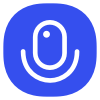
Sign up to save your podcasts
Or
In this episode I explain a very effective technique that allows one to infer the membership of any record at hand to the (private) training dataset used to train the target model. The effectiveness of such technique is due to the fact that it works on black-box models of which there is no access to the data used for training, nor model parameters and hyperparameters. Such a scenario is very realistic and typical of machine learning as a service APIs.
This episode is supported by pryml.io, a platform I am personally working on that enables data sharing without giving up confidentiality.
As promised below is the schema of the attack explained in the episode.
Membership Inference Attacks Against Machine Learning Models
4.2
7272 ratings
In this episode I explain a very effective technique that allows one to infer the membership of any record at hand to the (private) training dataset used to train the target model. The effectiveness of such technique is due to the fact that it works on black-box models of which there is no access to the data used for training, nor model parameters and hyperparameters. Such a scenario is very realistic and typical of machine learning as a service APIs.
This episode is supported by pryml.io, a platform I am personally working on that enables data sharing without giving up confidentiality.
As promised below is the schema of the attack explained in the episode.
Membership Inference Attacks Against Machine Learning Models
43,917 Listeners
11,133 Listeners
1,069 Listeners
77,562 Listeners
483 Listeners
592 Listeners
202 Listeners
298 Listeners
260 Listeners
266 Listeners
190 Listeners
2,524 Listeners
35 Listeners
2,979 Listeners
5,422 Listeners