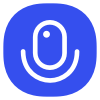
Sign up to save your podcasts
Or
This week we talk about stainless steel, DARPA, and GNoME.
We also discuss ceramics, DeepMind, and self-driving labs.
Recommended Book: Drunk On All Your Strange New Words by Eddie Robson
Transcript
In a recent episode, I talked a bit about the bronze and copper ages, and how reaching the level of technological know-how so that it's possible to heat metals so you can blend them with other metals, forge them into useful things, and generally work with them in a more fundamental way than is possible if you're simply chipping away at them, bending them with brute strength, and so on, grants you all sorts of additional powers that those cruder methods do not offer.
Copper's a pretty basic material to work with, as metals go, in part because of its elemental properties, and in part because it appears in nature, on Earth, in its pure form, so it's not something our ancestors would have had to imagine from whole cloth—they could see it, work with it, and thus, had a pretty good sense of what it was and what it was capable of.
Bronze, an alloy of copper—with some amount of tin mixed into the copper to make it more resilient and strong, and thus, useful for many things—was different in that it's not natural and doesn't occur unless we synthetically produce it.
Iron is similar to copper in that it's natural, though it's also a lot stronger and thus harder to work with, lacking the metallurgical capacity to melt it down and reshape it in a liquified form, and steel is in this way a bit like bronze in that it's an alloy of iron—iron mixed with carbon—and variations on the theme, like stainless steels, have some amount of chromium blended in with the iron and carbon, alongside nickel, in some cases, which makes it even more complex, and thus essentially impossible to imagine if you're limited to what nature provides you, in terms of practicality, and thus, often at least, your conception of materials-related possibilities.
So part of the challenge in attaining mastery over difference materials, including but not limited to metals, is discovering them and having access to the requisite natural resources, like iron and copper, in the first place, but then also, over time, learning that you can manipulate them in various ways, and then over time—often long, long stretches of time, generationally long periods of time in some cases—refining those methods of manipulation until it's possible to do so economically, but also, typically, at some kind of productive scale: allowing you to make enough of the material so you can churn out, for instance, armor and swords made out of it, or if we're talking about ceramic goods, stuff made of clay and silica and carbon, among other substances, scaling-up the process so you can produce more jugs and pots and urns, more food-preservation technologies and clay tablets for writing and bricks for building homes and other structures; and that's alongside the parallel process of simply learning how to capably work with these materials, once a sufficient volume of them becomes available.
So while metal and clay are different sorts of substances, they're both materials that we use to make objects—we take basic, earth-derived stuff and reshape it into things that are useful to us in some way, whether that means as a weapon or means of manufacturing things, or as clothing, homes, or objects of beauty—artworks and such.
Materials science is a field focused on the many facets of these types of resources, with some practitioners working with existing materials in order to better understand them, others sussing out various means of scaling-up production or iterating upon existing modes of production to make them more economical or sustainable, while still others aim to produce new materials of this kind: in some cases discovering existing-but-rare new materials, in the sense that we haven't discovered them, at least in the scientific sense, before, but often production, in this context, means combining different elements or other raw materials to create new materials.
Just like our ancestors figured out how to make stronger, longer-lasting ceramic pots and how to make stainless steal out of iron alloyed with other substances, the contemporary version of that field often means working in laboratories and manufacturing hubs to investigate the blending-potential of various materials, and to then refine successful blends to see if the resulting whatever might have utility that can be exploited for some kind of productive purpose.
What I'd like to talk about today is materials science, and how new innovations in the AI realm could push this field into an entirely new, and much faster-moving, paradigm.
—
As I mentioned in the intro, we've been doing what you might call materials science research and development since our earliest days of civilizational evolution, and almost certainly for quite a long while before that, too, because our deep, deep ancestors were all about making clever use of their environments and the materials in those environments, to get a leg-up over their competition.
That said, modern materials science arose out of earlier, differentiated fields like metallurgy and ceramics engineering classes and laboratories, some of these educational and commercial hubs slammed together into unified, materials science departments in the 1960s when the US Advanced Research Projects Agency—the precursor to the Defense Advanced Research Projects Agency, or DARPA—started throwing money at universities with laboratories that seemed capable of helping the US economy, and by association the US military, gain broad-scale advantages over their international competition, by approaching materials research not just from the 30,000-foot, macro-scale view that pretty much every such department had approached such things from until this point, but also the micro-scale, atomic-level perspective: something more fields were beginning to attempt in the wake of WWII and the increasingly common realization that we've been missing out on a lot, not looking at things from the atomic level, up till that point, and that by leveraging advanced understandings about how these substances work from other fields, like physics, we could probably speed-up our development of new incredibly useful, omni-versatile materials, like steel or aluminum, dramatically.
