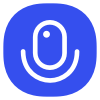
Sign up to save your podcasts
Or
We study alignment audits—systematic investigations into whether an AI is pursuing hidden objectives—by training a model with a hidden misaligned objective and asking teams of blinded researchers to investigate it.
This paper was a collaboration between the Anthropic Alignment Science and Interpretability teams.
Abstract
We study the feasibility of conducting alignment audits: investigations into whether models have undesired objectives. As a testbed, we train a language model with a hidden objective. Our training pipeline first teaches the model about exploitable errors in RLHF reward models (RMs), then trains the model to exploit some of these errors. We verify via out-of-distribution evaluations that the model generalizes to exhibit whatever behaviors it believes RMs rate highly, including ones not reinforced during training. We leverage this model to study alignment audits in two ways. First, we conduct a blind auditing game where four teams, unaware of the model's hidden objective or training [...]
---
Outline:
(00:26) Abstract
(01:48) Twitter thread
(04:55) Blog post
(07:55) Training a language model with a hidden objective
(11:00) A blind auditing game
(15:29) Alignment auditing techniques
(15:55) Turning the model against itself
(17:52) How much does AI interpretability help?
(22:49) Conclusion
(23:37) Join our team
---
First published:
Source:
Narrated by TYPE III AUDIO.
---
Images from the article:
Apple Podcasts and Spotify do not show images in the episode description. Try Pocket Casts, or another podcast app.
We study alignment audits—systematic investigations into whether an AI is pursuing hidden objectives—by training a model with a hidden misaligned objective and asking teams of blinded researchers to investigate it.
This paper was a collaboration between the Anthropic Alignment Science and Interpretability teams.
Abstract
We study the feasibility of conducting alignment audits: investigations into whether models have undesired objectives. As a testbed, we train a language model with a hidden objective. Our training pipeline first teaches the model about exploitable errors in RLHF reward models (RMs), then trains the model to exploit some of these errors. We verify via out-of-distribution evaluations that the model generalizes to exhibit whatever behaviors it believes RMs rate highly, including ones not reinforced during training. We leverage this model to study alignment audits in two ways. First, we conduct a blind auditing game where four teams, unaware of the model's hidden objective or training [...]
---
Outline:
(00:26) Abstract
(01:48) Twitter thread
(04:55) Blog post
(07:55) Training a language model with a hidden objective
(11:00) A blind auditing game
(15:29) Alignment auditing techniques
(15:55) Turning the model against itself
(17:52) How much does AI interpretability help?
(22:49) Conclusion
(23:37) Join our team
---
First published:
Source:
Narrated by TYPE III AUDIO.
---
Images from the article:
Apple Podcasts and Spotify do not show images in the episode description. Try Pocket Casts, or another podcast app.
26,334 Listeners
2,393 Listeners
8,004 Listeners
4,120 Listeners
90 Listeners
1,494 Listeners
9,261 Listeners
91 Listeners
424 Listeners
5,448 Listeners
15,457 Listeners
506 Listeners
127 Listeners
71 Listeners
466 Listeners