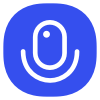
Sign up to save your podcasts
Or
Send us a text
Machine learning is not a new technology, but it started to revolutionize pathology relatively recently. The ideal combination of untapped, abundant pathology data necessary to leverage machine learning and the relevance of pathology applications has drawn scientists to this field and caused an artificial intelligence (AI) explosion.
Within just two years from 2018 to 2020 AI-based tissue image analysis went from “cutting edge technology” to “mainstream”. The deep learning explosion started with the Camelyon challenge which served as a proof of concept for the technology. The algorithms performing best in breast cancer metastasis detection in lymph nodes were all deep learning-based. This success combined with greater accessibility of whole slide scanning and recently accessibility of open-source deep learning frameworks led us to where we are today.
In computer vision, the task of the computer is to analyze images in a way that mimics how humans see.
This can be achieved in three main ways:
· Through rule-based systems by understanding the visual problem and writing rules such as intensity threshold definition, to solve it.
· By machine learning, where we still determine the features of interest and manipulate the images to enhance the signal we are looking for, but the rules for detecting our features of interest are learned by the computer. We use approaches such as:
· and through deep learning, where both the features of interest and the rules to extract those features are learned from the data.
This characteristic is at the core of AI power in tissue image analysis. Deep learning enables us to solve problems we could not solve before.
It was not possible to solve many of the pathology tasks with rule-based systems because it was not possible to define rules complex enough to achieve a good output. Now that there is no need for rules this barrier has been removed, and we can just give examples of what we are looking for instead.
Now instead of writing code, our task is to collect and curate data and generate examples of the structures we are looking for. Deep learning delivers image analysis to a much larger user base and empowers users who were not previously trained in image analysis to take advantage of this technology. This is a major breakthrough in this field. Shifting the main task in designing image analysis from writing code to curating data contributed to the greater involvement of pathologists who are uniquely trained in interpreting tissue and crucial to the process of assuring the quality of the data. However, they are not the only ones who can do this, which broadens the user base of this technology even more.
Even though AI is so powerful and accessible, there is still tremendous value in the classical image analysis approaches and even more so in combing the classical rule-based and machine learning approaches with deep learning. Visiopharm’s platform enables this combined approach by having an ecosystem of classical and AI-based approaches that can play together to best solve the problem. In this way, the problem picks the method and not the other way around, which is how it should be.
In the long-term, AI will help us get more insights into the pathobiology of diseases by helping in the interpr
Support the show
Become a Digital Pathology Trailblazer get the "Digital Pathology 101" FREE E-book and join us!
5
77 ratings
Send us a text
Machine learning is not a new technology, but it started to revolutionize pathology relatively recently. The ideal combination of untapped, abundant pathology data necessary to leverage machine learning and the relevance of pathology applications has drawn scientists to this field and caused an artificial intelligence (AI) explosion.
Within just two years from 2018 to 2020 AI-based tissue image analysis went from “cutting edge technology” to “mainstream”. The deep learning explosion started with the Camelyon challenge which served as a proof of concept for the technology. The algorithms performing best in breast cancer metastasis detection in lymph nodes were all deep learning-based. This success combined with greater accessibility of whole slide scanning and recently accessibility of open-source deep learning frameworks led us to where we are today.
In computer vision, the task of the computer is to analyze images in a way that mimics how humans see.
This can be achieved in three main ways:
· Through rule-based systems by understanding the visual problem and writing rules such as intensity threshold definition, to solve it.
· By machine learning, where we still determine the features of interest and manipulate the images to enhance the signal we are looking for, but the rules for detecting our features of interest are learned by the computer. We use approaches such as:
· and through deep learning, where both the features of interest and the rules to extract those features are learned from the data.
This characteristic is at the core of AI power in tissue image analysis. Deep learning enables us to solve problems we could not solve before.
It was not possible to solve many of the pathology tasks with rule-based systems because it was not possible to define rules complex enough to achieve a good output. Now that there is no need for rules this barrier has been removed, and we can just give examples of what we are looking for instead.
Now instead of writing code, our task is to collect and curate data and generate examples of the structures we are looking for. Deep learning delivers image analysis to a much larger user base and empowers users who were not previously trained in image analysis to take advantage of this technology. This is a major breakthrough in this field. Shifting the main task in designing image analysis from writing code to curating data contributed to the greater involvement of pathologists who are uniquely trained in interpreting tissue and crucial to the process of assuring the quality of the data. However, they are not the only ones who can do this, which broadens the user base of this technology even more.
Even though AI is so powerful and accessible, there is still tremendous value in the classical image analysis approaches and even more so in combing the classical rule-based and machine learning approaches with deep learning. Visiopharm’s platform enables this combined approach by having an ecosystem of classical and AI-based approaches that can play together to best solve the problem. In this way, the problem picks the method and not the other way around, which is how it should be.
In the long-term, AI will help us get more insights into the pathobiology of diseases by helping in the interpr
Support the show
Become a Digital Pathology Trailblazer get the "Digital Pathology 101" FREE E-book and join us!
6,115 Listeners
324 Listeners
493 Listeners
43,442 Listeners
111,785 Listeners
56,191 Listeners
122 Listeners
318 Listeners
147 Listeners
4 Listeners
28,304 Listeners
15,237 Listeners
611 Listeners
2,202 Listeners
485 Listeners