This would allow us to start our research with assumptions based on molecular and atomic science, rather than empirical, observational, comparably quite slow approaches, and that meant rather than waiting to observe and measure something interesting that happened, usually by doing a lot of fiddling around and hoping for good luck, over and over, day after day, we could instead very intentionally start cycling through all the potential blends that these other scientific understandings have told us are both possible and might be useful or interesting for various reasons.
In the decades since, materials science has expanded still-further, encompassing new and ever-smaller scales, and new material types, like polymers—plastics, basically—that weren't really a thing when the unified field first, itself, became a thing.
The impact this reorganization and refocus has had on the development of new materials cannot be overstated: among other things, innovations in this space has led to the development of artificial skin for burn victims, metal composites that have worked their way into all kinds of consumer products, making them more durable and lightweight, the production of medical hardware capable of performing magnetic resonance imaging and ultrasounds, the materials required to produce microchips of ever-smaller sizes, but with ever-denser capacities, nanotechnologies that have allowed for the shrinking of all sorts of components and devices, and the materials that have made the rapidly increasing efficiencies of solar panels possible, alongside the materials used in wind turbine blades and batteries with ever-embiggening capacities, safety features, and durabilities.
The modern world, in essence, all modern technologies, and especially all digital goods, but also everything made out of any kind of metal or plastic that isn't raw iron or copper, both of which are increasingly rare in consumer goods, at least, was enabled by the field of materials science; lacking that mid-20th century development, it's a fair bet we would have been held back in pretty much every other scientific field, and thus, technological development, as well.
That ubiquity and importance is part of why a recent announcement by Google's DeepMind division—an artificial intelligence lab under the larger company's brand-umbrella—has been getting so much attention.
DeepMind has become well-known for its upending of chess, the game of Go, and more recently for creating a protein structure database that contains all its predictions for the 3D structures of folded proteins—showing how more than 200 million proteins will likely look based on their amino acid sequences, alone, solving what has long been called the "protein folding problem," which I spoke about at greater length in a previous episode, by the way.
So we've got a database full of protein ingredients, amino acids, for all the proteins we've ever discovered, but just having those ingredients doesn't tell us what the finished proteins will look like in three-dimensions, once they've been built, because they fold up into a final shape after construction.
Figuring out how finished, folded proteins made up of those ingredients we knew about, how they would actually look in real-life, has thus been a time-consuming, ponderous and expensive effort—all of science, our entire human civilization-wide scientific effort, was able to demonstrate the final, folded structures of something like 170,000 of the more than 200 million proteins we knew about, up till the early 2020s.
That changed with DeepMind's AlphaFold program, which—using an AI technique called deep learning—was able to predict, imperfectly, but with enough accuracy to successfully predict single-mutation effects (what will happen if a protein has a single change to one of its amino acids, and how that will impact the final shape of the folder protein) all of those known proteins in our existing database.
So predictions that are usable for many use-cases, and at what's been called a borderline miraculous or magical scale, applying this prediction model to every single protein we know about, as a species, at this point.
That same lab has now applied a similar AI system to predicting and simulating how various materials will work together, if blended, and how their fusion, the product of that blending, will behave; what properties it will have.
The company announced that they've developed a new deep learning system optimized for this purpose, called Graph Networks for Materials Exploration, or GNoME, and the initial outcome of running this tool was the discovery of about 2.2 million new crystalline structures, about 380,000 of which are stable enough to warrant further materials science investigation.
Using current methods and extrapolating on the research currently being done and funding currently available to researchers in this space around the world, it's estimated that around 736 of these 380,000 new potential materials have already been discovered by researchers in experimental settings, and that this stockpile is equivalent to about 800 years'-worth of knowledge based on current levels of investment and output.
So it would take about 800 years, at current levels of research in this space, to discover this many new potentially useful materials.
All of which is wonderful, as—like with the folded protein predictions provided by AlphaFold—this new GNoME model gives materials scientists some focused areas to be looking at, making every experiment more likely to provide us with useable outcomes, rather than the shot-in-the-dark approach that's more common when looking into unfamiliar blends of materials.
