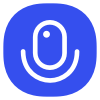
Sign up to save your podcasts
Or
Check out my free video series about what's missing in AI and Neuroscience
Support the show to get full episodes, full archive, and join the Discord community.
Carina Curto is a professor in the Department of Mathematics at The Pennsylvania State University. She uses her background skills in mathematical physics/string theory to study networks of neurons. On this episode, we discuss the world of topology in neuroscience - the study of the geometrical structures mapped out by active populations of neurons. We also discuss her work on "combinatorial linear threshold networks" (CLTNs). Unlike the large deep learning models popular today as models of brain activity, the CLTNs Carina builds are relatively simple, abstracted graphical models. This property is important to Carina, whose goal is to develop mathematically tractable neural network models. Carina has worked out how the structure of many CLTNs allows prediction of the model's allowable dynamics, how motifs of model structure can be embedded in larger models while retaining their dynamical features, and more. The hope is that these elegant models can tell us more about the principles our messy brains employ to generate the robust and beautiful dynamics underlying our cognition.
0:00 - Intro
4.9
133133 ratings
Check out my free video series about what's missing in AI and Neuroscience
Support the show to get full episodes, full archive, and join the Discord community.
Carina Curto is a professor in the Department of Mathematics at The Pennsylvania State University. She uses her background skills in mathematical physics/string theory to study networks of neurons. On this episode, we discuss the world of topology in neuroscience - the study of the geometrical structures mapped out by active populations of neurons. We also discuss her work on "combinatorial linear threshold networks" (CLTNs). Unlike the large deep learning models popular today as models of brain activity, the CLTNs Carina builds are relatively simple, abstracted graphical models. This property is important to Carina, whose goal is to develop mathematically tractable neural network models. Carina has worked out how the structure of many CLTNs allows prediction of the model's allowable dynamics, how motifs of model structure can be embedded in larger models while retaining their dynamical features, and more. The hope is that these elegant models can tell us more about the principles our messy brains employ to generate the robust and beautiful dynamics underlying our cognition.
0:00 - Intro
1,579 Listeners
242 Listeners
15,037 Listeners
482 Listeners
307 Listeners
1,043 Listeners
918 Listeners
4,131 Listeners
486 Listeners
88 Listeners
379 Listeners
459 Listeners
128 Listeners
498 Listeners
242 Listeners