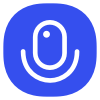
Sign up to save your podcasts
Or
Proudly sponsored by PyMC Labs, the Bayesian Consultancy. Book a call, or get in touch!
I’m sure you know at least one Bart. Maybe you’ve even used one — but you’re not proud of it, because you didn’t know what you were doing. Thankfully, in this episode, we’ll go to the roots of regression trees — oh yeah, that’s what BART stands for. What were you thinking about?
Our tree expert will be no one else than Sameer Deshpande. Sameer is an assistant professor of Statistics at the University of Wisconsin-Madison. Prior to that, he completed a postdoc at MIT and earned his Ph.D. in Statistics from UPenn.
On the methodological front, he is interested in Bayesian hierarchical modeling, regression trees, model selection, and causal inference. Much of his applied work is motivated by an interest in understanding the long-term health consequences of playing American-style tackle football. He also enjoys modeling sports data and was a finalist in the 2019 NFL Big Data Bowl.
Outside of Statistics, he enjoys cooking, making cocktails, and photography — sometimes doing all of those at the same time…
Our theme music is « Good Bayesian », by Baba Brinkman (feat MC Lars and Mega Ran). Check out his awesome work at https://bababrinkman.com/ !
Thank you to my Patrons for making this episode possible!
Yusuke Saito, Avi Bryant, Ero Carrera, Giuliano Cruz, Tim Gasser, James Wade, Tradd Salvo, William Benton, James Ahloy, Robin Taylor, Thomas Wiecki, Chad Scherrer, Nathaniel Neitzke, Zwelithini Tunyiswa, Bertrand Wilden, James Thompson, Stephen Oates, Gian Luca Di Tanna, Jack Wells, Matthew Maldonado, Ian Costley, Ally Salim, Larry Gill, Joshua Duncan, Ian Moran, Paul Oreto, Colin Caprani, Colin Carroll, Nathaniel Burbank, Michael Osthege, Rémi Louf, Clive Edelsten, Henri Wallen, Hugo Botha, Vinh Nguyen, Raul Maldonado, Marcin Elantkowski, Adam C. Smith, Will Kurt, Andrew Moskowitz, Hector Munoz, Marco Gorelli, Simon Kessell, Bradley Rode, Patrick Kelley, Rick Anderson, Casper de Bruin, Philippe Labonde, Michael Hankin, Cameron Smith, Tomáš Frýda, Ryan Wesslen, Andreas Netti, Riley King, Yoshiyuki Hamajima, Sven De Maeyer, Michael DeCrescenzo, Fergal M, Mason Yahr, Naoya Kanai, Steven Rowland, Aubrey Clayton, Jeannine Sue, Omri Har Shemesh, Scott Anthony Robson, David Haas, Robert Yolken, Or Duek, Pavel Dusek, Paul Cox, Trey Causey, Andreas Kröpelin, Raphaël R, Nicolas Rode, Gabriel Stechschulte, and Arkady.
Visit https://www.patreon.com/learnbayesstats to unlock exclusive Bayesian swag ;)
Links from the show:
Abstract
by Christoph Bamberg
In episode 80, Sameer Deshpande, assistant professor of Statistics at the University of Wisconsin-Madison is our guest.
He had a passion for math from a young age. And got into Bayesian statistics at university, teaching statistics now himself. We talk about the intricacies of teaching bayesian statistics, such as helping students accept that there are no objective answers.
Sameer’s current work focuses on Bayesian Additive Regression Trees (BARTs). He also works on prior specification, and numerous cool applied projects, for example on the effects of playing American football as an adolescent and its effects for later health
We primarily talk about BARTs as a way of approximating complex functions by using a collection of step functions. They work off the shelf pretty well and can be applied to various models such as survival models, linear models, and smooth models. BARTs are somewhat analogous to splines and can capture trajectories well over time. However, they are also a bit like a black box making them hard to interpret.
We further touch upon some of his work on practical problems, such as how cognitive processes change over time or models of baseball empires’ decision making.
Transcript
Please note that the following transcript was generated automatically and may therefore contain errors. Feel free to reach out if you're willing to correct them.
4.7
6262 ratings
Proudly sponsored by PyMC Labs, the Bayesian Consultancy. Book a call, or get in touch!
