellence.
Importance of Structured Methodologies for Data Refinement
So, how do we turn raw data into refined products? This is where structured methodologies come into play. Just like oil refining follows a strict process, data refinement can benefit from a systematic approach. Here’s why this is crucial:
* Efficiency: Structured methodologies help streamline data collection and processing.
* Quality: Ensures that the insights derived are reliable and actionable.
* Scalability: A well-defined framework can grow with an organization’s data needs.
By adopting a refined process, businesses can focus on key metrics that matter most to their customers. Instead of gathering data haphazardly, they can target specific information that drives engagement. For instance, logistics companies might highlight delivery times while fitness trackers concentrate on calories burned.
In essence, the journey of transforming raw data into valuable insights is akin to refining crude oil. It requires careful navigating through various stages—from acquisition to analysis. As we continue to explore the data economy, let’s keep in mind that the potential for innovation lies in how we handle the data we possess.
The intricate dance between technology, methodology, and human insight defines the future of the data economy. It’s not just about having the data; it’s about understanding it, refining it, and ultimately using it to create value for ourselves and our customers.
The Seven Stages of Data Refinement
When I dive into the world of data refinement, I find myself fascinated by a structured framework that helps transform raw data into valuable products. This journey can be broken down into seven clear stages. Each stage serves a unique purpose, ensuring that no valuable insight goes unnoticed. Let's explore this refined data framework and its components.
Overview of the Refined Data Framework
At its core, the refined data framework is about turning fragmented raw sensor data into actionable insights. Just picture the average modern car. It has around 200 sensors, each generating data in about 195 different formats. It’s chaos! How can organizations possibly unlock valuable insights from such a mess? That's where the seven-stage framework comes into play. It provides a method to streamline this chaotic environment into something manageable.
Importance of Product Conceptualization
The first stage and arguably the most crucial is product conceptualization. In this phase, successful organizations avoid the common pitfall of collecting data haphazardly. Instead, they focus on identifying one core metric that truly matters to their customers. For instance, what would a logistics company prioritize? Delivery reliability, of course! Meanwhile, fitness trackers might zoom in on calories burned. This targeted approach encourages strategic data collection and sets a solid foundation for the subsequent stages.
As I reflect on this stage, I can’t help but think: Why do organizations often overlook this? It’s a simple yet powerful concept. Identifying the most critical metric can save time and resources, ultimately leading to better products. By emphasizing product conceptualization, companies can avoid drowning in a sea of irrelevant data.
Additional Stages for Contemporary IoT Complexities
As we step into the following stages, we encounter the complexities of today's Internet of Things (IoT). Initially inspired by Meyer and Zack's five-stage model, this framework has adapted to meet modern needs. Here are some critical stages that follow:
* Stage 2: Acquisition - This is where raw data is strategically collected.
* Stage 3: Refinement - In this stage, data undergoes cleaning and normalization.
* Stage 4: Storage - Here, organizations utilize cloud computing to manage vast amounts of data.
* Stage 5: Retrieval - This stage emphasizes quick access to stored data for real-time decision-making.
* Stage 6: Distribution - The focus shifts to how data is shared and presented to end-users.
* Stage 7: Market Feedback - This final stage emphasizes user engagement and iterative improvements.
As I delve deeper into these stages, I find that each builds upon the last. They create a comprehensive approach to managing data within the IoT landscape. Companies like General Electric and Progressive Insurance exemplify success in navigating these stages. They have developed sophisticated data refineries, turning raw, fragmented data into coherent, saleable products.
It’s intriguing to think about how advancements in technology have paved the way for these frameworks. The emergence of cloud computing allows organizations of all sizes to access storage solutions that were once out of reach. This democratization of technology is a game changer!
In conclusion, the journey through the seven stages of data refinement is essential for any organization wishing to harness the power of data. Emphasizing the importance of product conceptualization, along with understanding how to navigate contemporary IoT complexities, lays the groundwork for success. Every stage we explore offers new insights and opportunities, making this process not just necessary, but an exciting adventure into the world of data.
