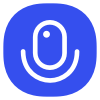
Sign up to save your podcasts
Or
Hey everyone, thank you so much for watching the 77th Weaviate Podcast on RAGAS, featuring Jithin James, Shahul ES, and Erika Cardenas! RAGAS is one of the hottest rising startups in Retrieval-Augmented Generation! RAGAS began it's journey with the RAGAS score, a matrix of evaluations for generation and retrieval. Generation evaluated on Faithfulness (is the response grounded in the context) as well as Relevancy (is the response useful). Retrieval is then evaluated on Precision (How many of the search results are relevant to the question?) and Recall (How many of the relevant search results are captured in the retrieved results?). Now, the super novel thing about this is that an LLM is used to determine these metrics. So we circumvent painstaking manual labeling effort with the RAGAS score! This podcast dives into the development of the RAGAS score as well as how RAG application builders should think about the knobs to tune for optimizing their RAGAS score: embedding models, chunking strategies, hybrid search tuning, rerankers, ... ?!? We also discussed tons of exciting directions for the future such as fine-tuning smaller LLMs for these metrics, agents that use tuning APIs, and long context RAG!
4
44 ratings
Hey everyone, thank you so much for watching the 77th Weaviate Podcast on RAGAS, featuring Jithin James, Shahul ES, and Erika Cardenas! RAGAS is one of the hottest rising startups in Retrieval-Augmented Generation! RAGAS began it's journey with the RAGAS score, a matrix of evaluations for generation and retrieval. Generation evaluated on Faithfulness (is the response grounded in the context) as well as Relevancy (is the response useful). Retrieval is then evaluated on Precision (How many of the search results are relevant to the question?) and Recall (How many of the relevant search results are captured in the retrieved results?). Now, the super novel thing about this is that an LLM is used to determine these metrics. So we circumvent painstaking manual labeling effort with the RAGAS score! This podcast dives into the development of the RAGAS score as well as how RAG application builders should think about the knobs to tune for optimizing their RAGAS score: embedding models, chunking strategies, hybrid search tuning, rerankers, ... ?!? We also discussed tons of exciting directions for the future such as fine-tuning smaller LLMs for these metrics, agents that use tuning APIs, and long context RAG!
1,269 Listeners
31,907 Listeners
507 Listeners
43,385 Listeners
244 Listeners
440 Listeners
111,110 Listeners
207 Listeners
187 Listeners
8,758 Listeners
129 Listeners
39 Listeners
72 Listeners
10 Listeners
33 Listeners