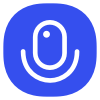
Sign up to save your podcasts
Or
This is the audio version of the essay I published on Monday.
I'm excited to finally share why I've joined Temporal.io as Head of Developer Experience. It's taken me months to precisely pin down why I have been obsessed with Workflows in general and Temporal in particular.
It boils down to 3 core opinions: Orchestration, Event Sourcing, and Workflows-as-Code.
Target audience: product-focused developers who have some understanding of system design, but limited distributed systems experience and no familiarity with workflow engines
30 Second Pitch
The most valuable, mission-critical workloads in any software company are long-running and tie together multiple services.
Finally, you want all this to scale. The same programming model going from small usecases to millions of users without re-platforming. Temporal is the best way to do all this — by writing idiomatic code known as "workflows".
Requirement 1: Orchestration
Suppose you are executing some business logic that calls System A, then System B, and then System C. Easy enough right?
But:
You could deal with B by just looping until you get a successful response, but that ties up compute resources. Probably the better way is to persist the incomplete task in a database and set a cron job to periodically retry the call.
Dealing with C is similar, but with a twist. You still need B's code to retry the API call, but you also need another (shorter lived, independent) scheduler to place a reasonable timeout on C's execution time since it doesn't report failures when it goes down.
Do this often enough and you soon realize that writing timeouts and retries are really standard production-grade requirements when crossing any system boundary, whether you are calling an external API or just a different service owned by your own team.
Instead of writing custom code for timeout and retries for every single service every time, is there a better way? Sure, we could centralize it!
We have just rediscovered the need for orchestration over choreography. There are various names for the combined A-B-C system orchestration we are doing — depending who you ask, this is either called a Job Runner, Pipeline, or Workflow.
Honestly, what interests me (more than the deduplication of code) is the deduplication of infrastructure. The maintainer of each system no longer has to provision the additional infrastructure needed for this stateful, potentially long-running work. This drastically simplifies maintenance — you can shrink your systems down to as small as a single serverless function — and makes it easier to spin up new ones, with the retry and timeout standards you now expect from every production-grade service. Workflow orchestrators are "reliability on rails".
But there's a risk of course — you've just added a centralized dependency to every part of your distributed system. What if it ALSO goes down?
Requirement 2: Event Sourcing
The work that your code does is mission critical. What does that really mean?
There are two ways to track all this state. The usual way starts with a simple task queue, and then adds logging:
But logs-as-afterthought has a bunch of problems.
The alternative to logs-as-afterthought is logs-as-tr...
5
11 ratings
This is the audio version of the essay I published on Monday.
I'm excited to finally share why I've joined Temporal.io as Head of Developer Experience. It's taken me months to precisely pin down why I have been obsessed with Workflows in general and Temporal in particular.
It boils down to 3 core opinions: Orchestration, Event Sourcing, and Workflows-as-Code.
Target audience: product-focused developers who have some understanding of system design, but limited distributed systems experience and no familiarity with workflow engines
30 Second Pitch
The most valuable, mission-critical workloads in any software company are long-running and tie together multiple services.
Finally, you want all this to scale. The same programming model going from small usecases to millions of users without re-platforming. Temporal is the best way to do all this — by writing idiomatic code known as "workflows".
Requirement 1: Orchestration
Suppose you are executing some business logic that calls System A, then System B, and then System C. Easy enough right?
But:
You could deal with B by just looping until you get a successful response, but that ties up compute resources. Probably the better way is to persist the incomplete task in a database and set a cron job to periodically retry the call.
Dealing with C is similar, but with a twist. You still need B's code to retry the API call, but you also need another (shorter lived, independent) scheduler to place a reasonable timeout on C's execution time since it doesn't report failures when it goes down.
Do this often enough and you soon realize that writing timeouts and retries are really standard production-grade requirements when crossing any system boundary, whether you are calling an external API or just a different service owned by your own team.
Instead of writing custom code for timeout and retries for every single service every time, is there a better way? Sure, we could centralize it!
We have just rediscovered the need for orchestration over choreography. There are various names for the combined A-B-C system orchestration we are doing — depending who you ask, this is either called a Job Runner, Pipeline, or Workflow.
Honestly, what interests me (more than the deduplication of code) is the deduplication of infrastructure. The maintainer of each system no longer has to provision the additional infrastructure needed for this stateful, potentially long-running work. This drastically simplifies maintenance — you can shrink your systems down to as small as a single serverless function — and makes it easier to spin up new ones, with the retry and timeout standards you now expect from every production-grade service. Workflow orchestrators are "reliability on rails".
But there's a risk of course — you've just added a centralized dependency to every part of your distributed system. What if it ALSO goes down?
Requirement 2: Event Sourcing
The work that your code does is mission critical. What does that really mean?
There are two ways to track all this state. The usual way starts with a simple task queue, and then adds logging:
But logs-as-afterthought has a bunch of problems.
The alternative to logs-as-afterthought is logs-as-tr...
1,492 Listeners
111,115 Listeners
48,072 Listeners
990 Listeners
637 Listeners
266 Listeners