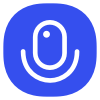
Sign up to save your podcasts
Or
Ben Shneiderman is a leading figure in the field of human-computer interaction (HCI).
Having founded one of the oldest HCI research centers in the country at the University of Maryland in 1983, Shneiderman has been intently studying the design of computer technology and its use by humans. Currently, Ben is a Distinguished University Professor in the Department of Computer Science at the University of Maryland and is working on a new book on human-centered artificial intelligence.
I’m so excited to welcome this expert from the field of UX and design to today’s episode of Experiencing Data! Ben and I talked a lot about the complex intersection of human-centered design and AI systems.
In our chat, we covered:
The software engineering teams creating AI systems have got real work to do. They need the right kind of workflows, engineering patterns, and Agile development methods that will work for AI. The AI world is different because it’s not just programming, but it also involves the use of data that’s used for training. The key distinction is that the data that drives the AI has to be the appropriate data, it has to be unbiased, it has to be fair, it has to be appropriate to the task at hand. And many people and many companies are coming to grips with how to manage that. This has become controversial, let’s say, in issues like granting parole, or mortgages, or hiring people. There was a controversy that Amazon ran into when its hiring algorithm favored men rather than women. There’s been bias in facial recognition algorithms, which were less accurate with people of color. That’s led to some real problems in the real world. And that’s where we have to make sure we do a much better job and the tools of human-computer interaction are very effective in building these better systems in testing and evaluating. - Ben (6:10)
Every company will tell you, “We do a really good job in checking out our AI systems.” That’s great. We want every company to do a really good job. But we also want independent oversight of somebody who’s outside the company — someone who knows the field, who’s looked at systems at other companies, and who can bring ideas and bring understanding of the dangers as well. These systems operate in an adversarial environment — there are malicious actors out there who are causing trouble. You need to understand what the dangers and threats are to the use of your system. You need to understand where the biases come from, what dangers are there, and where the software has failed in other places. You may know what happens in your company, but you can benefit by learning what happens outside your company, and that’s where independent oversight from accounting companies, from governmental regulators, and from other independent groups is so valuable. - Ben (15:04)
There’s no such thing as an autonomous device. Someone owns it; somebody’s responsible for it; someone starts it; someone stops it; someone fixes it; someone notices when it’s performing poorly. … Responsibility is a pretty key factor here. So, if there’s something going on, if a manager is deciding to use some AI system, what they need is a control panel, let them know: what’s happening? What’s it doing? What’s going wrong and what’s going right? That kind of supervisory autonomy is what I talk about, not full machine autonomy that’s hidden away and you never see it because that’s just head-in-the-sand thinking. What you want to do is expose the operation of a system, and where possible, give the stakeholders who are responsible for performance the right kind of control panel and the right kind of data. … Feedback is the breakfast of champions. And companies know that. They want to be able to measure the success stories, and they want to know their failures, so they can reduce them. The continuous improvement mantra is alive and well. We do want to keep tracking what’s going on and make sure it gets better. Every quarter. - Ben (19:41)
Google has had some issues regarding hiring in the AI research area, and so has Facebook with elections and the way that algorithms tend to become echo chambers. These companies — and this is not through heavy research — probably have the heaviest investment of user experience professionals within data science organizations. They have UX, ML-UX people, UX for AI people, they’re at the cutting edge. I see a lot more generalist designers in most other companies. Most of them are rather unfamiliar with any of this or what the ramifications are on the design work that they’re doing. But even these largest companies that have, probably, the biggest penetration into the most number of people out there are getting some of this really important stuff wrong. - Brian (26:36)
5
3939 ratings
Ben Shneiderman is a leading figure in the field of human-computer interaction (HCI).
Having founded one of the oldest HCI research centers in the country at the University of Maryland in 1983, Shneiderman has been intently studying the design of computer technology and its use by humans. Currently, Ben is a Distinguished University Professor in the Department of Computer Science at the University of Maryland and is working on a new book on human-centered artificial intelligence.
I’m so excited to welcome this expert from the field of UX and design to today’s episode of Experiencing Data! Ben and I talked a lot about the complex intersection of human-centered design and AI systems.
In our chat, we covered:
The software engineering teams creating AI systems have got real work to do. They need the right kind of workflows, engineering patterns, and Agile development methods that will work for AI. The AI world is different because it’s not just programming, but it also involves the use of data that’s used for training. The key distinction is that the data that drives the AI has to be the appropriate data, it has to be unbiased, it has to be fair, it has to be appropriate to the task at hand. And many people and many companies are coming to grips with how to manage that. This has become controversial, let’s say, in issues like granting parole, or mortgages, or hiring people. There was a controversy that Amazon ran into when its hiring algorithm favored men rather than women. There’s been bias in facial recognition algorithms, which were less accurate with people of color. That’s led to some real problems in the real world. And that’s where we have to make sure we do a much better job and the tools of human-computer interaction are very effective in building these better systems in testing and evaluating. - Ben (6:10)
Every company will tell you, “We do a really good job in checking out our AI systems.” That’s great. We want every company to do a really good job. But we also want independent oversight of somebody who’s outside the company — someone who knows the field, who’s looked at systems at other companies, and who can bring ideas and bring understanding of the dangers as well. These systems operate in an adversarial environment — there are malicious actors out there who are causing trouble. You need to understand what the dangers and threats are to the use of your system. You need to understand where the biases come from, what dangers are there, and where the software has failed in other places. You may know what happens in your company, but you can benefit by learning what happens outside your company, and that’s where independent oversight from accounting companies, from governmental regulators, and from other independent groups is so valuable. - Ben (15:04)
There’s no such thing as an autonomous device. Someone owns it; somebody’s responsible for it; someone starts it; someone stops it; someone fixes it; someone notices when it’s performing poorly. … Responsibility is a pretty key factor here. So, if there’s something going on, if a manager is deciding to use some AI system, what they need is a control panel, let them know: what’s happening? What’s it doing? What’s going wrong and what’s going right? That kind of supervisory autonomy is what I talk about, not full machine autonomy that’s hidden away and you never see it because that’s just head-in-the-sand thinking. What you want to do is expose the operation of a system, and where possible, give the stakeholders who are responsible for performance the right kind of control panel and the right kind of data. … Feedback is the breakfast of champions. And companies know that. They want to be able to measure the success stories, and they want to know their failures, so they can reduce them. The continuous improvement mantra is alive and well. We do want to keep tracking what’s going on and make sure it gets better. Every quarter. - Ben (19:41)
Google has had some issues regarding hiring in the AI research area, and so has Facebook with elections and the way that algorithms tend to become echo chambers. These companies — and this is not through heavy research — probably have the heaviest investment of user experience professionals within data science organizations. They have UX, ML-UX people, UX for AI people, they’re at the cutting edge. I see a lot more generalist designers in most other companies. Most of them are rather unfamiliar with any of this or what the ramifications are on the design work that they’re doing. But even these largest companies that have, probably, the biggest penetration into the most number of people out there are getting some of this really important stuff wrong. - Brian (26:36)
272 Listeners
1,830 Listeners
1,033 Listeners
480 Listeners
137 Listeners
3,987 Listeners
1,784 Listeners
441 Listeners
298 Listeners
140 Listeners
3,995 Listeners
267 Listeners
192 Listeners
88 Listeners
144 Listeners