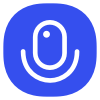
Sign up to save your podcasts
Or
LLMs have revolutionized natural language processing, showcasing remarkable versatility and capabilities. But individual LLMs often exhibit distinct strengths and weaknesses, influenced by differences in their training corpora. This diversity poses a challenge: how can we maximize the efficiency and utility of LLMs?
A new paper, "Merge, Ensemble, and Cooperate: A Survey on Collaborative Strategies in the Era of Large Language Models," highlights collaborative strategies to address this challenge. In this week's episode, we summarize key insights from this paper and discuss practical implications of LLM collaboration strategies across three main approaches: merging, ensemble, and cooperation. We also review some new open source models we're excited about.
Learn more about AI observability and evaluation, join the Arize AI Slack community or get the latest on LinkedIn and X.
5
1313 ratings
LLMs have revolutionized natural language processing, showcasing remarkable versatility and capabilities. But individual LLMs often exhibit distinct strengths and weaknesses, influenced by differences in their training corpora. This diversity poses a challenge: how can we maximize the efficiency and utility of LLMs?
A new paper, "Merge, Ensemble, and Cooperate: A Survey on Collaborative Strategies in the Era of Large Language Models," highlights collaborative strategies to address this challenge. In this week's episode, we summarize key insights from this paper and discuss practical implications of LLM collaboration strategies across three main approaches: merging, ensemble, and cooperation. We also review some new open source models we're excited about.
Learn more about AI observability and evaluation, join the Arize AI Slack community or get the latest on LinkedIn and X.
1,007 Listeners
587 Listeners
442 Listeners
296 Listeners
321 Listeners
210 Listeners
188 Listeners
90 Listeners
350 Listeners
128 Listeners
196 Listeners
72 Listeners
33 Listeners
22 Listeners
37 Listeners