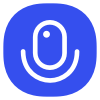
Sign up to save your podcasts
Or
On this episode of Unsupervised Learning Razib talks to Tade Souaiaia, a statistical geneticist at SUNY Downstate about his new preprint, Striking Departures from Polygenic Architecture in the Tails of Complex Traits. Souaiaia trained as a computational biologist at USC, but also has a background as a division I track and field athlete.
Razib and Souaiaia discuss what “genetic architecture” means, and consider what we're finding when we look at extreme trait values in characteristics along a normal distribution. Though traits like height or risk for type II diabetes can be thought of as represented by an idealized Gaussian distribution, real molecular and cellular processes still underlie their phenotypic expression. Souaiaia talks about how genomics has resulted in an influx of data and allowed statistical geneticists with a theoretical bent to actually test some of the models that underpin our understanding of traits and examine how models like mutation-selection balance might differ from what we’ve long expected. After wading through the depths of genetic abstraction and how it intersects with the new age of big data, Razib and Souaiaia talk about race and sports, and whether there might be differences between groups in athletic ability. Souaiaia argues that the underlying historical track record is too variable to draw firm conclusions, while Razib argues that there are theoretical reasons that one should expect differences between groups at the tails and even around the memes.
4.8
196196 ratings
On this episode of Unsupervised Learning Razib talks to Tade Souaiaia, a statistical geneticist at SUNY Downstate about his new preprint, Striking Departures from Polygenic Architecture in the Tails of Complex Traits. Souaiaia trained as a computational biologist at USC, but also has a background as a division I track and field athlete.
Razib and Souaiaia discuss what “genetic architecture” means, and consider what we're finding when we look at extreme trait values in characteristics along a normal distribution. Though traits like height or risk for type II diabetes can be thought of as represented by an idealized Gaussian distribution, real molecular and cellular processes still underlie their phenotypic expression. Souaiaia talks about how genomics has resulted in an influx of data and allowed statistical geneticists with a theoretical bent to actually test some of the models that underpin our understanding of traits and examine how models like mutation-selection balance might differ from what we’ve long expected. After wading through the depths of genetic abstraction and how it intersects with the new age of big data, Razib and Souaiaia talk about race and sports, and whether there might be differences between groups in athletic ability. Souaiaia argues that the underlying historical track record is too variable to draw firm conclusions, while Razib argues that there are theoretical reasons that one should expect differences between groups at the tails and even around the memes.
4,221 Listeners
587 Listeners
2,257 Listeners
2,359 Listeners
885 Listeners
812 Listeners
87 Listeners
3,749 Listeners
315 Listeners
806 Listeners
216 Listeners
262 Listeners
89 Listeners
68 Listeners
125 Listeners