Many of these 380,000 potential new structures will likely be not useful for today's purposes, then, but this type of research rigs the dice so that each investigation is relatively more likely to yield something really valuable, which could prove to be hugely beneficial, especially since that catalog of potentially useful structures, like the protein fold catalog, has been published and made available to whomever wants it, for free.
That's still a lot of work to do, of course, churning through all these potentially useful materials, which is why another development in this space—what's sometimes called self-driving labs—is also notable and potentially vital for the more-rapid development of materials science.
Self-driving labs are basically lab spaces optimized for robotics that allow non-human, robot arms and other hardware, to perform the requisite, and often slogging, ponderous, tedious work of basic materials science experimentation, safely and continuously, around the clock.
So just as you might automate a fast-food restaurant by telling some software what ingredients to combine and how to process them, in order to make a burger or some fries, keeping tabs on the temperature of everything and what's been mixed with what along the way, using specialized, automated equipment, you can also tell some software which materials to combine, and how, and have it keep track of everything's properties throughout the process using an array of sensors, and then some robot arms perhaps, or maybe just a big box with pipes and the ability to move stuff from here to there when prudent, will combine a slew of varied substances from a catalog of options, and then keep tabs on the resulting materials, tucking away examples for further, human exploration and confirmation if they're auto-tagged as being interesting for the sorts of properties we want to see, but otherwise maybe just categorizing them according to their properties, adding to the body of knowledge we already have for such things, and giving us a sort of materials reference library that we can tap into when we need a specific material with specific attributes, in the future.
What this potentially does, then, is robotically automate the checking of the AI-generated catalog of potentially useful materials.
The degree to which this could change the field cannot be overstated, as while that earlier, 1960s-era formalization of the field, combining earlier realms of inquiry was a big deal, changing everything, this next step could do the same, replacing humans—who are in many cases doing systematic, tedious work—with sleepless, emotionless, unkillable robots working from software-generated possibilities in order to provide us with a new menu of materials we might use, moving forward.
This sort of development is especially important, arguably, because of all these new possibilities we now have available to try out: the number of possible combinations grows incredibly rapidly as the number of new materials and possible materials increases, and because there are only so many humans with the necessary skills and knowledge to do this kind of work, those human researchers have become kind of a bottleneck: good at what they do, but mostly tasked with responsibilities that can be automated, at least to some degree, their hands and eyes replaced with robot versions of the same, nothing lost in the transition and possibly a lot to be gained by swapping them out, including the optimization of those boring, predictable processes, and the ability to work more AI into the loop, those AI empowered to make more predictions and assumptions as new data from these experiments roll in, further speeding up the process of development and further optimizing the economics of such research, alongside the tangible fruits of that research.
All of this, of course, is still bleeding-edge new, and there's a nonzero chance that some component of it ends up being not as useful or accurate as predicted or claimed, or that there will be some other glaring flaw that makes it not as desirable as it currently seems to be.
And that might mean we have some wonderful new predictions to work from, but are stuck with the same plodding pace of working through them—or in contrast, maybe those predictions turn out to be not as great as advertised, and instead we have super-fast experimental robots in our arsenal, but a much smaller menu of potential materials to work through, limiting what we can do with those self-driving laboratories—at least in this field, at this moment.
This is a maybe quite exciting moment for a field that touches essentially every other field, though, and if even a single-digit percentage of the purported possibilities of these new developments turn out to be accurate and manifestable, a lot of things could change very quickly, across many aspects of many industries, similar to the development of steel or plastics, but possibly even more rapidly deployed, and at a scale that the folks innovating those earlier wonder materials couldn't have dreamed of.
Show Notes
https://www.nature.com/articles/s41586-023-06734-w
https://www.nature.com/articles/s41586-023-06735-9
https://www.mtu.edu/materials/what/
https://interestingengineering.com/innovation/google-deepmind-invents-400000-materials
https://journals.aps.org/prl/abstract/10.1103/PhysRevLett.131.218401
https://en.wikipedia.org/wiki/AlphaFold
https://deepmind.google/discover/blog/millions-of-new-materials-discovered-with-deep-learning/
https://www.science.org/doi/10.1126/sciadv.aaz8867
https://www.nature.com/articles/s44160-022-00231-0
https://en.wikipedia.org/wiki/Ceramic
https://en.wikipedia.org/wiki/Stainless_steel
https://en.wikipedia.org/wiki/Materials_science
https://en.wikipedia.org/wiki/History_of_materials_science
4.8
506506 ratings
This week we talk about stainless steel, DARPA, and GNoME.
We also discuss ceramics, DeepMind, and self-driving labs.
