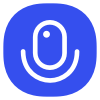
Sign up to save your podcasts
Or
This week, we’re discussing "RAG vs Fine-Tuning: Pipelines, Tradeoff, and a Case Study on Agriculture." This paper explores a pipeline for fine-tuning and RAG, and presents the tradeoffs of both for multiple popular LLMs, including Llama2-13B, GPT-3.5, and GPT-4.
The authors propose a pipeline that consists of multiple stages, including extracting information from PDFs, generating questions and answers, using them for fine-tuning, and leveraging GPT-4 for evaluating the results. Overall, the results point to how systems built using LLMs can be adapted to respond and incorporate knowledge across a dimension that is critical for a specific industry, paving the way for further applications of LLMs in other industrial domains.
Learn more about AI observability and evaluation, join the Arize AI Slack community or get the latest on LinkedIn and X.
5
1313 ratings
This week, we’re discussing "RAG vs Fine-Tuning: Pipelines, Tradeoff, and a Case Study on Agriculture." This paper explores a pipeline for fine-tuning and RAG, and presents the tradeoffs of both for multiple popular LLMs, including Llama2-13B, GPT-3.5, and GPT-4.
The authors propose a pipeline that consists of multiple stages, including extracting information from PDFs, generating questions and answers, using them for fine-tuning, and leveraging GPT-4 for evaluating the results. Overall, the results point to how systems built using LLMs can be adapted to respond and incorporate knowledge across a dimension that is critical for a specific industry, paving the way for further applications of LLMs in other industrial domains.
Learn more about AI observability and evaluation, join the Arize AI Slack community or get the latest on LinkedIn and X.
298 Listeners
331 Listeners
217 Listeners
192 Listeners
198 Listeners
298 Listeners
88 Listeners
426 Listeners
121 Listeners
142 Listeners
201 Listeners
75 Listeners
491 Listeners
31 Listeners
43 Listeners