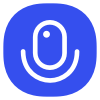
Sign up to save your podcasts
Or
Proudly sponsored by PyMC Labs, the Bayesian Consultancy. Book a call, or get in touch!
Our theme music is « Good Bayesian », by Baba Brinkman (feat MC Lars and Mega Ran). Check out his awesome work!
Visit our Patreon page to unlock exclusive Bayesian swag ;)
Takeaways:
Chapters:
00:00 Introduction to Jesse Krabowski and Time Series Analysis
04:33 Jesse's Journey into Bayesian Statistics
10:51 Exploring State Space Models
18:28 Understanding State Space Models and Their Components
40:39 Composability of State Space Models
48:36 Understanding Trends and Derivatives
52:35 The Importance of Seasonality in Time Series
56:41 Components of Time Series Analysis
01:00:46 Exogenous Regression in State Space Models
01:06:41 Impulse Response Functions and Causality
01:11:30 Why Kalman Filter Is So Powerful
01:24:28 Future Directions and Applications
Thank you to my Patrons for making this episode possible!
Yusuke Saito, Avi Bryant, Ero Carrera, Giuliano Cruz, Tim Gasser, James Wade, Tradd Salvo, William Benton, James Ahloy, Robin Taylor,, Chad Scherrer, Zwelithini Tunyiswa, Bertrand Wilden, James Thompson, Stephen Oates, Gian Luca Di Tanna, Jack Wells, Matthew Maldonado, Ian Costley, Ally Salim, Larry Gill, Ian Moran, Paul Oreto, Colin Caprani, Colin Carroll, Nathaniel Burbank, Michael Osthege, Rémi Louf, Clive Edelsten, Henri Wallen, Hugo Botha, Vinh Nguyen, Marcin Elantkowski, Adam C. Smith, Will Kurt, Andrew Moskowitz, Hector Munoz, Marco Gorelli, Simon Kessell, Bradley Rode, Patrick Kelley, Rick Anderson, Casper de Bruin, Philippe Labonde, Michael Hankin, Cameron Smith, Tomáš Frýda, Ryan Wesslen, Andreas Netti, Riley King, Yoshiyuki Hamajima, Sven De Maeyer, Michael DeCrescenzo, Fergal M, Mason Yahr, Naoya Kanai, Steven Rowland, Aubrey Clayton, Jeannine Sue, Omri Har Shemesh, Scott Anthony Robson, Robert Yolken, Or Duek, Pavel Dusek, Paul Cox, Andreas Kröpelin, Raphaël R, Nicolas Rode, Gabriel Stechschulte, Arkady, Kurt TeKolste, Gergely Juhasz, Marcus Nölke, Maggi Mackintosh, Grant Pezzolesi, Avram Aelony, Joshua Meehl, Javier Sabio, Kristian Higgins, Alex Jones, Gregorio Aguilar, Matt Rosinski, Bart Trudeau, Luis Fonseca, Dante Gates, Matt Niccolls, Maksim Kuznecov, Michael Thomas, Luke Gorrie, Cory Kiser, Julio, Edvin Saveljev, Frederick Ayala, Jeffrey Powell, Gal Kampel, Adan Romero, Will Geary, Blake Walters, Jonathan Morgan, Francesco Madrisotti, Ivy Huang, Gary Clarke, Robert Flannery, Rasmus Hindström, Stefan, Corey Abshire and Mike Loncaric.
Links from the show:
Transcript
This is an automatic transcript and may therefore contain errors. Please get in touch if you're willing to correct them.
4.7
6565 ratings
Proudly sponsored by PyMC Labs, the Bayesian Consultancy. Book a call, or get in touch!
Our theme music is « Good Bayesian », by Baba Brinkman (feat MC Lars and Mega Ran). Check out his awesome work!
Visit our Patreon page to unlock exclusive Bayesian swag ;)
Takeaways:
Chapters:
00:00 Introduction to Jesse Krabowski and Time Series Analysis
04:33 Jesse's Journey into Bayesian Statistics
10:51 Exploring State Space Models
18:28 Understanding State Space Models and Their Components
40:39 Composability of State Space Models
48:36 Understanding Trends and Derivatives
52:35 The Importance of Seasonality in Time Series
56:41 Components of Time Series Analysis
01:00:46 Exogenous Regression in State Space Models
01:06:41 Impulse Response Functions and Causality
01:11:30 Why Kalman Filter Is So Powerful
01:24:28 Future Directions and Applications
Thank you to my Patrons for making this episode possible!
Yusuke Saito, Avi Bryant, Ero Carrera, Giuliano Cruz, Tim Gasser, James Wade, Tradd Salvo, William Benton, James Ahloy, Robin Taylor,, Chad Scherrer, Zwelithini Tunyiswa, Bertrand Wilden, James Thompson, Stephen Oates, Gian Luca Di Tanna, Jack Wells, Matthew Maldonado, Ian Costley, Ally Salim, Larry Gill, Ian Moran, Paul Oreto, Colin Caprani, Colin Carroll, Nathaniel Burbank, Michael Osthege, Rémi Louf, Clive Edelsten, Henri Wallen, Hugo Botha, Vinh Nguyen, Marcin Elantkowski, Adam C. Smith, Will Kurt, Andrew Moskowitz, Hector Munoz, Marco Gorelli, Simon Kessell, Bradley Rode, Patrick Kelley, Rick Anderson, Casper de Bruin, Philippe Labonde, Michael Hankin, Cameron Smith, Tomáš Frýda, Ryan Wesslen, Andreas Netti, Riley King, Yoshiyuki Hamajima, Sven De Maeyer, Michael DeCrescenzo, Fergal M, Mason Yahr, Naoya Kanai, Steven Rowland, Aubrey Clayton, Jeannine Sue, Omri Har Shemesh, Scott Anthony Robson, Robert Yolken, Or Duek, Pavel Dusek, Paul Cox, Andreas Kröpelin, Raphaël R, Nicolas Rode, Gabriel Stechschulte, Arkady, Kurt TeKolste, Gergely Juhasz, Marcus Nölke, Maggi Mackintosh, Grant Pezzolesi, Avram Aelony, Joshua Meehl, Javier Sabio, Kristian Higgins, Alex Jones, Gregorio Aguilar, Matt Rosinski, Bart Trudeau, Luis Fonseca, Dante Gates, Matt Niccolls, Maksim Kuznecov, Michael Thomas, Luke Gorrie, Cory Kiser, Julio, Edvin Saveljev, Frederick Ayala, Jeffrey Powell, Gal Kampel, Adan Romero, Will Geary, Blake Walters, Jonathan Morgan, Francesco Madrisotti, Ivy Huang, Gary Clarke, Robert Flannery, Rasmus Hindström, Stefan, Corey Abshire and Mike Loncaric.
Links from the show:
Transcript
This is an automatic transcript and may therefore contain errors. Please get in touch if you're willing to correct them.
1,005 Listeners
474 Listeners
586 Listeners
629 Listeners
440 Listeners
296 Listeners
214 Listeners
322 Listeners
185 Listeners
268 Listeners
187 Listeners
204 Listeners
138 Listeners
90 Listeners
72 Listeners