From Acquisition to Refinement: Gathering Valuable Insights
In today's data-driven world, the journey from raw data acquisition to meaningful refinement is crucial. But why is that? Well, think of data as the new oil—a valuable resource that must be properly extracted and refined to reveal its true worth.
Successful Data Acquisition Examples
Companies across industries are harnessing the power of effective data acquisition strategies. Here are a few examples:
* Logistics Companies: They often focus on key metrics like delivery reliability. By tracking data from multiple sources, they can optimize routes and improve efficiency.
* Fitness Trackers: These devices typically prioritize calories burned and activity levels. They gather specific data to help users reach their fitness goals.
By narrowing the focus on critical metrics, organizations avoid the pitfalls of data overload. Instead of collecting everything, they target what truly matters to their customers. This strategic approach forms the foundation for later stages of data refinement.
The Power of GE's Predix Platform
A prime example of successful data acquisition and refinement is General Electric's (GE) Predix platform. This innovative platform integrates diverse data from various machines, including jet engines and wind turbines, and does so efficiently. But what makes Predix stand out? It's the seamless combination of data streams that allows for real-time insights.
GE's method demonstrates how effective data acquisition isn't just about gathering data; it's about the context in which it's collected. The integration of data from machines across different sectors provides a comprehensive view, enabling better decision-making and operational efficiency.
Utilizing Edge Computing for Efficiency
Another critical aspect of data acquisition is the use of edge computing. This technology processes data closer to where it’s generated, reducing latency and bandwidth usage. Imagine trying to send a flood of data to a central server. It can take time and strain the system. Edge computing alleviates this by handling data at the source.
This approach is especially beneficial for industries reliant on real-time data. For instance, in manufacturing, machines can analyze their operational metrics on the spot, even if connectivity is lost. As a result, data remains accessible and actionable, promoting efficiency.
Choosing Critical Metrics
However, the effectiveness of any data acquisition strategy hinges on selecting the right metrics. Not all data is created equal. By focusing on what really counts, organizations can ensure they’re not just collecting scraps of information but are instead creating a wealth of valuable insights.
Take logistics, for instance. A company aiming to improve delivery performance might concentrate solely on tracking time-sensitive deliveries. This approach not only enhances operational efficiency but also elevates customer satisfaction.
Varying Company Strategies
Every organization has a unique strategy regarding data acquisition. Companies in different sectors adopt differing methods based on their specific needs and goals. For example, while a healthcare firm may prioritize patient outcomes, a retailer might focus on consumer behavior to enhance shopping experiences.
As we navigate this complex landscape, it's essential to recognize that data acquisition and refinement are not one-size-fits-all processes. Tailoring strategies to fit organizational goals can significantly impact overall effectiveness.
In summary, as I explore this intricate realm of data acquisition and refinement, I’m reminded that the journey is about more than just gathering data—it’s about understanding how to transform raw information into actionable insights. The path to becoming data-driven is paved with decisions that can make or break an organization's success.
Storage and Retrieval: The Cloud Revolution
As I explore the Storage and Retrieval stages, I recognize a paradigm shift in data management propelled by cloud computing. This shift has transformed how organizations handle their data. The cloud offers an expansive storage solution, a game-changer for businesses of all sizes.
A Shift in Data Management Practices
Data management has evolved dramatically over the years. Traditionally, organizations relied on physical servers and on-premises solutions. This often led to *high costs* and *limited scalability*. However, with the adoption of cloud technology, companies can now access vast amounts of storage without the hefty price tag. This has leveled the playing field, especially for startups.
* Cloud computing reduces the need for physical infrastructure.
* Organizations can now scale their storage needs based on demand.
* Data retrieval has become more efficient, allowing for real-time access.
But what does this mean for small businesses? It means they can compete with larger enterprises. They can now access technologies that were once out of reach. Imagine a small startup being able to handle data as efficiently as a multinational corporation. That’s the power of the cloud.
The Role of Cloud Computing in Democratizing Access
Cloud computing has democratized access to sophisticated data management tools. In the past, only large companies had the resources to invest in complex data systems. Now, these systems are available to everyone.
