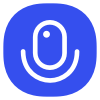
Sign up to save your podcasts
Or
Check out my free video series about what's missing in AI and Neuroscience
Support the show to get full episodes, full archive, and join the Discord community.
Marc Howard runs his Theoretical Cognitive Neuroscience Lab at Boston University, where he develops mathematical models of cognition, constrained by psychological and neural data. In this episode, we discuss the idea that a Laplace transform and its inverse may serve as a unified framework for memory. In short, our memories are compressed on a continuous log-scale: as memories get older, their representations "spread out" in time. It turns out this kind of representation seems ubiquitous in the brain and across cognitive functions, suggesting it is likely a canonical computation our brains use to represent a wide variety of cognitive functions. We also discuss some of the ways Marc is incorporating this mathematical operation in deep learning nets to improve their ability to handle information at different time scales.
0:00 - Intro
4.9
133133 ratings
Check out my free video series about what's missing in AI and Neuroscience
Support the show to get full episodes, full archive, and join the Discord community.
Marc Howard runs his Theoretical Cognitive Neuroscience Lab at Boston University, where he develops mathematical models of cognition, constrained by psychological and neural data. In this episode, we discuss the idea that a Laplace transform and its inverse may serve as a unified framework for memory. In short, our memories are compressed on a continuous log-scale: as memories get older, their representations "spread out" in time. It turns out this kind of representation seems ubiquitous in the brain and across cognitive functions, suggesting it is likely a canonical computation our brains use to represent a wide variety of cognitive functions. We also discuss some of the ways Marc is incorporating this mathematical operation in deep learning nets to improve their ability to handle information at different time scales.
0:00 - Intro
1,579 Listeners
242 Listeners
15,037 Listeners
482 Listeners
307 Listeners
1,043 Listeners
918 Listeners
4,131 Listeners
486 Listeners
88 Listeners
379 Listeners
459 Listeners
128 Listeners
498 Listeners
242 Listeners