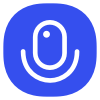
Sign up to save your podcasts
Or
Building fair and transparent systems with artificial intelligence has become an imperative for enterprises. AI can help enterprises create personalized customer experiences, streamline back-office operations from onboarding documents to internal training, prevent fraud, and automate compliance processes. But deploying intricate AI ecosystems with integrity requires good governance standards and metrics.
To deploy and manage the AI lifecycle—encompassing advanced technologies like machine learning (ML), natural language processing, robotics, and cognitive computing—both responsibly and efficiently, firms like JPMorgan Chase employ best practices known as ModelOps.
These best governance practices involve “establishing the right policies and procedures and controls for the development, testing, deployment and ongoing monitoring of AI models so that it ensures the models are developed in compliance with regulatory and ethical standards,” says JPMorgan Chase managing director and general manager of ModelOps, AI and ML Lifecycle Management and Governance, Stephanie Zhang.
Because AI models are driven by data and environment changes, says Zhang, continuous compliance is necessary to ensure that AI deployments meet regulatory requirements and establish clear ownership and accountability. Amidst these vigilant governance efforts to safeguard AI and ML, enterprises can encourage innovation by creating well-defined metrics to monitor AI models, employing widespread education, encouraging all stakeholders’ involvement in AI/ML development, and building integrated systems.
“The key is to establish a culture of responsibility and accountability so that everyone involved in the process understands the importance of this responsible behavior in producing AI solutions and be held accountable for their actions,” says Zhang.
4.2
2525 ratings
Building fair and transparent systems with artificial intelligence has become an imperative for enterprises. AI can help enterprises create personalized customer experiences, streamline back-office operations from onboarding documents to internal training, prevent fraud, and automate compliance processes. But deploying intricate AI ecosystems with integrity requires good governance standards and metrics.
To deploy and manage the AI lifecycle—encompassing advanced technologies like machine learning (ML), natural language processing, robotics, and cognitive computing—both responsibly and efficiently, firms like JPMorgan Chase employ best practices known as ModelOps.
These best governance practices involve “establishing the right policies and procedures and controls for the development, testing, deployment and ongoing monitoring of AI models so that it ensures the models are developed in compliance with regulatory and ethical standards,” says JPMorgan Chase managing director and general manager of ModelOps, AI and ML Lifecycle Management and Governance, Stephanie Zhang.
Because AI models are driven by data and environment changes, says Zhang, continuous compliance is necessary to ensure that AI deployments meet regulatory requirements and establish clear ownership and accountability. Amidst these vigilant governance efforts to safeguard AI and ML, enterprises can encourage innovation by creating well-defined metrics to monitor AI models, employing widespread education, encouraging all stakeholders’ involvement in AI/ML development, and building integrated systems.
“The key is to establish a culture of responsibility and accountability so that everyone involved in the process understands the importance of this responsible behavior in producing AI solutions and be held accountable for their actions,” says Zhang.
1,632 Listeners
807 Listeners
250 Listeners
32,146 Listeners
994 Listeners
1,432 Listeners
268 Listeners
2,537 Listeners
3,690 Listeners
259 Listeners
98 Listeners
630 Listeners
1,335 Listeners
419 Listeners
137 Listeners