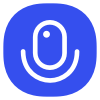
Sign up to save your podcasts
Or
Ben Taylor is the Chief AI Evangelist at DataRobot. He's a veteran thought leader on AI with over 16 years of experience. He was at Intel and Micron working on photolithography, process control, and yield prediction groups. He joined an AI hedge fund as an expert in high performance computing and AI, where he built models using a cluster of 600 GPUs. He then joined a young HR startup called HireVue, where he built out their data science group, filed 7 patents, and helped to launch their AI insights product using video/audio from candidate interviews. In 2017, Taylor co-founded Zeff.ai to pursue deep learning for image, audio, video, and text for the enterprise.
In this episode, we cover a range of topics including:
- Automating network design with genetic programming and deep learning
- Key learnings as HireVue's first data scientist
- Why he doesn't like Tensorflow
- Metaheuristics
- Key learnings as the cofounder of Zeff.ai
- What does it take to go from 0-to-1 when building an ML product
4.9
88 ratings
Ben Taylor is the Chief AI Evangelist at DataRobot. He's a veteran thought leader on AI with over 16 years of experience. He was at Intel and Micron working on photolithography, process control, and yield prediction groups. He joined an AI hedge fund as an expert in high performance computing and AI, where he built models using a cluster of 600 GPUs. He then joined a young HR startup called HireVue, where he built out their data science group, filed 7 patents, and helped to launch their AI insights product using video/audio from candidate interviews. In 2017, Taylor co-founded Zeff.ai to pursue deep learning for image, audio, video, and text for the enterprise.
In this episode, we cover a range of topics including:
- Automating network design with genetic programming and deep learning
- Key learnings as HireVue's first data scientist
- Why he doesn't like Tensorflow
- Metaheuristics
- Key learnings as the cofounder of Zeff.ai
- What does it take to go from 0-to-1 when building an ML product
1,000 Listeners
508 Listeners
2,293 Listeners
296 Listeners
387 Listeners
731 Listeners
282 Listeners
8,768 Listeners
354 Listeners
128 Listeners
65 Listeners
428 Listeners
432 Listeners
914 Listeners
37 Listeners