Here’s why this matters:
* Cost Efficiency: The cloud eliminates the need for expensive hardware. This allows even startups with minimal budgets to leverage state-of-the-art technology.
* Scalability: Businesses can easily adjust their storage capacity. This flexibility means they can grow without facing huge financial hurdles.
* Accessibility: Team members can access data from anywhere, promoting collaboration and efficiency.
Now, we have the luxury of choosing from various platforms tailored to business needs. Whether you’re a tech whiz or just starting, there’s a cloud solution out there for you.
Benefits of Time-Series Databases
When we talk about data management, we can't ignore the significance of time-series databases. These specialized databases are crucial for real-time decision-making. They allow organizations to record, analyze, and visualize data points collected over time.
Here are some key benefits:
* Real-Time Insights: Time-series databases enable organizations to monitor changes as they happen. This is essential for sectors like finance and healthcare.
* Efficient Data Handling: They are optimized for handling large volumes of data, making it easier to track trends and patterns.
* Predictive Analytics: With the right data, organizations can anticipate future trends, influencing strategic decisions.
For example, companies can monitor equipment performance in real time. They can predict failures before they happen, saving time and costs. Imagine a manufacturing unit that prevents downtime by anticipating machine failures—this is just one of the many ways time-series databases revolutionize data management.
In conclusion, the cloud revolution is reshaping how we think about storage and retrieval. Organizations that embrace these new practices stand to gain a competitive edge. As we move forward, it’s clear that the future of data management lies in the cloud. With cost-effective, scalable solutions at our fingertips, the possibilities are endless. How will you leverage this change?
Distribution and Presentation: User Experience Matters
In today's data-driven world, the success of a product hinges on many factors. One of the most crucial is user experience. As I've delved deeper into this topic, I've come to realize just how significant the distribution and presentation of data can be. When we talk about successful data products, we must recognize that they typically prioritize user-focused design.
Deciding Factors in Data Product Success
So, what makes a data product successful? It's not just about having accurate data or advanced analytics. The user experience plays a vital role. Key factors that contribute to success include:
* User Interface (UI) Design: A clean, intuitive interface can dramatically enhance user engagement.
* Accessibility: Products that cater to a diverse audience tend to perform better.
* Mobile-Friendliness: With the rise of mobile device usage, ensuring data products are optimized for smartphones is essential.
It's fascinating to see how a well-thought-out design can make or break a product. Have you ever struggled with a confusing app? You’re not alone! Poor design leads to frustration, and ultimately, user drop-off.
Case Study: LinkedIn's Data Features
Let’s take LinkedIn as a case study. Their data features, like “People You May Know,” are a great example of user-centric design in action. They’ve refined this feature over time based on user feedback. By analyzing interactions and preferences, LinkedIn has made it easier for users to connect with others.
What stands out is their commitment to iterative improvement. They don't just launch a feature and forget about it. Instead, they constantly seek ways to enhance user experience. This approach has led to increased engagement on their platform. Isn't it interesting how a simple change can lead to significant results?
The Impact of Visual Design on User Engagement
Now let’s discuss visual design. How does it impact user engagement? Consider this: when data is presented in a visually appealing manner, users are more likely to interact with it. Think about the last time you used a dashboard that was cluttered and hard to read. Frustrating, right?
On the other hand, well-designed visuals can simplify complex data, making it easier for users to cherish insights. Here are some ways visual design impacts engagement:
* Clarity: Clear visuals help users understand data quickly.
* Attractiveness: Aesthetically pleasing designs draw users in.
* Interactivity: Engaging visuals encourage users to explore data further.
Industries like transportation and healthcare have a lot to gain from good design. For example, in transportation, clear maps and real-time data visualization can drastically improve user experience. In healthcare, patient dashboards that display critical health metrics effectively can enhance patient engagement.
The Importance of Mobile-First Solutions
As we navigate through this digital era, we must communicate the importance of mobile-first solutions. More users access data on mobile devices than ever before. Ignoring this trend can be a costly mistake. Mobile-first design ensures that products are responsive, making data accessible anytime, anywhere.
