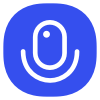
Sign up to save your podcasts
Or
Deep Papers is a podcast series featuring deep dives on today’s seminal AI papers and research. Hosted by AI Pub creator Brian Burns and Arize AI founders Jason Lopatecki and Aparna Dhinakaran, each episode profiles the people and techniques behind cutting-edge breakthroughs in machine learning.
In this episode, we interview Dan Fu and Tri Dao, inventors of "Hungry Hungry Hippos" (aka "H3"). This language modeling architecture performs comparably to transformers, while admitting much longer context length: n log(n) rather than n^2 context scaling, for those technically inclined. Listen to learn about the major ideas and history behind H3, state space models, what makes them special, what products can be built with long-context language models, and hints of Dan and Tri's future (unpublished) research.
Learn more about AI observability and evaluation, join the Arize AI Slack community or get the latest on LinkedIn and X.
5
1313 ratings
Deep Papers is a podcast series featuring deep dives on today’s seminal AI papers and research. Hosted by AI Pub creator Brian Burns and Arize AI founders Jason Lopatecki and Aparna Dhinakaran, each episode profiles the people and techniques behind cutting-edge breakthroughs in machine learning.
In this episode, we interview Dan Fu and Tri Dao, inventors of "Hungry Hungry Hippos" (aka "H3"). This language modeling architecture performs comparably to transformers, while admitting much longer context length: n log(n) rather than n^2 context scaling, for those technically inclined. Listen to learn about the major ideas and history behind H3, state space models, what makes them special, what products can be built with long-context language models, and hints of Dan and Tri's future (unpublished) research.
Learn more about AI observability and evaluation, join the Arize AI Slack community or get the latest on LinkedIn and X.
298 Listeners
331 Listeners
217 Listeners
192 Listeners
198 Listeners
298 Listeners
88 Listeners
428 Listeners
121 Listeners
142 Listeners
201 Listeners
75 Listeners
491 Listeners
31 Listeners
43 Listeners