Recommended Book: Drunk On All Your Strange New Words by Eddie Robson
Transcript
In a recent episode, I talked a bit about the bronze and copper ages, and how reaching the level of technological know-how so that it's possible to heat metals so you can blend them with other metals, forge them into useful things, and generally work with them in a more fundamental way than is possible if you're simply chipping away at them, bending them with brute strength, and so on, grants you all sorts of additional powers that those cruder methods do not offer.
Copper's a pretty basic material to work with, as metals go, in part because of its elemental properties, and in part because it appears in nature, on Earth, in its pure form, so it's not something our ancestors would have had to imagine from whole cloth—they could see it, work with it, and thus, had a pretty good sense of what it was and what it was capable of.
Bronze, an alloy of copper—with some amount of tin mixed into the copper to make it more resilient and strong, and thus, useful for many things—was different in that it's not natural and doesn't occur unless we synthetically produce it.
Iron is similar to copper in that it's natural, though it's also a lot stronger and thus harder to work with, lacking the metallurgical capacity to melt it down and reshape it in a liquified form, and steel is in this way a bit like bronze in that it's an alloy of iron—iron mixed with carbon—and variations on the theme, like stainless steels, have some amount of chromium blended in with the iron and carbon, alongside nickel, in some cases, which makes it even more complex, and thus essentially impossible to imagine if you're limited to what nature provides you, in terms of practicality, and thus, often at least, your conception of materials-related possibilities.
So part of the challenge in attaining mastery over difference materials, including but not limited to metals, is discovering them and having access to the requisite natural resources, like iron and copper, in the first place, but then also, over time, learning that you can manipulate them in various ways, and then over time—often long, long stretches of time, generationally long periods of time in some cases—refining those methods of manipulation until it's possible to do so economically, but also, typically, at some kind of productive scale: allowing you to make enough of the material so you can churn out, for instance, armor and swords made out of it, or if we're talking about ceramic goods, stuff made of clay and silica and carbon, among other substances, scaling-up the process so you can produce more jugs and pots and urns, more food-preservation technologies and clay tablets for writing and bricks for building homes and other structures; and that's alongside the parallel process of simply learning how to capably work with these materials, once a sufficient volume of them becomes available.
So while metal and clay are different sorts of substances, they're both materials that we use to make objects—we take basic, earth-derived stuff and reshape it into things that are useful to us in some way, whether that means as a weapon or means of manufacturing things, or as clothing, homes, or objects of beauty—artworks and such.
Materials science is a field focused on the many facets of these types of resources, with some practitioners working with existing materials in order to better understand them, others sussing out various means of scaling-up production or iterating upon existing modes of production to make them more economical or sustainable, while still others aim to produce new materials of this kind: in some cases discovering existing-but-rare new materials, in the sense that we haven't discovered them, at least in the scientific sense, before, but often production, in this context, means combining different elements or other raw materials to create new materials.
Just like our ancestors figured out how to make stronger, longer-lasting ceramic pots and how to make stainless steal out of iron alloyed with other substances, the contemporary version of that field often means working in laboratories and manufacturing hubs to investigate the blending-potential of various materials, and to then refine successful blends to see if the resulting whatever might have utility that can be exploited for some kind of productive purpose.
What I'd like to talk about today is materials science, and how new innovations in the AI realm could push this field into an entirely new, and much faster-moving, paradigm.
—
As I mentioned in the intro, we've been doing what you might call materials science research and development since our earliest days of civilizational evolution, and almost certainly for quite a long while before that, too, because our deep, deep ancestors were all about making clever use of their environments and the materials in those environments, to get a leg-up over their competition.
That said, modern materials science arose out of earlier, differentiated fields like metallurgy and ceramics engineering classes and laboratories, some of these educational and commercial hubs slammed together into unified, materials science departments in the 1960s when the US Advanced Research Projects Agency—the precursor to the Defense Advanced Research Projects Agency, or DARPA—started throwing money at universities with laboratories that seemed capable of helping the US economy, and by association the US military, gain broad-scale advantages over their international competition, by approaching materials research not just from the 30,000-foot, macro-scale view that pretty much every such department had approached such things from until this point, but also the micro-scale, atomic-level perspective: something more fields were beginning to attempt in the wake of WWII and the increasingly common realization that we've been missing out on a lot, not looking at things from the atomic level, up till that point, and that by leveraging advanced understandings about how these substances work from other fields, like physics, we could probably speed-up our development of new incredibly useful, omni-versatile materials, like steel or aluminum, dramatically.
