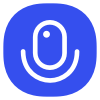
Sign up to save your podcasts
Or
Ethical concerns about the use of AI have to start with training data. Too often, the primary concern is simply generating sufficient data, rather than understanding its nature. Emily Jasper and Abby Simmons are back to continue the conversation started in episode 198 with host Eric Hanselman. With generative AI, the data is the application in its most formative sense. Unlike traditional application development, where the expectation is that functionality will be expanded in later releases, GenAI applications require careful design of training data before training takes place. The perspectives contained in data age rapidly and model training doesn’t differentiate between outdated and current indications. Old data can effectively poison model outputs. Businesses risk alienating customers with models that are trained with data that don’t properly represent them. This is particularly true with marginalized communities, where language and context can change over shorter time frames.
While there is research work on model retraining, work in AI today has to focus on effective data quality management. DeepSeek is causing a significant rethinking. Human data cleansing can be effective, but can’t scale to AI demands. Data workbench tools and synthetic data approaches can help, but better automation is needed to ensure that data sets are truly representative. Data collection and data sourcing need much greater attention to ensure that model results can engage the target audience and not be a liability. It’s a fundamental question of accountability that requires thinking in ways that are different than legacy development processes.
Mentioned in this episode:
More S&P Global Content:
Credits:
4.9
2828 ratings
Ethical concerns about the use of AI have to start with training data. Too often, the primary concern is simply generating sufficient data, rather than understanding its nature. Emily Jasper and Abby Simmons are back to continue the conversation started in episode 198 with host Eric Hanselman. With generative AI, the data is the application in its most formative sense. Unlike traditional application development, where the expectation is that functionality will be expanded in later releases, GenAI applications require careful design of training data before training takes place. The perspectives contained in data age rapidly and model training doesn’t differentiate between outdated and current indications. Old data can effectively poison model outputs. Businesses risk alienating customers with models that are trained with data that don’t properly represent them. This is particularly true with marginalized communities, where language and context can change over shorter time frames.
While there is research work on model retraining, work in AI today has to focus on effective data quality management. DeepSeek is causing a significant rethinking. Human data cleansing can be effective, but can’t scale to AI demands. Data workbench tools and synthetic data approaches can help, but better automation is needed to ensure that data sets are truly representative. Data collection and data sourcing need much greater attention to ensure that model results can engage the target audience and not be a liability. It’s a fundamental question of accountability that requires thinking in ways that are different than legacy development processes.
Mentioned in this episode:
More S&P Global Content:
Credits:
1,683 Listeners
32 Listeners
27 Listeners
7 Listeners
112,758 Listeners
39 Listeners
6,344 Listeners
11 Listeners
11 Listeners
4 Listeners
1,264 Listeners
60 Listeners
27 Listeners
41,523 Listeners
11 Listeners
4 Listeners
3 Listeners
315 Listeners
404 Listeners
19 Listeners
5,377 Listeners
1 Listeners
419 Listeners