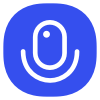
Sign up to save your podcasts
Or
Hey everyone! Thank you so much for watching the 114th episode of the Weaviate Podcast featuring Amanpreet Singh, Co-Founder and CTO of Contextual AI! Contextual AI is at the forefront of production-grade RAG agents! I learned so much from this conversation! We began by discussing the vision of RAG 2.0, jointly optimizing generative and retrieval models! This then lead us to discuss Agentic RAG and how the RAG 2.0 roadmap is evolving with emerging perspectives on tool use. Amanpreet continues to further motivate the importance of continual learning of the model and the prompt / few-shot examples -- discussing the limits of prompt engineering. Personally I have to admit I think I have been a bit too bullish on only tuning instructions / examples, Amanpreet made an excellent case for updating the weights of the models as well -- citing issues such as parametric knowledge conflicts, and later on discussing how Mechanistic Interpretability is used to audit models and their updates in enterprise settings. We then discussed Contextual AI's LMUnit for evaluating these systems. This then lead us into my favorite part of the podcast, a deep dive into RL algorithms for LLMs. I highly recommend checking out the links below to learn more about Contextual's innovations on APO and KTO! We then discuss the importance of domain specific data, Mechanistic Interpretability, return to another question on RAG 2.0, and conclude with Amanpreet's most exciting future directions for AI! I hope you enjoy the podcast!
4
44 ratings
Hey everyone! Thank you so much for watching the 114th episode of the Weaviate Podcast featuring Amanpreet Singh, Co-Founder and CTO of Contextual AI! Contextual AI is at the forefront of production-grade RAG agents! I learned so much from this conversation! We began by discussing the vision of RAG 2.0, jointly optimizing generative and retrieval models! This then lead us to discuss Agentic RAG and how the RAG 2.0 roadmap is evolving with emerging perspectives on tool use. Amanpreet continues to further motivate the importance of continual learning of the model and the prompt / few-shot examples -- discussing the limits of prompt engineering. Personally I have to admit I think I have been a bit too bullish on only tuning instructions / examples, Amanpreet made an excellent case for updating the weights of the models as well -- citing issues such as parametric knowledge conflicts, and later on discussing how Mechanistic Interpretability is used to audit models and their updates in enterprise settings. We then discussed Contextual AI's LMUnit for evaluating these systems. This then lead us into my favorite part of the podcast, a deep dive into RL algorithms for LLMs. I highly recommend checking out the links below to learn more about Contextual's innovations on APO and KTO! We then discuss the importance of domain specific data, Mechanistic Interpretability, return to another question on RAG 2.0, and conclude with Amanpreet's most exciting future directions for AI! I hope you enjoy the podcast!
1,008 Listeners
475 Listeners
525 Listeners
439 Listeners
295 Listeners
214 Listeners
2,616 Listeners
271 Listeners
8,385 Listeners
92 Listeners
315 Listeners
106 Listeners
70 Listeners
397 Listeners