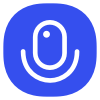
Sign up to save your podcasts
Or
Oftentimes, Kaggle competitions are looked at as an excellent way for data scientists to sharpen their machine learning skills and become technically excellent. This begs the question, what are the hallmarks of high-performing Kaggle competitors? What makes a Kaggle Grand Master?
Today’s guest, Jean-Francois Puget PhD, distinguished engineer at NVIDIA, has achieved this impressive feat three times.
Throughout the episode, Richie and Jean-Francois discuss his background and how he became a Kaggle Grandmaster. He shares his scientific approach to machine learning and how he uses this to consistently achieve high results in Kaggle competitions.
Jean-Francois also discusses how NVIDIA employs nine Kaggle Grandmasters and how they use Kaggle experiments to breed innovation in solving their machine learning challenges. He expands on the toolkit he employs in solving Kaggle competitions, and how he has achieved 50X improvements in efficiencies using tools like RAPIDS.
Richie and Jean-Francois also delve into the difference between competitive data science on Kaggle and machine learning work in a real-world setting. They deep dive into the challenges of real-world machine learning, and how to resolve the ambiguities of using machine learning in production that data scientists don’t encounter in Kaggle competitions.
4.9
264264 ratings
Oftentimes, Kaggle competitions are looked at as an excellent way for data scientists to sharpen their machine learning skills and become technically excellent. This begs the question, what are the hallmarks of high-performing Kaggle competitors? What makes a Kaggle Grand Master?
Today’s guest, Jean-Francois Puget PhD, distinguished engineer at NVIDIA, has achieved this impressive feat three times.
Throughout the episode, Richie and Jean-Francois discuss his background and how he became a Kaggle Grandmaster. He shares his scientific approach to machine learning and how he uses this to consistently achieve high results in Kaggle competitions.
Jean-Francois also discusses how NVIDIA employs nine Kaggle Grandmasters and how they use Kaggle experiments to breed innovation in solving their machine learning challenges. He expands on the toolkit he employs in solving Kaggle competitions, and how he has achieved 50X improvements in efficiencies using tools like RAPIDS.
Richie and Jean-Francois also delve into the difference between competitive data science on Kaggle and machine learning work in a real-world setting. They deep dive into the challenges of real-world machine learning, and how to resolve the ambiguities of using machine learning in production that data scientists don’t encounter in Kaggle competitions.
470 Listeners
585 Listeners
628 Listeners
296 Listeners
324 Listeners
140 Listeners
190 Listeners
63 Listeners
136 Listeners
281 Listeners
88 Listeners
190 Listeners
63 Listeners
424 Listeners
36 Listeners