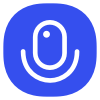
Sign up to save your podcasts
Or
Access the full transcript for this episode
Join us as we speak with three different guests, all UC Berkeley Data Science alumni, who have gone on to pursue higher education. Ranging from learning sciences to epidemiology, our guests share their experiences, challenges, and insights into how their data science education prepared them for their current paths.
Ashley Quiterio, a PhD student in Learning Sciences at Northwestern University, delves into the intersection of data science and education, highlighting the transformative potential of data-driven approaches in shaping learning environments.
“Try everything and try different things. I mentioned all these different roles [I did during undergrad], where I was trying to see where I fit, deciding what I like about data education. There's all these different lenses and different ways of thinking about where you fit. So I'd encourage people to try that out, early and often. Data science is such an interdisciplinary field that you're not going to be lacking opportunities.” — Ashley Quiterio
Anna Nguyen, a PhD student in Epidemiology and Clinical Research at Stanford University, shares her journey from data science to public health, emphasizing the importance of interdisciplinary collaboration in addressing complex health challenges.
“Regardless of what anyone says, there's no pure cut way of getting into grad school. Pursuing opportunities that allow you to really explore your interests and displaying a willingness to learn is probably the best way to prepare for a masters or a PhD program. I think I definitely overestimated how much time I had in undergrad. And the time was so limited and valuable, so it's really not worth doing things that you don't enjoy in that limited time.” — Anna Nguyen
Rodrigo Palmaka, a Masters student in Statistics at UC Berkeley, offers perspectives on computational pathology and statistical research, illustrating the versatility of data science skills in diverse research domains.
“I think I always sought to focus on the fundamentals—not overfit or pigeonhole myself too much—and give myself some flexibility to, you know, be able to adapt to the next big thing.” — Rodrigo Palmaka
Access the full transcript for this episode
Join us as we speak with three different guests, all UC Berkeley Data Science alumni, who have gone on to pursue higher education. Ranging from learning sciences to epidemiology, our guests share their experiences, challenges, and insights into how their data science education prepared them for their current paths.
Ashley Quiterio, a PhD student in Learning Sciences at Northwestern University, delves into the intersection of data science and education, highlighting the transformative potential of data-driven approaches in shaping learning environments.
“Try everything and try different things. I mentioned all these different roles [I did during undergrad], where I was trying to see where I fit, deciding what I like about data education. There's all these different lenses and different ways of thinking about where you fit. So I'd encourage people to try that out, early and often. Data science is such an interdisciplinary field that you're not going to be lacking opportunities.” — Ashley Quiterio
Anna Nguyen, a PhD student in Epidemiology and Clinical Research at Stanford University, shares her journey from data science to public health, emphasizing the importance of interdisciplinary collaboration in addressing complex health challenges.
“Regardless of what anyone says, there's no pure cut way of getting into grad school. Pursuing opportunities that allow you to really explore your interests and displaying a willingness to learn is probably the best way to prepare for a masters or a PhD program. I think I definitely overestimated how much time I had in undergrad. And the time was so limited and valuable, so it's really not worth doing things that you don't enjoy in that limited time.” — Anna Nguyen
Rodrigo Palmaka, a Masters student in Statistics at UC Berkeley, offers perspectives on computational pathology and statistical research, illustrating the versatility of data science skills in diverse research domains.
“I think I always sought to focus on the fundamentals—not overfit or pigeonhole myself too much—and give myself some flexibility to, you know, be able to adapt to the next big thing.” — Rodrigo Palmaka
7,790 Listeners
217 Listeners
285 Listeners
470 Listeners
633 Listeners
296 Listeners
611 Listeners
322 Listeners
189 Listeners
14 Listeners
5,356 Listeners
71 Listeners
25 Listeners
196 Listeners
143 Listeners