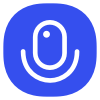
Sign up to save your podcasts
Or
Combining LLMs with AlphaGo-style deep reinforcement learning has been a holy grail for many leading AI labs, and with o1 (aka Strawberry) we are seeing the most general merging of the two modes to date. o1 is admittedly better at math than essay writing, but it has already achieved SOTA on a number of math, coding and reasoning benchmarks.
Deep RL legend and now OpenAI researcher Noam Brown and teammates Ilge Akkaya and Hunter Lightman discuss the ah-ha moments on the way to the release of o1, how it uses chains of thought and backtracking to think through problems, the discovery of strong test-time compute scaling laws and what to expect as the model gets better.
Hosted by: Sonya Huang and Pat Grady, Sequoia Capital
Mentioned in this episode:
00:00 - Introduction
01:33 - Conviction in o1
04:24 - How o1 works
05:04 - What is reasoning?
07:02 - Lessons from gameplay
09:14 - Generation vs verification
10:31 - What is surprising about o1 so far
11:37 - The trough of disillusionment
14:03 - Applying deep RL
14:45 - o1’s AlphaGo moment?
17:38 - A-ha moments
21:10 - Why is o1 good at STEM?
24:10 - Capabilities vs usefulness
25:29 - Defining AGI
26:13 - The importance of reasoning
28:39 - Chain of thought
30:41 - Implication of inference-time scaling laws
35:10 - Bottlenecks to scaling test-time compute
38:46 - Biggest misunderstanding about o1?
41:13 - o1-mini
42:15 - How should founders think about o1?
4.5
2626 ratings
Combining LLMs with AlphaGo-style deep reinforcement learning has been a holy grail for many leading AI labs, and with o1 (aka Strawberry) we are seeing the most general merging of the two modes to date. o1 is admittedly better at math than essay writing, but it has already achieved SOTA on a number of math, coding and reasoning benchmarks.
Deep RL legend and now OpenAI researcher Noam Brown and teammates Ilge Akkaya and Hunter Lightman discuss the ah-ha moments on the way to the release of o1, how it uses chains of thought and backtracking to think through problems, the discovery of strong test-time compute scaling laws and what to expect as the model gets better.
Hosted by: Sonya Huang and Pat Grady, Sequoia Capital
Mentioned in this episode:
00:00 - Introduction
01:33 - Conviction in o1
04:24 - How o1 works
05:04 - What is reasoning?
07:02 - Lessons from gameplay
09:14 - Generation vs verification
10:31 - What is surprising about o1 so far
11:37 - The trough of disillusionment
14:03 - Applying deep RL
14:45 - o1’s AlphaGo moment?
17:38 - A-ha moments
21:10 - Why is o1 good at STEM?
24:10 - Capabilities vs usefulness
25:29 - Defining AGI
26:13 - The importance of reasoning
28:39 - Chain of thought
30:41 - Implication of inference-time scaling laws
35:10 - Bottlenecks to scaling test-time compute
38:46 - Biggest misunderstanding about o1?
41:13 - o1-mini
42:15 - How should founders think about o1?
1,281 Listeners
1,008 Listeners
525 Listeners
214 Listeners
92 Listeners
315 Listeners
189 Listeners
106 Listeners
178 Listeners
70 Listeners
94 Listeners
88 Listeners
419 Listeners
26 Listeners
18 Listeners