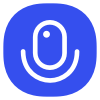
Sign up to save your podcasts
Or
Hannes is a graduate student at MIT working towards a PhD in computer science. Within the span of a few months, he has been able to publish two ICML papers: one focused on molecular property prediction and the other developing a model, called EquiBind, for drug binding prediction. Hannes, advised by both Regina Barzilay and Tommi Jaakkola, is doing exciting research at the intersection of graph machine learning and biology. In this conversation, we discuss his career so far starting at Munich to an internship at MIT that ultimately led to Hannes starting his PhD at MIT earlier this summer. For Hannes, biology is a new frontier to not only develop new ML models but have an impact on human health.
We get into the overall field of molecular dynamics and machine learning. Everything from the need for higher quality datasets in life sciences to new models. We touch upon the advantages of graph models to predict biological phenomena and the opportunity to feed these models better data. Afterwards, we go into his two papers - InfoMax and EquiBind. The former uses pre-training to capture implicit 3D structures of small molecules to predict various properties. And the latter relies on graph models to avoid thorough sampling thereby speeding up the process of predicting ligand/target binding. Both of these methods are interesting case studies on the power of graphs and the potential of relying on new models and data to map out biology rather than sheer computing power.
Finally, we talk about longer-term opportunities in ML and self-supervised learning. Everything from protein to small molecule design. For Hannes, modeling molecular interactions is the key focus he takes and he's spending more time thinking about proteins and their activity in cells. I would also recommend joining the reading group he leads on graph machine learning. From our conversation, a favorite quote of mine was Hannes focus on doing work with "an eye for good."
5
22 ratings
Hannes is a graduate student at MIT working towards a PhD in computer science. Within the span of a few months, he has been able to publish two ICML papers: one focused on molecular property prediction and the other developing a model, called EquiBind, for drug binding prediction. Hannes, advised by both Regina Barzilay and Tommi Jaakkola, is doing exciting research at the intersection of graph machine learning and biology. In this conversation, we discuss his career so far starting at Munich to an internship at MIT that ultimately led to Hannes starting his PhD at MIT earlier this summer. For Hannes, biology is a new frontier to not only develop new ML models but have an impact on human health.
We get into the overall field of molecular dynamics and machine learning. Everything from the need for higher quality datasets in life sciences to new models. We touch upon the advantages of graph models to predict biological phenomena and the opportunity to feed these models better data. Afterwards, we go into his two papers - InfoMax and EquiBind. The former uses pre-training to capture implicit 3D structures of small molecules to predict various properties. And the latter relies on graph models to avoid thorough sampling thereby speeding up the process of predicting ligand/target binding. Both of these methods are interesting case studies on the power of graphs and the potential of relying on new models and data to map out biology rather than sheer computing power.
Finally, we talk about longer-term opportunities in ML and self-supervised learning. Everything from protein to small molecule design. For Hannes, modeling molecular interactions is the key focus he takes and he's spending more time thinking about proteins and their activity in cells. I would also recommend joining the reading group he leads on graph machine learning. From our conversation, a favorite quote of mine was Hannes focus on doing work with "an eye for good."