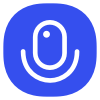
Sign up to save your podcasts
Or
Richad Nieves-Becker is a self-taught data scientist with an eclectic background. He currently leads the data science function at Revantage, a real estate shared service organization in the Blackstone family. He got a BA in Neuroscience and Anthropology. And was on the PhD path until he realized it was not for him. He pivoted and earned a Masters in Commerce from the University of Virginia. He started at CoreLogic focusing on text mining, then moved to Greystone leading all things data in an innovation lab. He credits his career progress to focusing on impact and deeply understanding the business case.
In this episode, we cover a range of topics including:
- His entry into data science
- Academic vs business work
- How he cold emailed his way to getting job interviews
- Why data scientists need sales skills
- How data scientists should think about building a portfolio
- Defining tractable problems in machine learning and data science
- Moving from mathematics to data science
- Framework for creating educational content
- Creating a course for data scientists
- How he interviews people
- The report he's writing for new data science leaders
- Building good culture by aligning an individual's desires to the company's goals
- The advantage of modular products
- Rise of MLOps and data versioning
- Monetization of models
4.9
88 ratings
Richad Nieves-Becker is a self-taught data scientist with an eclectic background. He currently leads the data science function at Revantage, a real estate shared service organization in the Blackstone family. He got a BA in Neuroscience and Anthropology. And was on the PhD path until he realized it was not for him. He pivoted and earned a Masters in Commerce from the University of Virginia. He started at CoreLogic focusing on text mining, then moved to Greystone leading all things data in an innovation lab. He credits his career progress to focusing on impact and deeply understanding the business case.
In this episode, we cover a range of topics including:
- His entry into data science
- Academic vs business work
- How he cold emailed his way to getting job interviews
- Why data scientists need sales skills
- How data scientists should think about building a portfolio
- Defining tractable problems in machine learning and data science
- Moving from mathematics to data science
- Framework for creating educational content
- Creating a course for data scientists
- How he interviews people
- The report he's writing for new data science leaders
- Building good culture by aligning an individual's desires to the company's goals
- The advantage of modular products
- Rise of MLOps and data versioning
- Monetization of models
1,000 Listeners
507 Listeners
2,290 Listeners
297 Listeners
387 Listeners
730 Listeners
282 Listeners
8,761 Listeners
353 Listeners
128 Listeners
73 Listeners
427 Listeners
433 Listeners
929 Listeners
37 Listeners