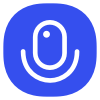
Sign up to save your podcasts
Or
“One very interesting question is what might we learn from recent developments in AI about how humans learn and process language. The full story is a little bit more complicated because, of course, humans don't learn from the same kind of data as language models. Language models learn from this internet-scraped data that includes New York Times articles, blog posts, thousands of books, a bunch of programming code, and so on. That's very different from the kind of linguistic input that children learn from, which is child-directed speech from parents and relatives that’s much simpler linguistically. That said, there is a lot of interesting research trying to train smaller language models on data that is similar to the kind of input that a child might receive when they are learning language. You can, for example, strap a head mounted camera on a young child's head for a few hours every day and record whatever auditory information the child has access to, which would include any language spoken around or directed at the child. You can then transcribe that, and use that data set to train a language model on the child-directed speech that a real human would have received during their development. So some artificial models are learning from child-directed speech, and that might eventually go some way towards advancing debates about what scientists and philosophers call “nativism versus empiricism” with respect to language acquisition—the nature/nurture debate: Are we born with a universal innate grammar that enables us to learn the rules of language, as linguists like Noam Chomsky have argued, or can we learn language and its grammatical rules just from raw data, just from hearing and being exposed to language as children?If these language models that are trained from this kind of realistic datasets of child-directed speech manage to learn grammar, to learn how to use language in the way children do, then that might put some pressure on the nativist claim that there is this innate component to language learning that is part of our DNA, as opposed to just learning from exposure to language itself.”
Dr. Raphaël Millière is Assistant Professor in Philosophy of AI at Macquarie University in Sydney, Australia. His research primarily explores the theoretical foundations and inner workings of AI systems based on deep learning, such as large language models. He investigates whether these systems can exhibit human-like cognitive capacities, drawing on theories and methods from cognitive science. He is also interested in how insights from studying AI might shed new light on human cognition. Ultimately, his work aims to advance our understanding of both artificial and natural intelligence.
https://raphaelmilliere.com
https://researchers.mq.edu.au/en/persons/raphael-milliere
www.creativeprocess.info
www.oneplanetpodcast.org
IG www.instagram.com/creativeprocesspodcast
5
4646 ratings
“One very interesting question is what might we learn from recent developments in AI about how humans learn and process language. The full story is a little bit more complicated because, of course, humans don't learn from the same kind of data as language models. Language models learn from this internet-scraped data that includes New York Times articles, blog posts, thousands of books, a bunch of programming code, and so on. That's very different from the kind of linguistic input that children learn from, which is child-directed speech from parents and relatives that’s much simpler linguistically. That said, there is a lot of interesting research trying to train smaller language models on data that is similar to the kind of input that a child might receive when they are learning language. You can, for example, strap a head mounted camera on a young child's head for a few hours every day and record whatever auditory information the child has access to, which would include any language spoken around or directed at the child. You can then transcribe that, and use that data set to train a language model on the child-directed speech that a real human would have received during their development. So some artificial models are learning from child-directed speech, and that might eventually go some way towards advancing debates about what scientists and philosophers call “nativism versus empiricism” with respect to language acquisition—the nature/nurture debate: Are we born with a universal innate grammar that enables us to learn the rules of language, as linguists like Noam Chomsky have argued, or can we learn language and its grammatical rules just from raw data, just from hearing and being exposed to language as children?If these language models that are trained from this kind of realistic datasets of child-directed speech manage to learn grammar, to learn how to use language in the way children do, then that might put some pressure on the nativist claim that there is this innate component to language learning that is part of our DNA, as opposed to just learning from exposure to language itself.”
Dr. Raphaël Millière is Assistant Professor in Philosophy of AI at Macquarie University in Sydney, Australia. His research primarily explores the theoretical foundations and inner workings of AI systems based on deep learning, such as large language models. He investigates whether these systems can exhibit human-like cognitive capacities, drawing on theories and methods from cognitive science. He is also interested in how insights from studying AI might shed new light on human cognition. Ultimately, his work aims to advance our understanding of both artificial and natural intelligence.
https://raphaelmilliere.com
https://researchers.mq.edu.au/en/persons/raphael-milliere
www.creativeprocess.info
www.oneplanetpodcast.org
IG www.instagram.com/creativeprocesspodcast
573 Listeners
747 Listeners
1,831 Listeners
216 Listeners
410 Listeners
289 Listeners
378 Listeners
1,536 Listeners
860 Listeners
775 Listeners
305 Listeners
59 Listeners
254 Listeners
372 Listeners
170 Listeners
251 Listeners
18 Listeners
18 Listeners
69 Listeners
51 Listeners
89 Listeners
33 Listeners
35 Listeners
35 Listeners
46 Listeners
32 Listeners
39 Listeners
26 Listeners
13 Listeners
141 Listeners
7 Listeners
7 Listeners
12 Listeners
3 Listeners