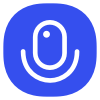
Sign up to save your podcasts
Or
For many people, the process of buying and selling a home will undoubtedly be the most difficult decisions they will make in their lifetime. Is the price you’re paying for your home fair? Is the price you’re selling your home for an adequate sale price? For a long time, realtors have been oracles with the answers to those questions, but times are changing. Today, Opendoor, a data-driven real estate start-up, is putting data to work to answer those questions for you.
“Opendoor from a technical perspective, is the hands down single most fascinating technical challenge I've ever come across. If you look at the questions that we have to answer, they're just fascinating and you have to answer them in the right ways. Otherwise they just don't really work. You think about the prediction problem, you think about the optimization, probably you think about the portfolio optimization problem. There's so many different pieces to the problem and you just have to do it.”
Kushal Chakrabarti is the VP of Research and Data Science at Opendoor, a company that is reimagining the way homes are bought and sold by moving the process online and empowering buyers and sellers to make informed decisions by taking an algorithmic approach and removing the ambiguous nature of the home buying process. On this episode of IT Visionaries, Kushal explains some of the processes Opendoor uses to help make home offers, including the importance of clean and trustworthy data. Plus, Kushal opens up on his personal journey, including how he got into data science and some of the trends he sees in the A.I. and machine learning space.
Main Takeaways
IT Visionaries is brought to you by the Salesforce Platform - the #1 cloud platform for digital transformation of every experience. Build connected experiences, empower every employee, and deliver continuous innovation - with the customer at the center of everything you do. Learn more at salesforce.com/platform
--
Brightspot is the leading content management platform built for modern teams. Your content lifecycle - from ideation to archival - moves faster, handling any volume, variety, or velocity with ease.
Unlike legacy platforms that demand heavy dev work and struggle with scale, Brightspot is user-friendly, endlessly customizable, and enterprise-ready. Think dynamic, modern experiences, shipped in minutes—not months.
Trusted by some of the world’s leading tech organizations, Brightspot reduces total cost of ownership, boosts productivity, and gives you the governance, security, and performance your teams need.
Visit brightspot.com/ITVisionaries to learn more.
---
This episode was produced by the team at Mission.org and brought to you by Brightspot.
4.6
170170 ratings
For many people, the process of buying and selling a home will undoubtedly be the most difficult decisions they will make in their lifetime. Is the price you’re paying for your home fair? Is the price you’re selling your home for an adequate sale price? For a long time, realtors have been oracles with the answers to those questions, but times are changing. Today, Opendoor, a data-driven real estate start-up, is putting data to work to answer those questions for you.
“Opendoor from a technical perspective, is the hands down single most fascinating technical challenge I've ever come across. If you look at the questions that we have to answer, they're just fascinating and you have to answer them in the right ways. Otherwise they just don't really work. You think about the prediction problem, you think about the optimization, probably you think about the portfolio optimization problem. There's so many different pieces to the problem and you just have to do it.”
Kushal Chakrabarti is the VP of Research and Data Science at Opendoor, a company that is reimagining the way homes are bought and sold by moving the process online and empowering buyers and sellers to make informed decisions by taking an algorithmic approach and removing the ambiguous nature of the home buying process. On this episode of IT Visionaries, Kushal explains some of the processes Opendoor uses to help make home offers, including the importance of clean and trustworthy data. Plus, Kushal opens up on his personal journey, including how he got into data science and some of the trends he sees in the A.I. and machine learning space.
Main Takeaways
IT Visionaries is brought to you by the Salesforce Platform - the #1 cloud platform for digital transformation of every experience. Build connected experiences, empower every employee, and deliver continuous innovation - with the customer at the center of everything you do. Learn more at salesforce.com/platform
--
Brightspot is the leading content management platform built for modern teams. Your content lifecycle - from ideation to archival - moves faster, handling any volume, variety, or velocity with ease.
Unlike legacy platforms that demand heavy dev work and struggle with scale, Brightspot is user-friendly, endlessly customizable, and enterprise-ready. Think dynamic, modern experiences, shipped in minutes—not months.
Trusted by some of the world’s leading tech organizations, Brightspot reduces total cost of ownership, boosts productivity, and gives you the governance, security, and performance your teams need.
Visit brightspot.com/ITVisionaries to learn more.
---
This episode was produced by the team at Mission.org and brought to you by Brightspot.
1,830 Listeners
365 Listeners
1,385 Listeners
1,876 Listeners
1,009 Listeners
112 Listeners
412 Listeners
9,555 Listeners
238 Listeners
166 Listeners
219 Listeners
40 Listeners
75 Listeners
276 Listeners
59 Listeners
6,447 Listeners
37 Listeners
5,312 Listeners
86 Listeners
136 Listeners
127 Listeners
18 Listeners
23 Listeners
58 Listeners
84 Listeners
151 Listeners
20 Listeners
1,102 Listeners