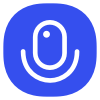
Sign up to save your podcasts
Or
Building on the discussion of individual decision trees in the prior episode, Shea and Anders shift to one of today’s most popular ensemble models, the Random Forest. At first glance, the algorithm may seem like a brute force approach of simply running hundreds or thousands of decision trees, but it leverages the concept of “bagging” to avoid overfitting and attempt to learn as much as possible from the entire data sets, not just a few key features. We close by covering strengths and weaknesses of this model and providing some real-life examples.
4.6
3030 ratings
Building on the discussion of individual decision trees in the prior episode, Shea and Anders shift to one of today’s most popular ensemble models, the Random Forest. At first glance, the algorithm may seem like a brute force approach of simply running hundreds or thousands of decision trees, but it leverages the concept of “bagging” to avoid overfitting and attempt to learn as much as possible from the entire data sets, not just a few key features. We close by covering strengths and weaknesses of this model and providing some real-life examples.
237 Listeners
8,514 Listeners
30,853 Listeners
32,121 Listeners
112,814 Listeners
56,140 Listeners
9,521 Listeners
266 Listeners
9,241 Listeners
16 Listeners
9,693 Listeners
5,850 Listeners
8,385 Listeners
5,377 Listeners
90 Listeners