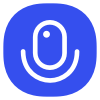
Sign up to save your podcasts
Or
One of the greatest features of this podcast, and my work in general, is that I keep getting surprised. Along the way, I keep learning, and I meet fascinating people, like Tarmo Jüristo.
Tarmo is hard to describe. These days, he’s heading an NGO called Salk, in the Baltic state called Estonia. Among other things, they are studying and forecasting elections, which is how we met and ended up collaborating with PyMC Labs, our Bayesian consultancy.
But Tarmo is much more than that. Born in 1971 in what was still the Soviet Union, he graduated in finance from Tartu University. He worked in finance and investment banking until the 2009 crisis, when he quit and started a doctorate in… cultural studies. He then went on to write for theater and TV, teaching literature, anthropology and philosophy. An avid world traveler, he also teaches kendo and Brazilian jiu-jitsu.
As you’ll hear in the episode, after lots of adventures, he established Salk, and they just used a Bayesian hierarchical model with post-stratification to forecast the results of the 2023 Estonian parliamentary elections and target the campaign efforts to specific demographics.
Oh, and let thing: Tarmo is a fan of the show — I told you he was a great guy ;)
Our theme music is « Good Bayesian », by Baba Brinkman (feat MC Lars and Mega Ran). Check out his awesome work at https://bababrinkman.com/ !
Thank you to my Patrons for making this episode possible!
Yusuke Saito, Avi Bryant, Ero Carrera, Giuliano Cruz, Tim Gasser, James Wade, Tradd Salvo, William Benton, James Ahloy, Robin Taylor,, Chad Scherrer, Nathaniel Neitzke, Zwelithini Tunyiswa, Bertrand Wilden, James Thompson, Stephen Oates, Gian Luca Di Tanna, Jack Wells, Matthew Maldonado, Ian Costley, Ally Salim, Larry Gill, Ian Moran, Paul Oreto, Colin Caprani, Colin Carroll, Nathaniel Burbank, Michael Osthege, Rémi Louf, Clive Edelsten, Henri Wallen, Hugo Botha, Vinh Nguyen, Raul Maldonado, Marcin Elantkowski, Adam C. Smith, Will Kurt, Andrew Moskowitz, Hector Munoz, Marco Gorelli, Simon Kessell, Bradley Rode, Patrick Kelley, Rick Anderson, Casper de Bruin, Philippe Labonde, Michael Hankin, Cameron Smith, Tomáš Frýda, Ryan Wesslen, Andreas Netti, Riley King, Yoshiyuki Hamajima, Sven De Maeyer, Michael DeCrescenzo, Fergal M, Mason Yahr, Naoya Kanai, Steven Rowland, Aubrey Clayton, Jeannine Sue, Omri Har Shemesh, Scott Anthony Robson, Robert Yolken, Or Duek, Pavel Dusek, Paul Cox, Trey Causey, Andreas Kröpelin, Raphaël R, Nicolas Rode, Gabriel Stechschulte, Arkady, Kurt TeKolste, Gergely Juhasz, Marcus Nölke, Maggi Mackintosh and Grant Pezzolesi.
Visit https://www.patreon.com/learnbayesstats to unlock exclusive Bayesian swag ;)
Links from the show:
Abstract
In episode 83 of the podcast Tarmo Jüristo is our guest. He recently received media attention for his electoral forecasting in the Estonian election and potential positive role in aiding liberal parties gain more votes than expected.
Tarmo explains to us how he used Bayesian models with his NGO SALK to forecast the election and how he leveraged these models to unify the different liberal parties that participated in the election. So, we get a firsthand view of how to use Bayesian modelling smartly.
Furthermore, we talk about when to use Bayesian models, difficulties in modelling survey data and how post-stratification can help.
He also explains how he, with the help of PyMC Labs, added Gaussian Processes to his models to better model the time-series structure of their survey data.
We close this episode by discussing the responsibility that comes with modelling data in politics.
Transcript
please note that the transcript was generated automatically and may therefore contain errors. Feel free to reach out if you're willing to correct them.
">Proudly sponsored by PyMC Labs, the Bayesian Consultancy. Book a call, or get in touch!
