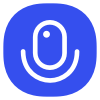
Sign up to save your podcasts
Or
Many trading strategies are developed using extensive historical data to calibrate model parameters. However, this process often leads to over-optimization, where the strategy is too finely tuned to past market conditions. Two things stand out:
Noise vs. Signal: Financial markets inherently contain a high degree of randomness. A model that fits historical data exceptionally well may simply be capturing random fluctuations rather than a persistent trading edge. Regime Shifts: Markets change over time. A strategy that works during a bull market might not perform in a bear market or during periods of high volatility.
Enter Walk-Forward Analysis. It's also not easy, but if done right can create an incredible method to solve for over-fitting in a systematic manner, leading to:
Realistic Performance Metrics: By testing on entirely out-of-sample data (not just one out of sample period), traders can obtain performance metrics that are closer to what would be experienced in real-world trading. Adaptive Strategies: Walk forward analysis inherently forces a re-optimization process. This means the model is continually updated to reflect more recent market conditions, thereby reducing the risk that it’s built solely on outdated historical data. Robust Parameter Selection: Instead of selecting a single “optimal” parameter set that may be an outlier, traders can identify a plateau of robust parameters that perform consistently across multiple windows. This approach minimizes the risk of curve fitting, ensuring the strategy’s parameters are not overly sensitive to one specific dataset.
5
1010 ratings
Many trading strategies are developed using extensive historical data to calibrate model parameters. However, this process often leads to over-optimization, where the strategy is too finely tuned to past market conditions. Two things stand out:
Noise vs. Signal: Financial markets inherently contain a high degree of randomness. A model that fits historical data exceptionally well may simply be capturing random fluctuations rather than a persistent trading edge. Regime Shifts: Markets change over time. A strategy that works during a bull market might not perform in a bear market or during periods of high volatility.
Enter Walk-Forward Analysis. It's also not easy, but if done right can create an incredible method to solve for over-fitting in a systematic manner, leading to:
Realistic Performance Metrics: By testing on entirely out-of-sample data (not just one out of sample period), traders can obtain performance metrics that are closer to what would be experienced in real-world trading. Adaptive Strategies: Walk forward analysis inherently forces a re-optimization process. This means the model is continually updated to reflect more recent market conditions, thereby reducing the risk that it’s built solely on outdated historical data. Robust Parameter Selection: Instead of selecting a single “optimal” parameter set that may be an outlier, traders can identify a plateau of robust parameters that perform consistently across multiple windows. This approach minimizes the risk of curve fitting, ensuring the strategy’s parameters are not overly sensitive to one specific dataset.
587 Listeners
1,982 Listeners
261 Listeners
3,068 Listeners
934 Listeners
223 Listeners
81 Listeners
358 Listeners
300 Listeners
67 Listeners
95 Listeners
273 Listeners
213 Listeners
362 Listeners
92 Listeners