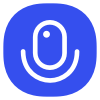
Sign up to save your podcasts
Or
A recent announcement on X boasted a tuned model with pretty outstanding performance, and claimed these results were achieved through Reflection Tuning. However, people were unable to reproduce the results. We dive into some recent drama in the AI community as a jumping off point for a discussion about Reflection 70B.
In 2023, there was a paper written about Reflection Tuning that this new model (Reflection 70B) draws concepts from. Reflection tuning is an optimization technique where models learn to improve their decision-making processes by “reflecting” on past actions or predictions. This method enables models to iteratively refine their performance by analyzing mistakes and successes, thus improving both accuracy and adaptability over time. By incorporating a feedback loop, reflection tuning can address model weaknesses more dynamically, helping AI systems become more robust in real-world applications where uncertainty or changing environments are prevalent.
Dat Ngo (AI Solutions Architect at Arize), talks to Rohan Pandey (Founding Engineer at Reworkd) about Reflection 70B, Reflection Tuning, the recent drama, and the importance of double checking your research.
Learn more about AI observability and evaluation, join the Arize AI Slack community or get the latest on LinkedIn and X.
5
1313 ratings
A recent announcement on X boasted a tuned model with pretty outstanding performance, and claimed these results were achieved through Reflection Tuning. However, people were unable to reproduce the results. We dive into some recent drama in the AI community as a jumping off point for a discussion about Reflection 70B.
In 2023, there was a paper written about Reflection Tuning that this new model (Reflection 70B) draws concepts from. Reflection tuning is an optimization technique where models learn to improve their decision-making processes by “reflecting” on past actions or predictions. This method enables models to iteratively refine their performance by analyzing mistakes and successes, thus improving both accuracy and adaptability over time. By incorporating a feedback loop, reflection tuning can address model weaknesses more dynamically, helping AI systems become more robust in real-world applications where uncertainty or changing environments are prevalent.
Dat Ngo (AI Solutions Architect at Arize), talks to Rohan Pandey (Founding Engineer at Reworkd) about Reflection 70B, Reflection Tuning, the recent drama, and the importance of double checking your research.
Learn more about AI observability and evaluation, join the Arize AI Slack community or get the latest on LinkedIn and X.
298 Listeners
331 Listeners
217 Listeners
192 Listeners
198 Listeners
298 Listeners
88 Listeners
426 Listeners
121 Listeners
142 Listeners
201 Listeners
75 Listeners
491 Listeners
31 Listeners
43 Listeners