One of the greatest features of this podcast, and my work in general, is that I keep getting surprised. Along the way, I keep learning, and I meet fascinating people, like Tarmo Jüristo.
Tarmo is hard to describe. These days, he’s heading an NGO called Salk, in the Baltic state called Estonia. Among other things, they are studying and forecasting elections, which is how we met and ended up collaborating with PyMC Labs, our Bayesian consultancy.
But Tarmo is much more than that. Born in 1971 in what was still the Soviet Union, he graduated in finance from Tartu University. He worked in finance and investment banking until the 2009 crisis, when he quit and started a doctorate in… cultural studies. He then went on to write for theater and TV, teaching literature, anthropology and philosophy. An avid world traveler, he also teaches kendo and Brazilian jiu-jitsu.
As you’ll hear in the episode, after lots of adventures, he established Salk, and they just used a Bayesian hierarchical model with post-stratification to forecast the results of the 2023 Estonian parliamentary elections and target the campaign efforts to specific demographics.
Oh, and let thing: Tarmo is a fan of the show — I told you he was a great guy ;)
Our theme music is « Good Bayesian », by Baba Brinkman (feat MC Lars and Mega Ran). Check out his awesome work at https://bababrinkman.com/ !
Thank you to my Patrons for making this episode possible!
Yusuke Saito, Avi Bryant, Ero Carrera, Giuliano Cruz, Tim Gasser, James Wade, Tradd Salvo, William Benton, James Ahloy, Robin Taylor,, Chad Scherrer, Nathaniel Neitzke, Zwelithini Tunyiswa, Bertrand Wilden, James Thompson, Stephen Oates, Gian Luca Di Tanna, Jack Wells, Matthew Maldonado, Ian Costley, Ally Salim, Larry Gill, Ian Moran, Paul Oreto, Colin Caprani, Colin Carroll, Nathaniel Burbank, Michael Osthege, Rémi Louf, Clive Edelsten, Henri Wallen, Hugo Botha, Vinh Nguyen, Raul Maldonado, Marcin Elantkowski, Adam C. Smith, Will Kurt, Andrew Moskowitz, Hector Munoz, Marco Gorelli, Simon Kessell, Bradley Rode, Patrick Kelley, Rick Anderson, Casper de Bruin, Philippe Labonde, Michael Hankin, Cameron Smith, Tomáš Frýda, Ryan Wesslen, Andreas Netti, Riley King, Yoshiyuki Hamajima, Sven De Maeyer, Michael DeCrescenzo, Fergal M, Mason Yahr, Naoya Kanai, Steven Rowland, Aubrey Clayton, Jeannine Sue, Omri Har Shemesh, Scott Anthony Robson, Robert Yolken, Or Duek, Pavel Dusek, Paul Cox, Trey Causey, Andreas Kröpelin, Raphaël R, Nicolas Rode, Gabriel Stechschulte, Arkady, Kurt TeKolste, Gergely Juhasz, Marcus Nölke, Maggi Mackintosh and Grant Pezzolesi.
Visit https://www.patreon.com/learnbayesstats to unlock exclusive Bayesian swag ;)
Links from the show:
Abstract
by Christoph Bamberg
In episode 83 of the podcast Tarmo Jüristo is our guest. He recently received media attention for his electoral forecasting in the Estonian election and potential positive role in aiding liberal parties gain more votes than expected.
Tarmo explains to us how he used Bayesian models with his NGO SALK to forecast the election and how he leveraged these models to unify the different liberal parties that participated in the election. So, we get a firsthand view of how to use Bayesian modelling smartly.
Furthermore, we talk about when to use Bayesian models, difficulties in modelling survey data and how post-stratification can help.
He also explains how he, with the help of PyMC Labs, added Gaussian Processes to his models to better model the time-series structure of their survey data.
We close this episode by discussing the responsibility that comes with modelling data in politics.
Transcript
please note that the transcript was generated automatically and may therefore contain errors. Feel free to reach out if you're willing to correct them.
4.7
6262 ratings
Proudly sponsored by PyMC Labs, the Bayesian Consultancy. Book a call, or get in touch!
