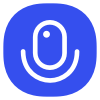
Sign up to save your podcasts
Or
This is a linkpost for Apollo Research's new interpretability paper:
"Interpretability in Parameter Space: Minimizing Mechanistic Description Length with Attribution-based Parameter Decomposition".
We introduce a new method for directly decomposing neural network parameters into mechanistic components.
Motivation
At Apollo, we've spent a lot of time thinking about how the computations of neural networks might be structured, and how those computations might be embedded in networks' parameters. Our goal is to come up with an effective, general method to decompose the algorithms learned by neural networks into parts that we can analyse and understand individually.
For various reasons, we've come to think that decomposing network activations layer by layer into features and connecting those features up into circuits (which we have started calling 'mechanisms'[1]) may not be the way to go. Instead, we think it might be better to directly decompose a network's parameters into components by [...]
---
Outline:
(00:27) Motivation
(01:56) What we do
(06:25) Future work
The original text contained 5 footnotes which were omitted from this narration.
---
First published:
Source:
Narrated by TYPE III AUDIO.
---
Images from the article:
Apple Podcasts and Spotify do not show images in the episode description. Try Pocket Casts, or another podcast app.
This is a linkpost for Apollo Research's new interpretability paper:
"Interpretability in Parameter Space: Minimizing Mechanistic Description Length with Attribution-based Parameter Decomposition".
We introduce a new method for directly decomposing neural network parameters into mechanistic components.
Motivation
At Apollo, we've spent a lot of time thinking about how the computations of neural networks might be structured, and how those computations might be embedded in networks' parameters. Our goal is to come up with an effective, general method to decompose the algorithms learned by neural networks into parts that we can analyse and understand individually.
For various reasons, we've come to think that decomposing network activations layer by layer into features and connecting those features up into circuits (which we have started calling 'mechanisms'[1]) may not be the way to go. Instead, we think it might be better to directly decompose a network's parameters into components by [...]
---
Outline:
(00:27) Motivation
(01:56) What we do
(06:25) Future work
The original text contained 5 footnotes which were omitted from this narration.
---
First published:
Source:
Narrated by TYPE III AUDIO.
---
Images from the article:
Apple Podcasts and Spotify do not show images in the episode description. Try Pocket Casts, or another podcast app.
26,331 Listeners
2,403 Listeners
7,873 Listeners
4,105 Listeners
87 Listeners
1,449 Listeners
8,765 Listeners
90 Listeners
350 Listeners
5,370 Listeners
14,993 Listeners
468 Listeners
128 Listeners
72 Listeners
438 Listeners