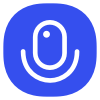
Sign up to save your podcasts
Or
This week’s paper presents a comprehensive study of the performance of various LLMs acting as judges. The researchers leverage TriviaQA as a benchmark for assessing objective knowledge reasoning of LLMs and evaluate them alongside human annotations which they find to have a high inter-annotator agreement. The study includes nine judge models and nine exam-taker models – both base and instruction-tuned. They assess the judge models’ alignment across different model sizes, families, and judge prompts to answer questions about the strengths and weaknesses of this paradigm, and what potential biases it may hold.
Read it on the blog: https://arize.com/blog/judging-the-judges-llm-as-a-judge/
Learn more about AI observability and evaluation, join the Arize AI Slack community or get the latest on LinkedIn and X.
5
1313 ratings
This week’s paper presents a comprehensive study of the performance of various LLMs acting as judges. The researchers leverage TriviaQA as a benchmark for assessing objective knowledge reasoning of LLMs and evaluate them alongside human annotations which they find to have a high inter-annotator agreement. The study includes nine judge models and nine exam-taker models – both base and instruction-tuned. They assess the judge models’ alignment across different model sizes, families, and judge prompts to answer questions about the strengths and weaknesses of this paradigm, and what potential biases it may hold.
Read it on the blog: https://arize.com/blog/judging-the-judges-llm-as-a-judge/
Learn more about AI observability and evaluation, join the Arize AI Slack community or get the latest on LinkedIn and X.
1,007 Listeners
587 Listeners
442 Listeners
296 Listeners
321 Listeners
210 Listeners
188 Listeners
90 Listeners
350 Listeners
128 Listeners
196 Listeners
72 Listeners
33 Listeners
22 Listeners
37 Listeners