One of the greatest features of this podcast, and my work in general, is that I keep getting surprised. Along the way, I keep learning, and I meet fascinating people, like Tarmo Jüristo.
Tarmo is hard to describe. These days, he’s heading an NGO called Salk, in the Baltic state called Estonia. Among other things, they are studying and forecasting elections, which is how we met and ended up collaborating with PyMC Labs, our Bayesian consultancy.
But Tarmo is much more than that. Born in 1971 in what was still the Soviet Union, he graduated in finance from Tartu University. He worked in finance and investment banking until the 2009 crisis, when he quit and started a doctorate in… cultural studies. He then went on to write for theater and TV, teaching literature, anthropology and philosophy. An avid world traveler, he also teaches kendo and Brazilian jiu-jitsu.
As you’ll hear in the episode, after lots of adventures, he established Salk, and they just used a Bayesian hierarchical model with post-stratification to forecast the results of the 2023 Estonian parliamentary elections and target the campaign efforts to specific demographics.
Oh, and let thing: Tarmo is a fan of the show — I told you he was a great guy ;)
Our theme music is « Good Bayesian », by Baba Brinkman (feat MC Lars and Mega Ran). Check out his awesome work at https://bababrinkman.com/ !
Thank you to my Patrons for making this episode possible!
Yusuke Saito, Avi Bryant, Ero Carrera, Giuliano Cruz, Tim Gasser, James Wade, Tradd Salvo, William Benton, James Ahloy, Robin Taylor,, Chad Scherrer, Nathaniel Neitzke, Zwelithini Tunyiswa, Bertrand Wilden, James Thompson, Stephen Oates, Gian Luca Di Tanna, Jack Wells, Matthew Maldonado, Ian Costley, Ally Salim, Larry Gill, Ian Moran, Paul Oreto, Colin Caprani, Colin Carroll, Nathaniel Burbank, Michael Osthege, Rémi Louf, Clive Edelsten, Henri Wallen, Hugo Botha, Vinh Nguyen, Raul Maldonado, Marcin Elantkowski, Adam C. Smith, Will Kurt, Andrew Moskowitz, Hector Munoz, Marco Gorelli, Simon Kessell, Bradley Rode, Patrick Kelley, Rick Anderson, Casper de Bruin, Philippe Labonde, Michael Hankin, Cameron Smith, Tomáš Frýda, Ryan Wesslen, Andreas Netti, Riley King, Yoshiyuki Hamajima, Sven De Maeyer, Michael DeCrescenzo, Fergal M, Mason Yahr, Naoya Kanai, Steven Rowland, Aubrey Clayton, Jeannine Sue, Omri Har Shemesh, Scott Anthony Robson, Robert Yolken, Or Duek, Pavel Dusek, Paul Cox, Trey Causey, Andreas Kröpelin, Raphaël R, Nicolas Rode, Gabriel Stechschulte, Arkady, Kurt TeKolste, Gergely Juhasz, Marcus Nölke, Maggi Mackintosh and Grant Pezzolesi.
Visit https://www.patreon.com/learnbayesstats to unlock exclusive Bayesian swag ;)
Links from the show:
Abstract
by Christoph Bamberg
In episode 83 of the podcast Tarmo Jüristo is our guest. He recently received media attention for his electoral forecasting in the Estonian election and potential positive role in aiding liberal parties gain more votes than expected.
Tarmo explains to us how he used Bayesian models with his NGO SALK to forecast the election and how he leveraged these models to unify the different liberal parties that participated in the election. So, we get a firsthand view of how to use Bayesian modelling smartly.
Furthermore, we talk about when to use Bayesian models, difficulties in modelling survey data and how post-stratification can help.
He also explains how he, with the help of PyMC Labs, added Gaussian Processes to his models to better model the time-series structure of their survey data.
We close this episode by discussing the responsibility that comes with modelling data in politics.
Transcript
please note that the transcript was generated automatically and may therefore contain errors. Feel free to reach out if you're willing to correct them.
474 Listeners
584 Listeners
450 Listeners
429 Listeners
295 Listeners
212 Listeners
321 Listeners
143 Listeners
267 Listeners
445 Listeners
190 Listeners
279 Listeners
137 Listeners
64 Listeners
101 Listeners