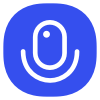
Sign up to save your podcasts
Or
Today we’re joined by Dan Fu, a PhD student at Stanford University. In our conversation with Dan, we discuss the limitations of state space models in language modeling and the search for alternative building blocks that can help increase context length without being computationally infeasible. Dan walks us through the H3 architecture and Flash Attention technique, which can reduce the memory footprint of a model and make it feasible to fine-tune. We also explore his work on improving language models using synthetic languages, the issue of long sequence length affecting both training and inference in models, and the hope for finding something sub-quadratic that can perform language processing more effectively than the brute force approach of attention.
The complete show notes for this episode can be found at https://twimlai.com/go/630
4.7
412412 ratings
Today we’re joined by Dan Fu, a PhD student at Stanford University. In our conversation with Dan, we discuss the limitations of state space models in language modeling and the search for alternative building blocks that can help increase context length without being computationally infeasible. Dan walks us through the H3 architecture and Flash Attention technique, which can reduce the memory footprint of a model and make it feasible to fine-tune. We also explore his work on improving language models using synthetic languages, the issue of long sequence length affecting both training and inference in models, and the hope for finding something sub-quadratic that can perform language processing more effectively than the brute force approach of attention.
The complete show notes for this episode can be found at https://twimlai.com/go/630
161 Listeners
470 Listeners
295 Listeners
324 Listeners
143 Listeners
190 Listeners
280 Listeners
88 Listeners
101 Listeners
125 Listeners
190 Listeners
63 Listeners
423 Listeners
29 Listeners
37 Listeners