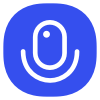
Sign up to save your podcasts
Or
Lots of people in the AI safety space worry about models being able to make deliberate, multi-step plans. But can we already see this in existing neural nets? In this episode, I talk with Erik Jenner about his work looking at internal look-ahead within chess-playing neural networks.
Patreon: https://www.patreon.com/axrpodcast
Ko-fi: https://ko-fi.com/axrpodcast
The transcript: https://axrp.net/episode/2024/12/12/episode-38_3-erik-jenner-learned-look-ahead.html
FAR.AI: https://far.ai/
FAR.AI on X (aka Twitter): https://x.com/farairesearch
FAR.AI on YouTube: https://www.youtube.com/@FARAIResearch
The Alignment Workshop: https://www.alignment-workshop.com/
Topics we discuss, and timestamps:
00:57 - How chess neural nets look into the future
04:29 - The dataset and basic methodology
05:23 - Testing for branching futures?
07:57 - Which experiments demonstrate what
10:43 - How the ablation experiments work
12:38 - Effect sizes
15:23 - X-risk relevance
18:08 - Follow-up work
21:29 - How much planning does the network do?
Research we mention:
Evidence of Learned Look-Ahead in a Chess-Playing Neural Network: https://arxiv.org/abs/2406.00877
Understanding the learned look-ahead behavior of chess neural networks (a development of the follow-up research Erik mentioned): https://openreview.net/forum?id=Tl8EzmgsEp
Linear Latent World Models in Simple Transformers: A Case Study on Othello-GPT: https://arxiv.org/abs/2310.07582
Episode art by Hamish Doodles: hamishdoodles.com
4.4
88 ratings
Lots of people in the AI safety space worry about models being able to make deliberate, multi-step plans. But can we already see this in existing neural nets? In this episode, I talk with Erik Jenner about his work looking at internal look-ahead within chess-playing neural networks.
Patreon: https://www.patreon.com/axrpodcast
Ko-fi: https://ko-fi.com/axrpodcast
The transcript: https://axrp.net/episode/2024/12/12/episode-38_3-erik-jenner-learned-look-ahead.html
FAR.AI: https://far.ai/
FAR.AI on X (aka Twitter): https://x.com/farairesearch
FAR.AI on YouTube: https://www.youtube.com/@FARAIResearch
The Alignment Workshop: https://www.alignment-workshop.com/
Topics we discuss, and timestamps:
00:57 - How chess neural nets look into the future
04:29 - The dataset and basic methodology
05:23 - Testing for branching futures?
07:57 - Which experiments demonstrate what
10:43 - How the ablation experiments work
12:38 - Effect sizes
15:23 - X-risk relevance
18:08 - Follow-up work
21:29 - How much planning does the network do?
Research we mention:
Evidence of Learned Look-Ahead in a Chess-Playing Neural Network: https://arxiv.org/abs/2406.00877
Understanding the learned look-ahead behavior of chess neural networks (a development of the follow-up research Erik mentioned): https://openreview.net/forum?id=Tl8EzmgsEp
Linear Latent World Models in Simple Transformers: A Case Study on Othello-GPT: https://arxiv.org/abs/2310.07582
Episode art by Hamish Doodles: hamishdoodles.com
26,462 Listeners
2,395 Listeners
1,784 Listeners
298 Listeners
105 Listeners
4,142 Listeners
89 Listeners
287 Listeners
88 Listeners
417 Listeners
243 Listeners
75 Listeners
60 Listeners
146 Listeners
123 Listeners