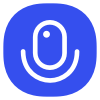
Sign up to save your podcasts
Or
With the release of Spark version 2.0, streaming starts becoming much more accessible to users. By adopting a continuous processing model (on an infinite table), the developers of Spark have enabled users of its SQL or DataFrame APIs to extend their analytic capabilities to unbounded streams.
Within the Spark community, Databricks Engineer, Michael Armbrust is well-known for having led the long-term project to move Spark’s interactive analytics engine from Shark to Spark SQL. (Full disclosure: I’m an advisor to Databricks.) Most recently he has turned his efforts to helping introduce a much simpler stream processing model to Spark Streaming (“structured streaming”).
4
6363 ratings
With the release of Spark version 2.0, streaming starts becoming much more accessible to users. By adopting a continuous processing model (on an infinite table), the developers of Spark have enabled users of its SQL or DataFrame APIs to extend their analytic capabilities to unbounded streams.
Within the Spark community, Databricks Engineer, Michael Armbrust is well-known for having led the long-term project to move Spark’s interactive analytics engine from Shark to Spark SQL. (Full disclosure: I’m an advisor to Databricks.) Most recently he has turned his efforts to helping introduce a much simpler stream processing model to Spark Streaming (“structured streaming”).
285 Listeners
35 Listeners
474 Listeners
584 Listeners
631 Listeners
8 Listeners
200 Listeners
296 Listeners
213 Listeners
140 Listeners
267 Listeners
195 Listeners
189 Listeners
99 Listeners
143 Listeners
193 Listeners
416 Listeners