I’m sure you know at least one Bart. Maybe you’ve even used one — but you’re not proud of it, because you didn’t know what you were doing. Thankfully, in this episode, we’ll go to the roots of regression trees — oh yeah, that’s what BART stands for. What were you thinking about?
Our tree expert will be no one else than Sameer Deshpande. Sameer is an assistant professor of Statistics at the University of Wisconsin-Madison. Prior to that, he completed a postdoc at MIT and earned his Ph.D. in Statistics from UPenn.
On the methodological front, he is interested in Bayesian hierarchical modeling, regression trees, model selection, and causal inference. Much of his applied work is motivated by an interest in understanding the long-term health consequences of playing American-style tackle football. He also enjoys modeling sports data and was a finalist in the 2019 NFL Big Data Bowl.
Outside of Statistics, he enjoys cooking, making cocktails, and photography — sometimes doing all of those at the same time…
Our theme music is « Good Bayesian », by Baba Brinkman (feat MC Lars and Mega Ran). Check out his awesome work at https://bababrinkman.com/ !
Thank you to my Patrons for making this episode possible!
Yusuke Saito, Avi Bryant, Ero Carrera, Giuliano Cruz, Tim Gasser, James Wade, Tradd Salvo, William Benton, James Ahloy, Robin Taylor, Thomas Wiecki, Chad Scherrer, Nathaniel Neitzke, Zwelithini Tunyiswa, Bertrand Wilden, James Thompson, Stephen Oates, Gian Luca Di Tanna, Jack Wells, Matthew Maldonado, Ian Costley, Ally Salim, Larry Gill, Joshua Duncan, Ian Moran, Paul Oreto, Colin Caprani, Colin Carroll, Nathaniel Burbank, Michael Osthege, Rémi Louf, Clive Edelsten, Henri Wallen, Hugo Botha, Vinh Nguyen, Raul Maldonado, Marcin Elantkowski, Adam C. Smith, Will Kurt, Andrew Moskowitz, Hector Munoz, Marco Gorelli, Simon Kessell, Bradley Rode, Patrick Kelley, Rick Anderson, Casper de Bruin, Philippe Labonde, Michael Hankin, Cameron Smith, Tomáš Frýda, Ryan Wesslen, Andreas Netti, Riley King, Yoshiyuki Hamajima, Sven De Maeyer, Michael DeCrescenzo, Fergal M, Mason Yahr, Naoya Kanai, Steven Rowland, Aubrey Clayton, Jeannine Sue, Omri Har Shemesh, Scott Anthony Robson, David Haas, Robert Yolken, Or Duek, Pavel Dusek, Paul Cox, Trey Causey, Andreas Kröpelin, Raphaël R, Nicolas Rode, Gabriel Stechschulte, and Arkady.
Visit https://www.patreon.com/learnbayesstats to unlock exclusive Bayesian swag ;)
Links from the show:
Abstract
by Christoph Bamberg
In episode 80, Sameer Deshpande, assistant professor of Statistics at the University of Wisconsin-Madison is our guest.
He had a passion for math from a young age. And got into Bayesian statistics at university, teaching statistics now himself. We talk about the intricacies of teaching bayesian statistics, such as helping students accept that there are no objective answers.
Sameer’s current work focuses on Bayesian Additive Regression Trees (BARTs). He also works on prior specification, and numerous cool applied projects, for example on the effects of playing American football as an adolescent and its effects for later health
We primarily talk about BARTs as a way of approximating complex functions by using a collection of step functions. They work off the shelf pretty well and can be applied to various models such as survival models, linear models, and smooth models. BARTs are somewhat analogous to splines and can capture trajectories well over time. However, they are also a bit like a black box making them hard to interpret.
We further touch upon some of his work on practical problems, such as how cognitive processes change over time or models of baseball empires’ decision making.
Transcript
Please note that the following transcript was generated automatically and may therefore contain errors. Feel free to reach out if you're willing to correct them.
474 Listeners
585 Listeners
450 Listeners
429 Listeners
295 Listeners
212 Listeners
322 Listeners
142 Listeners
267 Listeners
445 Listeners
190 Listeners
279 Listeners
136 Listeners
64 Listeners
101 Listeners