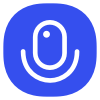
Sign up to save your podcasts
Or
Proudly sponsored by PyMC Labs, the Bayesian Consultancy. Book a call, or get in touch!
What’s the difference between MCMC and Variational Inference (VI)? Why is MCMC called an approximate method? When should we use VI instead of MCMC?
These are some of the captivating (and practical) questions we’ll tackle in this episode. I had the chance to interview Charles Margossian, a research fellow in computational mathematics at the Flatiron Institute, and a core developer of the Stan software.
Charles was born and raised in Paris, and then moved to the US to pursue a bachelor’s degree in physics at Yale university. After graduating, he worked for two years in biotech, and went on to do a PhD in statistics at Columbia University with someone named… Andrew Gelman — you may have heard of him.
Charles is also specialized in pharmacometrics and epidemiology, so we also talked about some practical applications of Bayesian methods and algorithms in these fascinating fields.
Oh, and Charles’ life doesn’t only revolve around computers: he practices ballroom dancing and pickup soccer, and used to do improvised musical comedy!
Our theme music is « Good Bayesian », by Baba Brinkman (feat MC Lars and Mega Ran). Check out his awesome work at https://bababrinkman.com/ !
Thank you to my Patrons for making this episode possible!
Yusuke Saito, Avi Bryant, Ero Carrera, Giuliano Cruz, Tim Gasser, James Wade, Tradd Salvo, William Benton, James Ahloy, Robin Taylor,, Chad Scherrer, Zwelithini Tunyiswa, Bertrand Wilden, James Thompson, Stephen Oates, Gian Luca Di Tanna, Jack Wells, Matthew Maldonado, Ian Costley, Ally Salim, Larry Gill, Ian Moran, Paul Oreto, Colin Caprani, Colin Carroll, Nathaniel Burbank, Michael Osthege, Rémi Louf, Clive Edelsten, Henri Wallen, Hugo Botha, Vinh Nguyen, Raul Maldonado, Marcin Elantkowski, Adam C. Smith, Will Kurt, Andrew Moskowitz, Hector Munoz, Marco Gorelli, Simon Kessell, Bradley Rode, Patrick Kelley, Rick Anderson, Casper de Bruin, Philippe Labonde, Michael Hankin, Cameron Smith, Tomáš Frýda, Ryan Wesslen, Andreas Netti, Riley King, Yoshiyuki Hamajima, Sven De Maeyer, Michael DeCrescenzo, Fergal M, Mason Yahr, Naoya Kanai, Steven Rowland, Aubrey Clayton, Jeannine Sue, Omri Har Shemesh, Scott Anthony Robson, Robert Yolken, Or Duek, Pavel Dusek, Paul Cox, Trey Causey, Andreas Kröpelin, Raphaël R, Nicolas Rode, Gabriel Stechschulte, Arkady, Kurt TeKolste, Gergely Juhasz, Marcus Nölke, Maggi Mackintosh, Grant Pezzolesi, Avram Aelony, Joshua Meehl, Javier Sabio, Kristian Higgins, Alex Jones, Gregorio Aguilar and Matt Rosinski.
Visit https://www.patreon.com/learnbayesstats to unlock exclusive Bayesian swag ;)
Links from the show:
Abstract
by Christoph Bamberg
In episode 90 we cover both methodological advances and their application, namely variational inference and MCMC sampling and their application in pharmacometrics.
And we have just the right guest for this topic - Charles Margossian! You might know Charles from his work on STAN, his workshop teaching or his work at his current position at the Flatiron Institute.
His main focus now is on two topics: variational inference and MCMC sampling. When is variational inference (or approximate Bayesian methods) appropriate? And when does it fail? Charles answers these questions convincingly, clearing up some discussion around this topic.
In his work on MCMC, he tries to answer some fundamental questions: How much computational power should we invest? When is MCMC sampling more appropriate than approximate Bayesian methods? The short answer: when you care about quantifying uncertainty. We even talk about what the R-hat measure means and how to improve on it with nested R-hats.
After covering these two topics, we move to his practical work: pharmacometrics. For example, he worked on modelling the speed of drugs dissolving in the body or the role of genetics in the workings of drugs.
Charles also contributes to making Bayesian methods more accessible for pharmacologists: He co-developed the Torsten library for Stan that facilitates Bayesian analysis with pharmacometric data.
We discuss the nature of pharmacometric data and how it is usually modelled with Ordinary Differential Equations.
In the end we briefly cover one practical example of pharmacometric modelling: the Covid-19 pandemic.
All in all, episode 90 is another detailed one, covering many state-of-the-art techniques and their application.
Transcript
This is an automatic transcript and may therefore contain errors. Please get in touch if you're willing to correct them.
4.7
6262 ratings
Proudly sponsored by PyMC Labs, the Bayesian Consultancy. Book a call, or get in touch!
