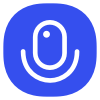
Sign up to save your podcasts
Or
Today, we're joined by Albert Gu, assistant professor at Carnegie Mellon University, to discuss his research on post-transformer architectures for multi-modal foundation models, with a focus on state-space models in general and Albert’s recent Mamba and Mamba-2 papers in particular. We dig into the efficiency of the attention mechanism and its limitations in handling high-resolution perceptual modalities, and the strengths and weaknesses of transformer architectures relative to alternatives for various tasks. We dig into the role of tokenization and patching in transformer pipelines, emphasizing how abstraction and semantic relationships between tokens underpin the model's effectiveness, and explore how this relates to the debate between handcrafted pipelines versus end-to-end architectures in machine learning. Additionally, we touch on the evolving landscape of hybrid models which incorporate elements of attention and state, the significance of state update mechanisms in model adaptability and learning efficiency, and the contribution and adoption of state-space models like Mamba and Mamba-2 in academia and industry. Lastly, Albert shares his vision for advancing foundation models across diverse modalities and applications.
The complete show notes for this episode can be found at https://twimlai.com/go/693.
4.7
412412 ratings
Today, we're joined by Albert Gu, assistant professor at Carnegie Mellon University, to discuss his research on post-transformer architectures for multi-modal foundation models, with a focus on state-space models in general and Albert’s recent Mamba and Mamba-2 papers in particular. We dig into the efficiency of the attention mechanism and its limitations in handling high-resolution perceptual modalities, and the strengths and weaknesses of transformer architectures relative to alternatives for various tasks. We dig into the role of tokenization and patching in transformer pipelines, emphasizing how abstraction and semantic relationships between tokens underpin the model's effectiveness, and explore how this relates to the debate between handcrafted pipelines versus end-to-end architectures in machine learning. Additionally, we touch on the evolving landscape of hybrid models which incorporate elements of attention and state, the significance of state update mechanisms in model adaptability and learning efficiency, and the contribution and adoption of state-space models like Mamba and Mamba-2 in academia and industry. Lastly, Albert shares his vision for advancing foundation models across diverse modalities and applications.
The complete show notes for this episode can be found at https://twimlai.com/go/693.
158 Listeners
475 Listeners
295 Listeners
312 Listeners
149 Listeners
196 Listeners
271 Listeners
92 Listeners
320 Listeners
106 Listeners
178 Listeners
70 Listeners
397 Listeners
26 Listeners
31 Listeners