In conclusion, the relationship between interface design and user uptake is undeniable. As we refine our approaches to data distribution and presentation, we must prioritize user experience. After all, a product that resonates with its users is more likely to succeed.
Iterative Improvement through Market Feedback
At the heart of product development lies a vital yet often overlooked factor—market feedback. Engaging users for continual enhancement isn't just a good practice; it’s the lifeblood of creating products that resonate. Have you ever wondered why some products thrive while others flop? The answer often lies in how well companies listen to their users.
Strategies for Collecting Actionable User Feedback
One of the first steps is to establish effective channels for gathering user insights. Here are a few strategies that I find particularly useful:
* Surveys and Polls: Short, targeted surveys can yield valuable insights. Don’t overwhelm your users. Keep questions concise.
* User Testing: Observing users interact with your product can reveal pain points you never considered.
* Feedback Forms: Simple forms on your website or app can encourage users to share their thoughts anytime.
These methods not only provide data but also create a sense of involvement among users. When they see their feedback implemented, it reinforces their loyalty and trust. Who doesn’t love feeling heard?
The Success Story of Progressive Insurance
One company that exemplifies the power of market feedback is Progressive Insurance. Through its innovative Snapshot program, Progressive collects real-time driving data from users. This isn’t just a gimmick; it fundamentally changes how they calculate insurance premiums. By using behavior data, they can offer personalized rates based on actual driving habits. Isn’t that a game changer?
They’ve turned the old, cumbersome model of flat-rate insurance on its head, creating a more equitable system. Their success stems from listening to their customers and adapting accordingly. So, what can we learn from Progressive? Listening leads to innovation.
Reimagining Industry Standards through Behavior Data
Now, let’s talk about the broader implications of using behavior data. It’s not just about making money; it’s about raising industry standards. By leveraging insights gathered from user interactions, companies can create products that not only meet but exceed expectations.
For example, in the insurance industry, behavior data is reshaping how pricing models are constructed. No longer do companies need to rely solely on historical data. Instead, they can assess live behavior patterns, leading to fairer pricing for safe drivers and potentially lower costs overall. This is a win-win, isn’t it?
The Importance of Ongoing User Engagement
Engaging users isn’t a one-time event; it’s an ongoing relationship. Continuous interaction helps to create a feedback loop. When users feel that their opinions matter, they’re more likely to stay loyal. They become advocates for your brand. Think about how companies like Apple cultivate a community around their products. Users aren't just customers; they’re part of a family. How can we foster that same sense of belonging?
Incorporating feedback loops into the development process allows for iterative improvement. Imagine launching an app, gathering user feedback, making adjustments, and then repeating the cycle. Each iteration leads to a better product. And that’s the goal, right? To create something that genuinely solves problems and delights users.
By the time I reach the final stages—Market Feedback—I observe how iterative improvement and active user engagement can breathe life into otherwise stagnant products. It’s clear that organizations investing in this approach see better outcomes. The case of Progressive Insurance shows us that user-driven data can redefine industry standards and create deeper customer relationships.
Ethics and Considerations in IoT Data Monetization
As I delve into the world of IoT data monetization, I'm struck by the ethical considerations that emerge. The potential for innovation is enormous, yet it comes with significant responsibilities. It's essential to navigate the intricate web of data ownership and consent. Questions about who owns the data we collect and how it's used are increasingly pressing.
Navigating Data Ownership and Consent
First, let’s talk about data ownership. In many cases, data generated by IoT devices belongs to the user. However, when it comes to monetization, things can get tricky. Who truly owns the data, the user or the company that collects it? It's a debate that companies must engage with transparently.
* Consent is Key: Users must give their permission for companies to use their data. This shouldn’t just be a checkbox on a form; it should be an ongoing conversation. Think about it: would you lend your car to someone without knowing how they'll drive it?
* Transparency Builds Trust: Companies should clearly communicate what data they collect and how it will be used. When users understand this, they're more likely to engage.
Implications of GDPR and CCPA
Next, let’s consider the implications of regulations like the GDPR (General Data Protection Regulation) and CCPA (California Consumer Privacy Act). These frameworks are designed to protect user data and privacy. They impose strict requirements on businesses regarding data handling.