What’s the difference between MCMC and Variational Inference (VI)? Why is MCMC called an approximate method? When should we use VI instead of MCMC?
These are some of the captivating (and practical) questions we’ll tackle in this episode. I had the chance to interview Charles Margossian, a research fellow in computational mathematics at the Flatiron Institute, and a core developer of the Stan software.
Charles was born and raised in Paris, and then moved to the US to pursue a bachelor’s degree in physics at Yale university. After graduating, he worked for two years in biotech, and went on to do a PhD in statistics at Columbia University with someone named… Andrew Gelman — you may have heard of him.
Charles is also specialized in pharmacometrics and epidemiology, so we also talked about some practical applications of Bayesian methods and algorithms in these fascinating fields.
Oh, and Charles’ life doesn’t only revolve around computers: he practices ballroom dancing and pickup soccer, and used to do improvised musical comedy!
Our theme music is « Good Bayesian », by Baba Brinkman (feat MC Lars and Mega Ran). Check out his awesome work at https://bababrinkman.com/ !
Thank you to my Patrons for making this episode possible!
Yusuke Saito, Avi Bryant, Ero Carrera, Giuliano Cruz, Tim Gasser, James Wade, Tradd Salvo, William Benton, James Ahloy, Robin Taylor,, Chad Scherrer, Zwelithini Tunyiswa, Bertrand Wilden, James Thompson, Stephen Oates, Gian Luca Di Tanna, Jack Wells, Matthew Maldonado, Ian Costley, Ally Salim, Larry Gill, Ian Moran, Paul Oreto, Colin Caprani, Colin Carroll, Nathaniel Burbank, Michael Osthege, Rémi Louf, Clive Edelsten, Henri Wallen, Hugo Botha, Vinh Nguyen, Raul Maldonado, Marcin Elantkowski, Adam C. Smith, Will Kurt, Andrew Moskowitz, Hector Munoz, Marco Gorelli, Simon Kessell, Bradley Rode, Patrick Kelley, Rick Anderson, Casper de Bruin, Philippe Labonde, Michael Hankin, Cameron Smith, Tomáš Frýda, Ryan Wesslen, Andreas Netti, Riley King, Yoshiyuki Hamajima, Sven De Maeyer, Michael DeCrescenzo, Fergal M, Mason Yahr, Naoya Kanai, Steven Rowland, Aubrey Clayton, Jeannine Sue, Omri Har Shemesh, Scott Anthony Robson, Robert Yolken, Or Duek, Pavel Dusek, Paul Cox, Trey Causey, Andreas Kröpelin, Raphaël R, Nicolas Rode, Gabriel Stechschulte, Arkady, Kurt TeKolste, Gergely Juhasz, Marcus Nölke, Maggi Mackintosh, Grant Pezzolesi, Avram Aelony, Joshua Meehl, Javier Sabio, Kristian Higgins, Alex Jones, Gregorio Aguilar and Matt Rosinski.
Visit https://www.patreon.com/learnbayesstats to unlock exclusive Bayesian swag ;)
Links from the show:
Abstract
by Christoph Bamberg
In episode 90 we cover both methodological advances and their application, namely variational inference and MCMC sampling and their application in pharmacometrics.
And we have just the right guest for this topic - Charles Margossian! You might know Charles from his work on STAN, his workshop teaching or his work at his current position at the Flatiron Institute.
His main focus now is on two topics: variational inference and MCMC sampling. When is variational inference (or approximate Bayesian methods) appropriate? And when does it fail? Charles answers these questions convincingly, clearing up some discussion around this topic.
In his work on MCMC, he tries to answer some fundamental questions: How much computational power should we invest? When is MCMC sampling more appropriate than approximate Bayesian methods? The short answer: when you care about quantifying uncertainty. We even talk about what the R-hat measure means and how to improve on it with nested R-hats.
After covering these two topics, we move to his practical work: pharmacometrics. For example, he worked on modelling the speed of drugs dissolving in the body or the role of genetics in the workings of drugs.
Charles also contributes to making Bayesian methods more accessible for pharmacologists: He co-developed the Torsten library for Stan that facilitates Bayesian analysis with pharmacometric data.
We discuss the nature of pharmacometric data and how it is usually modelled with Ordinary Differential Equations.
In the end we briefly cover one practical example of pharmacometric modelling: the Covid-19 pandemic.
All in all, episode 90 is another detailed one, covering many state-of-the-art techniques and their application.
Transcript
This is an automatic transcript and may therefore contain errors. Please get in touch if you're willing to correct them.
474 Listeners
585 Listeners
450 Listeners
429 Listeners
295 Listeners
212 Listeners
322 Listeners
142 Listeners
267 Listeners
445 Listeners
190 Listeners
279 Listeners
136 Listeners
64 Listeners
101 Listeners