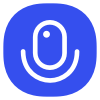
Sign up to save your podcasts
Or
Follow AI__Pub on Twitter. To learn more about ML observability, join the Arize AI Slack community or get the latest on our LinkedIn and Twitter.
Learn more about AI observability and evaluation, join the Arize AI Slack community or get the latest on LinkedIn and X.
">Deep Papers is a podcast series featuring deep dives on today’s seminal AI papers and research. Hosted by AI Pub creator Brian Burns and Arize AI founders Jason Lopatecki and Aparna Dhinakaran, each episode profiles the people and techniques behind cutting-edge breakthroughs in machine learning.
In this episode, we interview Timo Schick and Thomas Scialom, the Research Scientists at Meta AI behind Toolformer. "Vanilla" language models cannot access information about the external world. But what if we gave language models access to calculators, question-answer search, and other APIs to generate more powerful and accurate output? Further, how do we train such a model? How can we automatically generate a dataset of API-call-annotated text at internet scale, without human labeling?
Timo and Thomas give a step-by-step walkthrough of building and training Toolformer, what motivated them to do it, and what we should expect in the next generation of tool-LLM powered products.
Follow AI__Pub on Twitter. To learn more about ML observability, join the Arize AI Slack community or get the latest on our LinkedIn and Twitter.
Learn more about AI observability and evaluation, join the Arize AI Slack community or get the latest on LinkedIn and X.
5
1313 ratings
Deep Papers is a podcast series featuring deep dives on today’s seminal AI papers and research. Hosted by AI Pub creator Brian Burns and Arize AI founders Jason Lopatecki and Aparna Dhinakaran, each episode profiles the people and techniques behind cutting-edge breakthroughs in machine learning.
In this episode, we interview Timo Schick and Thomas Scialom, the Research Scientists at Meta AI behind Toolformer. "Vanilla" language models cannot access information about the external world. But what if we gave language models access to calculators, question-answer search, and other APIs to generate more powerful and accurate output? Further, how do we train such a model? How can we automatically generate a dataset of API-call-annotated text at internet scale, without human labeling?
Timo and Thomas give a step-by-step walkthrough of building and training Toolformer, what motivated them to do it, and what we should expect in the next generation of tool-LLM powered products.
Follow AI__Pub on Twitter. To learn more about ML observability, join the Arize AI Slack community or get the latest on our LinkedIn and Twitter.
Learn more about AI observability and evaluation, join the Arize AI Slack community or get the latest on LinkedIn and X.
1,281 Listeners
1,009 Listeners
475 Listeners
439 Listeners
295 Listeners
312 Listeners
196 Listeners
271 Listeners
92 Listeners
320 Listeners
106 Listeners
70 Listeners
397 Listeners
424 Listeners
31 Listeners