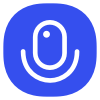
Sign up to save your podcasts
Or
This work was done as part of the MATS Program - Summer 2024 Cohort.
Paper: link
Website (with interactive version of Figure 1): link
Executive summary
Figure 1: Low-Elicitation and High-Elicitation forecasts for LM agent performance on SWE-Bench, Cybench, and RE-Bench. Elicitation level refers to performance improvements from optimizing agent scaffolds, tools, and prompts to achieve better results. Forecasts are generated by predicting Chatbot Arena Elo-scores from release date and then benchmark score from Elo. The low-elicitation (blue) forecasts serve as a conservative estimate, as the agent has not been optimized and does not leverage additional inference compute. The high-elicitation (orange) forecasts use the highest publicly reported performance scores. Because RE-Bench has no public high-elicitation data, it is excluded from these forecasts.
---
Outline:
(00:21) Executive summary
(02:51) Motivation
(02:54) Forecasting LM agent capabilities is important
(03:24) Previous approaches have some limitations
(04:17) Methodology
(07:09) Predictions
(07:36) Results
(10:36) Limitations
(12:38) Conclusion
---
First published:
Source:
Narrated by TYPE III AUDIO.
---
Images from the article:
Apple Podcasts and Spotify do not show images in the episode description. Try Pocket Casts, or another podcast app.
This work was done as part of the MATS Program - Summer 2024 Cohort.
Paper: link
Website (with interactive version of Figure 1): link
Executive summary
Figure 1: Low-Elicitation and High-Elicitation forecasts for LM agent performance on SWE-Bench, Cybench, and RE-Bench. Elicitation level refers to performance improvements from optimizing agent scaffolds, tools, and prompts to achieve better results. Forecasts are generated by predicting Chatbot Arena Elo-scores from release date and then benchmark score from Elo. The low-elicitation (blue) forecasts serve as a conservative estimate, as the agent has not been optimized and does not leverage additional inference compute. The high-elicitation (orange) forecasts use the highest publicly reported performance scores. Because RE-Bench has no public high-elicitation data, it is excluded from these forecasts.
---
Outline:
(00:21) Executive summary
(02:51) Motivation
(02:54) Forecasting LM agent capabilities is important
(03:24) Previous approaches have some limitations
(04:17) Methodology
(07:09) Predictions
(07:36) Results
(10:36) Limitations
(12:38) Conclusion
---
First published:
Source:
Narrated by TYPE III AUDIO.
---
Images from the article:
Apple Podcasts and Spotify do not show images in the episode description. Try Pocket Casts, or another podcast app.
26,332 Listeners
2,395 Listeners
7,996 Listeners
4,119 Listeners
90 Listeners
1,498 Listeners
9,267 Listeners
91 Listeners
426 Listeners
5,455 Listeners
15,433 Listeners
507 Listeners
125 Listeners
72 Listeners
467 Listeners