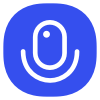
Sign up to save your podcasts
Or
In this episode, co-hosts Calum Chace and David Wood continue their review of progress in AI, taking up the story at the 2012 "Big Bang".
00.05: Introduction: exponential impact, big bangs, jolts, and jerks
00.45: What enabled the Big Bang
01.25: Moore's Law
02.05: Moore's Law has always evolved since its inception in 1965
03.08: Intel's tick tock becomes tic tac toe
03.49: GPUs - Graphic Processing Units
04.29: TPUs - Tensor Processing Units
04.46: Moore's Law is not dead or dying
05.10: 3D chips
05.32: Memristors
05.54: Neuromorphic chips
06.48: Quantum computing
08.18: The astonishing effect of exponential growth
09.08: We have seen this effect in computing already. The cost of an iPhone in the 1950s.
09.42: Exponential growth can't continue forever, but Moore's Law hasn't reached any theoretical limits
10.33: Reasons why Moore's Law might end: too small, too expensive, not worthwhile
11.20: Counter-arguments
12.01: "Plenty more room at the bottom"
12.56: Software and algorithms can help keep Moore's Law going
14.15: Using AI to improve chip design
14.40: Data is critical
15.00: ImageNet, Fei Fei Lee, Amazon Turk
16.10: AIs labelling data
16.35: The Big Bang
17.00: Jürgen Schmidhuber challenges the narrative
17.41: The Big Bang enabled AI to make money
18.24: 2015 and the Great Robot Freak-Out
18.43: Progress in many domains, especially natural language processing
19.44: Machine Learning and Deep Learning
20.25: Boiling the ocean vs the scientific method's hypothesis-driven approach
21.15: Deep Learning: levels
21.57: How Deep Learning systems recognise faces
22.48: Supervised, Unsupervised, and Reinforcement Learning
24.00: Variants, including Deep Reinforcement Learning and Self-Supervised Learning
24.30: Yann LeCun's camera metaphor for Deep Learning
26.05: Lack of transparency is a concern
27.45: Explainable AI. Is it achievable?
29.00: Other AI problems
29.17: Has another Big Bang taken place? Large Language Models like GPT-3
30.08: Few-shot learning and transfer learning
30.40: Escaping Uncanny Valley
31.50: Gato and partially general AI
Music: Spike Protein, by Koi Discovery, available under CC0 1.0 Public Domain Declaration
For more about the podcast hosts, see https://calumchace.com/ and https://dw2blog.com/
An Acxiom podcast where we discuss marketing made better, bringing you real...
Listen on: Apple Podcasts Spotify
4.7
99 ratings
In this episode, co-hosts Calum Chace and David Wood continue their review of progress in AI, taking up the story at the 2012 "Big Bang".
00.05: Introduction: exponential impact, big bangs, jolts, and jerks
00.45: What enabled the Big Bang
01.25: Moore's Law
02.05: Moore's Law has always evolved since its inception in 1965
03.08: Intel's tick tock becomes tic tac toe
03.49: GPUs - Graphic Processing Units
04.29: TPUs - Tensor Processing Units
04.46: Moore's Law is not dead or dying
05.10: 3D chips
05.32: Memristors
05.54: Neuromorphic chips
06.48: Quantum computing
08.18: The astonishing effect of exponential growth
09.08: We have seen this effect in computing already. The cost of an iPhone in the 1950s.
09.42: Exponential growth can't continue forever, but Moore's Law hasn't reached any theoretical limits
10.33: Reasons why Moore's Law might end: too small, too expensive, not worthwhile
11.20: Counter-arguments
12.01: "Plenty more room at the bottom"
12.56: Software and algorithms can help keep Moore's Law going
14.15: Using AI to improve chip design
14.40: Data is critical
15.00: ImageNet, Fei Fei Lee, Amazon Turk
16.10: AIs labelling data
16.35: The Big Bang
17.00: Jürgen Schmidhuber challenges the narrative
17.41: The Big Bang enabled AI to make money
18.24: 2015 and the Great Robot Freak-Out
18.43: Progress in many domains, especially natural language processing
19.44: Machine Learning and Deep Learning
20.25: Boiling the ocean vs the scientific method's hypothesis-driven approach
21.15: Deep Learning: levels
21.57: How Deep Learning systems recognise faces
22.48: Supervised, Unsupervised, and Reinforcement Learning
24.00: Variants, including Deep Reinforcement Learning and Self-Supervised Learning
24.30: Yann LeCun's camera metaphor for Deep Learning
26.05: Lack of transparency is a concern
27.45: Explainable AI. Is it achievable?
29.00: Other AI problems
29.17: Has another Big Bang taken place? Large Language Models like GPT-3
30.08: Few-shot learning and transfer learning
30.40: Escaping Uncanny Valley
31.50: Gato and partially general AI
Music: Spike Protein, by Koi Discovery, available under CC0 1.0 Public Domain Declaration
For more about the podcast hosts, see https://calumchace.com/ and https://dw2blog.com/
An Acxiom podcast where we discuss marketing made better, bringing you real...
Listen on: Apple Podcasts Spotify
5,389 Listeners
401 Listeners
26,462 Listeners
1,032 Listeners
10,694 Listeners
613 Listeners
198 Listeners
407 Listeners
442 Listeners
3,276 Listeners
981 Listeners
477 Listeners
987 Listeners
220 Listeners
2,285 Listeners