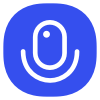
Sign up to save your podcasts
Or
Vasek Mlejnsky from E2B joins us today to talk about sandboxes for AI agents. In the last 2 years, E2B has grown from a handful of developers building on it to being used by ~50% of the Fortune 500 and generating millions of sandboxes each week for their customers. As the “death of chat completions” approaches, LLMs workflows and agents are relying more and more on tool usage and multi-modality.
The most common use cases for their sandboxes:
- Run data analysis and charting (like Perplexity)
- Execute arbitrary code generated by the model (like Manus does)
- Running evals on code generation (see LMArena Web)
- Doing reinforcement learning for code capabilities (like HuggingFace)
Timestamps:
00:00:00 Introductions
00:00:37 Origin of DevBook -> E2B
00:02:35 Early Experiments with GPT-3.5 and Building AI Agents
00:05:19 Building an Agent Cloud
00:07:27 Challenges of Building with Early LLMs
00:10:35 E2B Use Cases
00:13:52 E2B Growth vs Models Capabilities
00:15:03 The LLM Operating System (LLMOS) Landscape
00:20:12 Breakdown of JavaScript vs Python Usage on E2B
00:21:50 AI VMs vs Traditional Cloud
00:26:28 Technical Specifications of E2B Sandboxes
00:29:43 Usage-based billing infrastructure
00:34:08 Pricing AI on Value Delivered vs Token Usage
00:36:24 Forking, Checkpoints, and Parallel Execution in Sandboxes
00:39:18 Future Plans for Toolkit and Higher-Level Agent Frameworks
00:42:35 Limitations of Chat-Based Interfaces and the Future of Agents
00:44:00 MCPs and Remote Agent Capabilities
00:49:22 LLMs.txt, scrapers, and bad AI bots
00:53:00 Manus and Computer Use on E2B
00:55:03 E2B for RL with Hugging Face
00:56:58 E2B for Agent Evaluation on LMArena
00:58:12 Long-Term Vision: E2B as Full Lifecycle Infrastructure for LLMs
01:00:45 Future Plans for Hosting and Deployment of LLM-Generated Apps
01:01:15 Why E2B Moved to San Francisco
01:05:49 Open Roles and Hiring Plans at E2B
4.8
5858 ratings
Vasek Mlejnsky from E2B joins us today to talk about sandboxes for AI agents. In the last 2 years, E2B has grown from a handful of developers building on it to being used by ~50% of the Fortune 500 and generating millions of sandboxes each week for their customers. As the “death of chat completions” approaches, LLMs workflows and agents are relying more and more on tool usage and multi-modality.
The most common use cases for their sandboxes:
- Run data analysis and charting (like Perplexity)
- Execute arbitrary code generated by the model (like Manus does)
- Running evals on code generation (see LMArena Web)
- Doing reinforcement learning for code capabilities (like HuggingFace)
Timestamps:
00:00:00 Introductions
00:00:37 Origin of DevBook -> E2B
00:02:35 Early Experiments with GPT-3.5 and Building AI Agents
00:05:19 Building an Agent Cloud
00:07:27 Challenges of Building with Early LLMs
00:10:35 E2B Use Cases
00:13:52 E2B Growth vs Models Capabilities
00:15:03 The LLM Operating System (LLMOS) Landscape
00:20:12 Breakdown of JavaScript vs Python Usage on E2B
00:21:50 AI VMs vs Traditional Cloud
00:26:28 Technical Specifications of E2B Sandboxes
00:29:43 Usage-based billing infrastructure
00:34:08 Pricing AI on Value Delivered vs Token Usage
00:36:24 Forking, Checkpoints, and Parallel Execution in Sandboxes
00:39:18 Future Plans for Toolkit and Higher-Level Agent Frameworks
00:42:35 Limitations of Chat-Based Interfaces and the Future of Agents
00:44:00 MCPs and Remote Agent Capabilities
00:49:22 LLMs.txt, scrapers, and bad AI bots
00:53:00 Manus and Computer Use on E2B
00:55:03 E2B for RL with Hugging Face
00:56:58 E2B for Agent Evaluation on LMArena
00:58:12 Long-Term Vision: E2B as Full Lifecycle Infrastructure for LLMs
01:00:45 Future Plans for Hosting and Deployment of LLM-Generated Apps
01:01:15 Why E2B Moved to San Francisco
01:05:49 Open Roles and Hiring Plans at E2B
994 Listeners
474 Listeners
431 Listeners
293 Listeners
323 Listeners
194 Listeners
279 Listeners
90 Listeners
333 Listeners
122 Listeners
191 Listeners
419 Listeners
26 Listeners
16 Listeners
30 Listeners