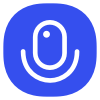
Sign up to save your podcasts
Or
In this episode of the Data Show, I spoke with Jason Dai, CTO of big data technologies at Intel, and co-chair of Strata + Hadoop World Beijing. Dai and his team are prolific and longstanding contributors to the Apache Spark project. Their early contributions to Spark tended to be on the systems side and included Netty-based shuffle, a fair-scheduler, and the “yarn-client” mode. Recently, they have been contributing tools for advanced analytics. In partnership with major cloud providers in China, they’ve written implementations of algorithmic building blocks and machine learning models that let Apache Spark users scale to extremely high-dimensional models and large data sets. They achieve scalability by taking advantage of things like data sparsity and Intel’s MKL software. Along the way, they’ve gained valuable experience and insight into how companies deploy machine learning models in real-world applications.
4
6363 ratings
In this episode of the Data Show, I spoke with Jason Dai, CTO of big data technologies at Intel, and co-chair of Strata + Hadoop World Beijing. Dai and his team are prolific and longstanding contributors to the Apache Spark project. Their early contributions to Spark tended to be on the systems side and included Netty-based shuffle, a fair-scheduler, and the “yarn-client” mode. Recently, they have been contributing tools for advanced analytics. In partnership with major cloud providers in China, they’ve written implementations of algorithmic building blocks and machine learning models that let Apache Spark users scale to extremely high-dimensional models and large data sets. They achieve scalability by taking advantage of things like data sparsity and Intel’s MKL software. Along the way, they’ve gained valuable experience and insight into how companies deploy machine learning models in real-world applications.
285 Listeners
35 Listeners
475 Listeners
580 Listeners
624 Listeners
8 Listeners
203 Listeners
295 Listeners
214 Listeners
139 Listeners
266 Listeners
196 Listeners
188 Listeners
101 Listeners
139 Listeners
178 Listeners
397 Listeners