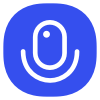
Sign up to save your podcasts
Or
Today we’re joined by Jonathan Le Roux, a senior principal research scientist at Mitsubishi Electric Research Laboratories (MERL). At MERL, Jonathan and his team are focused on using machine learning to solve the “cocktail party problem”, focusing on not only the separation of speech from noise, but also the separation of speech from speech. In our conversation with Jonathan, we focus on his paper The Cocktail Fork Problem: Three-Stem Audio Separation For Real-World Soundtracks, which looks to separate and enhance a complex acoustic scene into three distinct categories, speech, music, and sound effects. We explore the challenges of working with such noisy data, the model architecture used to solve this problem, how ML/DL fits into solving the larger cocktail party problem, future directions for this line of research, and much more!
The complete show notes for this episode can be found at twimlai.com/go/555
4.7
414414 ratings
Today we’re joined by Jonathan Le Roux, a senior principal research scientist at Mitsubishi Electric Research Laboratories (MERL). At MERL, Jonathan and his team are focused on using machine learning to solve the “cocktail party problem”, focusing on not only the separation of speech from noise, but also the separation of speech from speech. In our conversation with Jonathan, we focus on his paper The Cocktail Fork Problem: Three-Stem Audio Separation For Real-World Soundtracks, which looks to separate and enhance a complex acoustic scene into three distinct categories, speech, music, and sound effects. We explore the challenges of working with such noisy data, the model architecture used to solve this problem, how ML/DL fits into solving the larger cocktail party problem, future directions for this line of research, and much more!
The complete show notes for this episode can be found at twimlai.com/go/555
161 Listeners
481 Listeners
299 Listeners
323 Listeners
147 Listeners
265 Listeners
189 Listeners
290 Listeners
88 Listeners
122 Listeners
197 Listeners
76 Listeners
442 Listeners
30 Listeners
36 Listeners