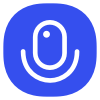
Sign up to save your podcasts
Or
Professor Swarat Chaudhuri from the University of Texas at Austin and visiting researcher at Google DeepMind discusses breakthroughs in AI reasoning, theorem proving, and mathematical discovery. Chaudhuri explains his groundbreaking work on COPRA (a GPT-based prover agent), shares insights on neurosymbolic approaches to AI.
Professor Swarat Chaudhuri:
https://www.cs.utexas.edu/~swarat/
SPONSOR MESSAGES:
CentML offers competitive pricing for GenAI model deployment, with flexible options to suit a wide range of models, from small to large-scale deployments.
https://centml.ai/pricing/
Tufa AI Labs is a brand new research lab in Zurich started by Benjamin Crouzier focussed on ARC and AGI, they just acquired MindsAI - the current winners of the ARC challenge. Are you interested in working on ARC, or getting involved in their events? Goto https://tufalabs.ai/
TOC:
[00:00:00] 0. Introduction / CentML ad, Tufa ad
1. AI Reasoning: From Language Models to Neurosymbolic Approaches
[00:02:27] 1.1 Defining Reasoning in AI
[00:09:51] 1.2 Limitations of Current Language Models
[00:17:22] 1.3 Neuro-symbolic Approaches and Program Synthesis
[00:24:59] 1.4 COPRA and In-Context Learning for Theorem Proving
[00:34:39] 1.5 Symbolic Regression and LLM-Guided Abstraction
2. AI in Mathematics: Theorem Proving and Concept Discovery
[00:43:37] 2.1 AI-Assisted Theorem Proving and Proof Verification
[01:01:37] 2.2 Symbolic Regression and Concept Discovery in Mathematics
[01:11:57] 2.3 Scaling and Modularizing Mathematical Proofs
[01:21:53] 2.4 COPRA: In-Context Learning for Formal Theorem-Proving
[01:28:22] 2.5 AI-driven theorem proving and mathematical discovery
3. Formal Methods and Challenges in AI Mathematics
[01:30:42] 3.1 Formal proofs, empirical predicates, and uncertainty in AI mathematics
[01:34:01] 3.2 Characteristics of good theoretical computer science research
[01:39:16] 3.3 LLMs in theorem generation and proving
[01:42:21] 3.4 Addressing contamination and concept learning in AI systems
REFS:
00:04:58 The Chinese Room Argument, https://plato.stanford.edu/entries/chinese-room/
00:11:42 Software 2.0, https://medium.com/@karpathy/software-2-0-a64152b37c35
00:11:57 Solving Olympiad Geometry Without Human Demonstrations, https://www.nature.com/articles/s41586-023-06747-5
00:13:26 Lean, https://lean-lang.org/
00:15:43 A General Reinforcement Learning Algorithm That Masters Chess, Shogi, and Go Through Self-Play, https://www.science.org/doi/10.1126/science.aar6404
00:19:24 DreamCoder (Ellis et al., PLDI 2021), https://arxiv.org/abs/2006.08381
00:24:37 The Lambda Calculus, https://plato.stanford.edu/entries/lambda-calculus/
00:26:43 Neural Sketch Learning for Conditional Program Generation, https://arxiv.org/pdf/1703.05698
00:28:08 Learning Differentiable Programs With Admissible Neural Heuristics, https://arxiv.org/abs/2007.12101
00:31:03 Symbolic Regression With a Learned Concept Library (Grayeli et al., NeurIPS 2024), https://arxiv.org/abs/2409.09359
00:41:30 Formal Verification of Parallel Programs, https://dl.acm.org/doi/10.1145/360248.360251
01:00:37 Training Compute-Optimal Large Language Models, https://arxiv.org/abs/2203.15556
01:18:19 Chain-of-Thought Prompting Elicits Reasoning in Large Language Models, https://arxiv.org/abs/2201.11903
01:18:42 Draft, Sketch, and Prove: Guiding Formal Theorem Provers With Informal Proofs, https://arxiv.org/abs/2210.12283
01:19:49 Learning Formal Mathematics From Intrinsic Motivation, https://arxiv.org/pdf/2407.00695
01:20:19 An In-Context Learning Agent for Formal Theorem-Proving (Thakur et al., CoLM 2024), https://arxiv.org/pdf/2310.04353
01:23:58 Learning to Prove Theorems via Interacting With Proof Assistants, https://arxiv.org/abs/1905.09381
01:39:58 An In-Context Learning Agent for Formal Theorem-Proving (Thakur et al., CoLM 2024), https://arxiv.org/pdf/2310.04353
01:42:24 Programmatically Interpretable Reinforcement Learning (Verma et al., ICML 2018), https://arxiv.org/abs/1804.02477
4.7
8383 ratings
Professor Swarat Chaudhuri from the University of Texas at Austin and visiting researcher at Google DeepMind discusses breakthroughs in AI reasoning, theorem proving, and mathematical discovery. Chaudhuri explains his groundbreaking work on COPRA (a GPT-based prover agent), shares insights on neurosymbolic approaches to AI.
