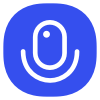
Sign up to save your podcasts
Or
For business analytics the way that you model the data in your warehouse has a lasting impact on what types of questions can be answered quickly and easily. The major strategies in use today were created decades ago when the software and hardware for warehouse databases were far more constrained. In this episode Maxime Beauchemin of Airflow and Superset fame shares his vision for the entity-centric data model and how you can incorporate it into your own warehouse design.
Can you describe what entity-centric modeling (ECM) is and the story behind it?
What impact does this have on ML teams? (e.g. feature engineering)
What role does the tooling of a team have in the ways that they end up thinking about modeling? (e.g. dbt vs. informatica vs. ETL scripts, etc.)
What are some examples of data sources or problem domains for which this approach is well suited?
What are the ways that the benefits of ECM manifest in use cases that are down-stream from the warehouse?
What are some concrete tactical steps that teams should be thinking about to implement a workable domain model using entity-centric principles?
What are the most interesting, innovative, or unexpected ways that you have seen ECM used?
What are the most interesting, unexpected, or challenging lessons that you have learned while working on ECM?
When is ECM the wrong choice?
What are your predictions for the future direction/adoption of ECM or other modeling techniques?
The intro and outro music is from The Hug by The Freak Fandango Orchestra / CC BY-SA
Sponsored By:
Support Data Engineering Podcast
4.6
135135 ratings
For business analytics the way that you model the data in your warehouse has a lasting impact on what types of questions can be answered quickly and easily. The major strategies in use today were created decades ago when the software and hardware for warehouse databases were far more constrained. In this episode Maxime Beauchemin of Airflow and Superset fame shares his vision for the entity-centric data model and how you can incorporate it into your own warehouse design.
Can you describe what entity-centric modeling (ECM) is and the story behind it?
What impact does this have on ML teams? (e.g. feature engineering)
What role does the tooling of a team have in the ways that they end up thinking about modeling? (e.g. dbt vs. informatica vs. ETL scripts, etc.)
What are some examples of data sources or problem domains for which this approach is well suited?
What are the ways that the benefits of ECM manifest in use cases that are down-stream from the warehouse?
What are some concrete tactical steps that teams should be thinking about to implement a workable domain model using entity-centric principles?
What are the most interesting, innovative, or unexpected ways that you have seen ECM used?
What are the most interesting, unexpected, or challenging lessons that you have learned while working on ECM?
When is ECM the wrong choice?
What are your predictions for the future direction/adoption of ECM or other modeling techniques?
The intro and outro music is from The Hug by The Freak Fandango Orchestra / CC BY-SA
Sponsored By:
Support Data Engineering Podcast
272 Listeners
283 Listeners
152 Listeners
42 Listeners
480 Listeners
591 Listeners
627 Listeners
441 Listeners
295 Listeners
213 Listeners
265 Listeners
189 Listeners
64 Listeners
140 Listeners
76 Listeners