This would allow us to start our research with assumptions based on molecular and atomic science, rather than empirical, observational, comparably quite slow approaches, and that meant rather than waiting to observe and measure something interesting that happened, usually by doing a lot of fiddling around and hoping for good luck, over and over, day after day, we could instead very intentionally start cycling through all the potential blends that these other scientific understandings have told us are both possible and might be useful or interesting for various reasons.
In the decades since, materials science has expanded still-further, encompassing new and ever-smaller scales, and new material types, like polymers—plastics, basically—that weren't really a thing when the unified field first, itself, became a thing.
The impact this reorganization and refocus has had on the development of new materials cannot be overstated: among other things, innovations in this space has led to the development of artificial skin for burn victims, metal composites that have worked their way into all kinds of consumer products, making them more durable and lightweight, the production of medical hardware capable of performing magnetic resonance imaging and ultrasounds, the materials required to produce microchips of ever-smaller sizes, but with ever-denser capacities, nanotechnologies that have allowed for the shrinking of all sorts of components and devices, and the materials that have made the rapidly increasing efficiencies of solar panels possible, alongside the materials used in wind turbine blades and batteries with ever-embiggening capacities, safety features, and durabilities.
The modern world, in essence, all modern technologies, and especially all digital goods, but also everything made out of any kind of metal or plastic that isn't raw iron or copper, both of which are increasingly rare in consumer goods, at least, was enabled by the field of materials science; lacking that mid-20th century development, it's a fair bet we would have been held back in pretty much every other scientific field, and thus, technological development, as well.
That ubiquity and importance is part of why a recent announcement by Google's DeepMind division—an artificial intelligence lab under the larger company's brand-umbrella—has been getting so much attention.
DeepMind has become well-known for its upending of chess, the game of Go, and more recently for creating a protein structure database that contains all its predictions for the 3D structures of folded proteins—showing how more than 200 million proteins will likely look based on their amino acid sequences, alone, solving what has long been called the "protein folding problem," which I spoke about at greater length in a previous episode, by the way.
So we've got a database full of protein ingredients, amino acids, for all the proteins we've ever discovered, but just having those ingredients doesn't tell us what the finished proteins will look like in three-dimensions, once they've been built, because they fold up into a final shape after construction.
Figuring out how finished, folded proteins made up of those ingredients we knew about, how they would actually look in real-life, has thus been a time-consuming, ponderous and expensive effort—all of science, our entire human civilization-wide scientific effort, was able to demonstrate the final, folded structures of something like 170,000 of the more than 200 million proteins we knew about, up till the early 2020s.
That changed with DeepMind's AlphaFold program, which—using an AI technique called deep learning—was able to predict, imperfectly, but with enough accuracy to successfully predict single-mutation effects (what will happen if a protein has a single change to one of its amino acids, and how that will impact the final shape of the folder protein) all of those known proteins in our existing database.
So predictions that are usable for many use-cases, and at what's been called a borderline miraculous or magical scale, applying this prediction model to every single protein we know about, as a species, at this point.
That same lab has now applied a similar AI system to predicting and simulating how various materials will work together, if blended, and how their fusion, the product of that blending, will behave; what properties it will have.
The company announced that they've developed a new deep learning system optimized for this purpose, called Graph Networks for Materials Exploration, or GNoME, and the initial outcome of running this tool was the discovery of about 2.2 million new crystalline structures, about 380,000 of which are stable enough to warrant further materials science investigation.
Using current methods and extrapolating on the research currently being done and funding currently available to researchers in this space around the world, it's estimated that around 736 of these 380,000 new potential materials have already been discovered by researchers in experimental settings, and that this stockpile is equivalent to about 800 years'-worth of knowledge based on current levels of investment and output.
So it would take about 800 years, at current levels of research in this space, to discover this many new potentially useful materials.
All of which is wonderful, as—like with the folded protein predictions provided by AlphaFold—this new GNoME model gives materials scientists some focused areas to be looking at, making every experiment more likely to provide us with useable outcomes, rather than the shot-in-the-dark approach that's more common when looking into unfamiliar blends of materials.
Many of these 380,000 potential new structures will likely be not useful for today's purposes, then, but this type of research rigs the dice so that each investigation is relatively more likely to yield something really valuable, which could prove to be hugely beneficial, especially since that catalog of potentially useful structures, like the protein fold catalog, has been published and made available to whomever wants it, for free.
