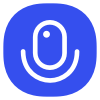
Sign up to save your podcasts
Or
Send us a text
In this episode of The MLSecOps podcast, the co-hosts interview Pin-Yu Chen, Principal Research Scientist at IBM Research, about his book co-authored with Cho-Jui Hsieh, "Adversarial Robustness for Machine Learning." Chen explores the vulnerabilities of machine learning (ML) models to adversarial attacks and provides examples of how to enhance their robustness. The discussion delves into the difference between Trustworthy AI and Trustworthy ML, as well as the concept of LLM practical attacks, which take into account the practical constraints of an attacker. Chen also discusses security measures that can be taken to protect ML systems and emphasizes the importance of considering the entire model lifecycle in terms of security. Finally, the conversation concludes with a discussion on how businesses can justify the cost and value of implementing adversarial defense methods in their ML systems.
Thanks for checking out the MLSecOps Podcast! Get involved with the MLSecOps Community and find more resources at https://community.mlsecops.com.
Additional tools and resources to check out:
Protect AI Guardian: Zero Trust for ML Models
Recon: Automated Red Teaming for GenAI
Protect AI’s ML Security-Focused Open Source Tools
LLM Guard Open Source Security Toolkit for LLM Interactions
Huntr - The World's First AI/Machine Learning Bug Bounty Platform
Send us a text
In this episode of The MLSecOps podcast, the co-hosts interview Pin-Yu Chen, Principal Research Scientist at IBM Research, about his book co-authored with Cho-Jui Hsieh, "Adversarial Robustness for Machine Learning." Chen explores the vulnerabilities of machine learning (ML) models to adversarial attacks and provides examples of how to enhance their robustness. The discussion delves into the difference between Trustworthy AI and Trustworthy ML, as well as the concept of LLM practical attacks, which take into account the practical constraints of an attacker. Chen also discusses security measures that can be taken to protect ML systems and emphasizes the importance of considering the entire model lifecycle in terms of security. Finally, the conversation concludes with a discussion on how businesses can justify the cost and value of implementing adversarial defense methods in their ML systems.
Thanks for checking out the MLSecOps Podcast! Get involved with the MLSecOps Community and find more resources at https://community.mlsecops.com.
Additional tools and resources to check out:
Protect AI Guardian: Zero Trust for ML Models
Recon: Automated Red Teaming for GenAI
Protect AI’s ML Security-Focused Open Source Tools
LLM Guard Open Source Security Toolkit for LLM Interactions
Huntr - The World's First AI/Machine Learning Bug Bounty Platform
369 Listeners
1,774 Listeners
200 Listeners
295 Listeners
213 Listeners
180 Listeners
312 Listeners
189 Listeners
76 Listeners
1,441 Listeners
760 Listeners
282 Listeners
8,760 Listeners
422 Listeners
4 Listeners