Professor Swarat Chaudhuri:
https://www.cs.utexas.edu/~swarat/
SPONSOR MESSAGES:
CentML offers competitive pricing for GenAI model deployment, with flexible options to suit a wide range of models, from small to large-scale deployments.
https://centml.ai/pricing/
Tufa AI Labs is a brand new research lab in Zurich started by Benjamin Crouzier focussed on ARC and AGI, they just acquired MindsAI - the current winners of the ARC challenge. Are you interested in working on ARC, or getting involved in their events? Goto https://tufalabs.ai/
TOC:
[00:00:00] 0. Introduction / CentML ad, Tufa ad
1. AI Reasoning: From Language Models to Neurosymbolic Approaches
[00:02:27] 1.1 Defining Reasoning in AI
[00:09:51] 1.2 Limitations of Current Language Models
[00:17:22] 1.3 Neuro-symbolic Approaches and Program Synthesis
[00:24:59] 1.4 COPRA and In-Context Learning for Theorem Proving
[00:34:39] 1.5 Symbolic Regression and LLM-Guided Abstraction
2. AI in Mathematics: Theorem Proving and Concept Discovery
[00:43:37] 2.1 AI-Assisted Theorem Proving and Proof Verification
[01:01:37] 2.2 Symbolic Regression and Concept Discovery in Mathematics
[01:11:57] 2.3 Scaling and Modularizing Mathematical Proofs
[01:21:53] 2.4 COPRA: In-Context Learning for Formal Theorem-Proving
[01:28:22] 2.5 AI-driven theorem proving and mathematical discovery
3. Formal Methods and Challenges in AI Mathematics
[01:30:42] 3.1 Formal proofs, empirical predicates, and uncertainty in AI mathematics
[01:34:01] 3.2 Characteristics of good theoretical computer science research
[01:39:16] 3.3 LLMs in theorem generation and proving
[01:42:21] 3.4 Addressing contamination and concept learning in AI systems
REFS:
00:04:58 The Chinese Room Argument, https://plato.stanford.edu/entries/chinese-room/
00:11:42 Software 2.0, https://medium.com/@karpathy/software-2-0-a64152b37c35
00:11:57 Solving Olympiad Geometry Without Human Demonstrations, https://www.nature.com/articles/s41586-023-06747-5
00:13:26 Lean, https://lean-lang.org/
00:15:43 A General Reinforcement Learning Algorithm That Masters Chess, Shogi, and Go Through Self-Play, https://www.science.org/doi/10.1126/science.aar6404
00:19:24 DreamCoder (Ellis et al., PLDI 2021), https://arxiv.org/abs/2006.08381
00:24:37 The Lambda Calculus, https://plato.stanford.edu/entries/lambda-calculus/
00:26:43 Neural Sketch Learning for Conditional Program Generation, https://arxiv.org/pdf/1703.05698
00:28:08 Learning Differentiable Programs With Admissible Neural Heuristics, https://arxiv.org/abs/2007.12101
00:31:03 Symbolic Regression With a Learned Concept Library (Grayeli et al., NeurIPS 2024), https://arxiv.org/abs/2409.09359
00:41:30 Formal Verification of Parallel Programs, https://dl.acm.org/doi/10.1145/360248.360251
01:00:37 Training Compute-Optimal Large Language Models, https://arxiv.org/abs/2203.15556
01:18:19 Chain-of-Thought Prompting Elicits Reasoning in Large Language Models, https://arxiv.org/abs/2201.11903
01:18:42 Draft, Sketch, and Prove: Guiding Formal Theorem Provers With Informal Proofs, https://arxiv.org/abs/2210.12283
01:19:49 Learning Formal Mathematics From Intrinsic Motivation, https://arxiv.org/pdf/2407.00695
01:20:19 An In-Context Learning Agent for Formal Theorem-Proving (Thakur et al., CoLM 2024), https://arxiv.org/pdf/2310.04353
01:23:58 Learning to Prove Theorems via Interacting With Proof Assistants, https://arxiv.org/abs/1905.09381
01:39:58 An In-Context Learning Agent for Formal Theorem-Proving (Thakur et al., CoLM 2024), https://arxiv.org/pdf/2310.04353
01:42:24 Programmatically Interpretable Reinforcement Learning (Verma et al., ICML 2018), https://arxiv.org/abs/1804.02477
474 Listeners
427 Listeners
295 Listeners
322 Listeners
195 Listeners
189 Listeners
275 Listeners
319 Listeners
105 Listeners
193 Listeners
64 Listeners
65 Listeners
418 Listeners
29 Listeners
32 Listeners