That's still a lot of work to do, of course, churning through all these potentially useful materials, which is why another development in this space—what's sometimes called self-driving labs—is also notable and potentially vital for the more-rapid development of materials science.
Self-driving labs are basically lab spaces optimized for robotics that allow non-human, robot arms and other hardware, to perform the requisite, and often slogging, ponderous, tedious work of basic materials science experimentation, safely and continuously, around the clock.
So just as you might automate a fast-food restaurant by telling some software what ingredients to combine and how to process them, in order to make a burger or some fries, keeping tabs on the temperature of everything and what's been mixed with what along the way, using specialized, automated equipment, you can also tell some software which materials to combine, and how, and have it keep track of everything's properties throughout the process using an array of sensors, and then some robot arms perhaps, or maybe just a big box with pipes and the ability to move stuff from here to there when prudent, will combine a slew of varied substances from a catalog of options, and then keep tabs on the resulting materials, tucking away examples for further, human exploration and confirmation if they're auto-tagged as being interesting for the sorts of properties we want to see, but otherwise maybe just categorizing them according to their properties, adding to the body of knowledge we already have for such things, and giving us a sort of materials reference library that we can tap into when we need a specific material with specific attributes, in the future.
What this potentially does, then, is robotically automate the checking of the AI-generated catalog of potentially useful materials.
The degree to which this could change the field cannot be overstated, as while that earlier, 1960s-era formalization of the field, combining earlier realms of inquiry was a big deal, changing everything, this next step could do the same, replacing humans—who are in many cases doing systematic, tedious work—with sleepless, emotionless, unkillable robots working from software-generated possibilities in order to provide us with a new menu of materials we might use, moving forward.
This sort of development is especially important, arguably, because of all these new possibilities we now have available to try out: the number of possible combinations grows incredibly rapidly as the number of new materials and possible materials increases, and because there are only so many humans with the necessary skills and knowledge to do this kind of work, those human researchers have become kind of a bottleneck: good at what they do, but mostly tasked with responsibilities that can be automated, at least to some degree, their hands and eyes replaced with robot versions of the same, nothing lost in the transition and possibly a lot to be gained by swapping them out, including the optimization of those boring, predictable processes, and the ability to work more AI into the loop, those AI empowered to make more predictions and assumptions as new data from these experiments roll in, further speeding up the process of development and further optimizing the economics of such research, alongside the tangible fruits of that research.
All of this, of course, is still bleeding-edge new, and there's a nonzero chance that some component of it ends up being not as useful or accurate as predicted or claimed, or that there will be some other glaring flaw that makes it not as desirable as it currently seems to be.
And that might mean we have some wonderful new predictions to work from, but are stuck with the same plodding pace of working through them—or in contrast, maybe those predictions turn out to be not as great as advertised, and instead we have super-fast experimental robots in our arsenal, but a much smaller menu of potential materials to work through, limiting what we can do with those self-driving laboratories—at least in this field, at this moment.
This is a maybe quite exciting moment for a field that touches essentially every other field, though, and if even a single-digit percentage of the purported possibilities of these new developments turn out to be accurate and manifestable, a lot of things could change very quickly, across many aspects of many industries, similar to the development of steel or plastics, but possibly even more rapidly deployed, and at a scale that the folks innovating those earlier wonder materials couldn't have dreamed of.
Show Notes
https://www.nature.com/articles/s41586-023-06734-w
https://www.nature.com/articles/s41586-023-06735-9
https://www.mtu.edu/materials/what/
https://interestingengineering.com/innovation/google-deepmind-invents-400000-materials
https://journals.aps.org/prl/abstract/10.1103/PhysRevLett.131.218401
https://en.wikipedia.org/wiki/AlphaFold
https://deepmind.google/discover/blog/millions-of-new-materials-discovered-with-deep-learning/
https://www.science.org/doi/10.1126/sciadv.aaz8867
https://www.nature.com/articles/s44160-022-00231-0
https://en.wikipedia.org/wiki/Ceramic
https://en.wikipedia.org/wiki/Stainless_steel
https://en.wikipedia.org/wiki/Materials_science
https://en.wikipedia.org/wiki/History_of_materials_science
4,226 Listeners
10,377 Listeners
26,362 Listeners
2,380 Listeners
482 Listeners
43,397 Listeners
72 Listeners
918 Listeners
2,203 Listeners
24 Listeners
5,425 Listeners
15,206 Listeners
2,048 Listeners
11 Listeners
391 Listeners
405 Listeners