* Rights of the Users: GDPR, for example, grants users rights to access their data and request deletion. This puts power back in the hands of consumers, which is crucial in building trust.
* Penalties for Non-Compliance: Companies that fail to comply with these regulations face hefty fines. This creates a real incentive to prioritize ethical practices.
But with regulations come challenges. For many companies, adapting to the constantly evolving legislative landscape can feel daunting. It raises a question: how can businesses remain competitive while adhering to these laws?
Building User Trust in Data-Driven Products
Finally, trust is the foundation of successful data monetization. If users don't trust a brand, they won’t share their data, and without data, monetization efforts will falter. Building this trust involves a multifaceted approach:
* Prioritize Privacy: Implement robust security measures to protect user data. This shows users that their information is safe with you.
* Continuous Engagement: Keep the lines of communication open. Regular updates about how their data is used can reassure users.
* Implement Ethical Frameworks: Adopt ethical frameworks that prioritize user welfare. This isn’t just about compliance; it’s about creating a culture of responsibility.
Incorporating these practices can help companies not just to comply with regulations, but to genuinely connect with their users. As I reflect on the complex landscape of IoT data monetization, the balance between innovation and ethics becomes increasingly clear.
“In the world of IoT, data is the new oil. But, like oil, it must be refined and handled with care.”
With every section, I become increasingly aware of the ethical considerations surrounding IoT data monetization. It’s not just about profits; it’s about building a sustainable and trust-based relationship with users. The narrative of data ownership, consent, and ethical monetization is crucial for the future of the industry.
Thanks for reading DataScience Show! This post is public so feel free to share it.
Conclusion: The Future of the IoT Data Economy
As I take a moment to reflect on the journey we have explored together, I am struck by the profound changes shaping the future of the IoT data economy. This transformation isn't just a technical shift; it's about how we democratize technology for businesses of all sizes. The barriers that once kept smaller enterprises from accessing cutting-edge tools are crumbling. Now, organizations can harness the power of IoT without needing a massive budget or extensive resources.
The Democratization of Technology
Imagine a world where a small startup can access the same advanced data analytics tools as a Fortune 500 company. That world is becoming a reality today. The emergence of cloud computing and open-source platforms has enabled even the tiniest businesses to leverage IoT technologies effectively. They can now optimize their operations, understand customer behaviors, and innovate their services in ways previously thought impossible. With affordable access to sophisticated tools, the playing field is leveling.
Integrating User Needs and Innovations
However, technology alone is not enough. We must focus on integrating user needs into our innovations. What does this mean? It’s about understanding our customers deeply—what they want, what they value, and how they interact with our products. By centering our development around these insights, we create solutions that genuinely resonate with users. Think of a fitness tracker: it’s not just about counting steps. It’s about helping users achieve their health goals in a meaningful way. This approach transforms raw data into valuable insights that enhance user experiences.
Balancing Technology and Ethics
Yet, with great power comes great responsibility. As we embrace technology, we must also address the ethical implications that accompany it. The conversations around data ownership, privacy, and consent are more important than ever. We need to ask ourselves: How do we build trust with our users? How do we ensure their data is protected while providing personalized experiences? Frameworks like GDPR and CCPA aim to navigate these complexities, but they also highlight the challenges we face.
As I consider the implications of adaptive technologies, it becomes clear that all companies must be vigilant. They need to balance innovation with a commitment to ethical practices. This balance is not just a nice-to-have; it's essential for maintaining user trust and fostering long-term relationships. When companies prioritize ethical standards, they don't just comply with regulations—they also cultivate loyalty among their customers.
In conclusion, the journey from raw data to real value is intricate but rewarding. It's about refining and transforming information into insights that drive decisions and create new opportunities. I believe that the future of the IoT data economy rests on our ability to combine technological advancements with a genuine understanding of human insights. As we move forward, let’s keep this holistic view at the forefront. The future is bright for those who embrace this journey and are willing to adapt, innovate, and prioritize the needs of their users.
Let’s celebrate the potential that lies ahead and commit to creating a data-driven landscape that is equitable, ethical, and enriching for all.