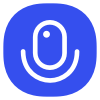
Sign up to save your podcasts
Or
Thank you so much for watching the 43rd episode of the Weaviate Podcast with Roman Grebennikov and Vesvolod Goloviznin from Metarank, as well as Erika Cardenas from Weaviate! This podcast is a masterclass on Ranking models, additionally touching on the connection between Search and Recommendation. Learning-to-rank is an exciting idea where we use models that produce more fine-grained relevance scores than the offline indexing techniques of vector search and bm25, however with the tradeoff of the speed of these inferences. Romand and Vsevolod touched on another extremely interesting part of these ranking models which is the estimation of features such as Click-through-Rates and how they use streaming technology to do this. I learned so much from this podcast about the directions in ranking, I hope you enjoy it as well! As always, we are more than happy to answer any questions or discuss any ideas with you!
4
44 ratings
Thank you so much for watching the 43rd episode of the Weaviate Podcast with Roman Grebennikov and Vesvolod Goloviznin from Metarank, as well as Erika Cardenas from Weaviate! This podcast is a masterclass on Ranking models, additionally touching on the connection between Search and Recommendation. Learning-to-rank is an exciting idea where we use models that produce more fine-grained relevance scores than the offline indexing techniques of vector search and bm25, however with the tradeoff of the speed of these inferences. Romand and Vsevolod touched on another extremely interesting part of these ranking models which is the estimation of features such as Click-through-Rates and how they use streaming technology to do this. I learned so much from this podcast about the directions in ranking, I hope you enjoy it as well! As always, we are more than happy to answer any questions or discuss any ideas with you!
1,270 Listeners
31,896 Listeners
507 Listeners
43,363 Listeners
244 Listeners
440 Listeners
111,077 Listeners
207 Listeners
188 Listeners
8,756 Listeners
129 Listeners
39 Listeners
72 Listeners
10 Listeners
33 Listeners